Hadoop_MapReduce_Shuffle工作原理
Shuffle 是连接 Mapper 和 Reducer 之间的桥梁,Mapper的输出结果必须经过Shuffle环节才能传递给Reducer
Shuffle分为Map阶段的数据准备和Reducer阶段的数据拷贝。
Shuffle核心机制:数据分区、排序、合并。
在 mapTask 阶段,通过代码:context.write(key, iw) 将 mapTask 输出的Key和Value写进buffer(缓冲区),缓冲区默认大小为100M,如果mapTask的输出很大(缓冲区大小不足),(默认当缓存超出缓冲区大小的80%时)会发生多次溢写操作(将数据从缓冲区写入磁盘),生成多个小文件,然后合并为一个大文件。
将数据从缓冲区写入磁盘之前,会先进行分区(partition),每个分区会默认按键(字典顺序)进行排序。
reduceTask 在执行前,不断拉取每个mapTask的最终结果,然后将从不同mapTask拉取来的数据做合并(merge),最终形成一个文件作为 reduceTask 的输入文件。
自定义分区
Partitioner类用于指定map的输出值对应由哪个reducer处理,而Hadoop默认的reducer只有一个,此时的Partitioner类没有意义,但当修改reducer的个数为多个的时候,Partitioner类就会指定key所对应的reducer节点序号(从0开始)。
默认地,Hadoop对KV对中的Key取hash值来确定分区。在这种情况下,当前数据要进入哪个分区,用户是不可控的。可以通过job.setPartitionerClass方法指定自定义的Partitioner类。
示例:将WordCount的统计结果按不同首字母输出到不同文件。
步骤:
- 自定义类继承Partitioner并重写getPartition()方法
- 在 job 中指定自定义的Partitioner类
- 根据自定义的Partitioner类的逻辑设置相应数量的reduce Task
注意:
- 如果reduceTask数量大于分区数量,会多产生几个空的输出文件part-r-000xx;
- 如果reduceTask数量小于分区数量且大于1,会报错(一部分数据得不到处理);
- 如果reduceTask数量为1(默认值),无论mapTask生成多少个分区文件,都会给这一个reduceTask处理,最终只产生一个输出文件。
输入数据(D:\data\poem.txt):
Two roads diverged in a yellow wood,
And sorry I could not travel both
And be one traveler, long I stood
And looked down one as far as I could
To where it bent in the undergrowth;
Then took the other, as just as fair,
And having perhaps the better claim,
Because it was grassy and wanted wear;
Though as for that the passing there
Had worn them really about the same,
And both that morning equally lay
In leaves no step had trodden black.
Oh, I kept the first for another day!
Yet knowing how way leads on to way,
I doubted if I should ever come back.
I shall be telling this with a sigh
Somewhere ages and ages hence:
Two roads diverged in a wood,
and I took the one less traveled by,
And that has made all the difference.
- 创建 MyPartitioner 类:
getPartition方法的返回值有0,1,2三种,表示三个分区。首字母为a-g的单词为0号分区,首字母为h-n的单词为1号分区,其他单词为2号分区,忽略大小写。
package com.blu.mywordcount.partition;
import org.apache.hadoop.io.IntWritable;
import org.apache.hadoop.io.Text;
import org.apache.hadoop.mapreduce.Partitioner;
/**
* 泛型是mapper输出的Key和Value
* @author BLU
*/
public class MyPartitioner extends Partitioner<Text, IntWritable>{
/**
* 定义分区
* 以a-g开头的单词放在一个文件中
* 以h-n开头的单词放在一个文件中
*/
@Override
public int getPartition(Text key, IntWritable value, int numPartitions) {
String word = key.toString();
int num = 2;
if(word.matches("(?i)^[a-g]{1}\\S*$")) {
num = 0;
}else if(word.matches("(?i)^[h-n]{1}\\S*$")){
num = 1;
}
return num;
}
}
- 修改 MyWordCount 类(因为分区数量为3,所以也要设置reduceTask的数量为3):
package com.blu.mywordcount;
import org.apache.hadoop.conf.Configuration;
import org.apache.hadoop.fs.Path;
import org.apache.hadoop.io.IntWritable;
import org.apache.hadoop.io.Text;
import org.apache.hadoop.mapreduce.Job;
import org.apache.hadoop.mapreduce.lib.input.FileInputFormat;
import org.apache.hadoop.mapreduce.lib.output.FileOutputFormat;
import com.blu.mywordcount.partition.MyPartitioner;
public class MyWordCount {
public static void main(String[] args) {
try {
Configuration conf = new Configuration();
Job job = Job.getInstance(conf);
job.setJarByClass(MyWordCount.class);
job.setMapperClass(MyWordCountMapper.class);
job.setReducerClass(MyWordCountReducer.class);
job.setMapOutputKeyClass(Text.class);
job.setMapOutputValueClass(IntWritable.class);
job.setOutputKeyClass(Text.class);
job.setOutputValueClass(IntWritable.class);
//设置自定义的分区
job.setPartitionerClass(MyPartitioner.class);
//设置reduceTask的数量
job.setNumReduceTasks(3);
FileInputFormat.addInputPath(job, new Path(args[0]));
FileOutputFormat.setOutputPath(job, new Path(args[1]));
boolean flag = job.waitForCompletion(true);
System.exit(flag ?0 : 1);
} catch (Exception e) {
e.printStackTrace();
}
}
}
关键代码:
//设置自定义的分区
job.setPartitionerClass(MyPartitioner.class);
//设置reduceTask的数量
job.setNumReduceTasks(3);
- 运行参数:
D:\data\poem.txt D:\data\output
- 运行结果:
part-r-00000
And 6
Because 1
a 3
about 1
ages 2
all 1
and 3
another 1
as 5
back. 1
be 2
bent 1
better 1
black. 1
both 2
by, 1
claim, 1
come 1
could 2
day! 1
difference. 1
diverged 2
doubted 1
down 1
equally 1
ever 1
fair, 1
far 1
first 1
for 2
grassy 1
part-r-00001
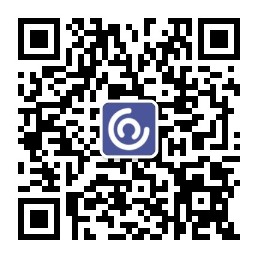
Had 1
I 8
In 1
had 1
has 1
having 1
hence: 1
how 1
if 1
in 3
it 2
just 1
kept 1
knowing 1
lay 1
leads 1
leaves 1
less 1
long 1
looked 1
made 1
morning 1
no 1
not 1
part-r-00002
Oh, 1
Somewhere 1
Then 1
Though 1
To 1
Two 2
Yet 1
on 1
one 3
other, 1
passing 1
perhaps 1
really 1
roads 2
same, 1
shall 1
should 1
sigh 1
sorry 1
step 1
stood 1
telling 1
that 3
the 8
them 1
there 1
this 1
to 1
took 2
travel 1
traveled 1
traveler, 1
trodden 1
undergrowth; 1
wanted 1
was 1
way 1
way, 1
wear; 1
where 1
with 1
wood, 2
worn 1
yellow 1
自定义排序 — 默认1个分区
示例:对以下文件的数据按照结余进行倒序或正序排序:
D:\data\sort.txt
lisi 160 200 -40.0
blu 4000 400 3600.0
zhangsan 100 50 50.0
- User类
package com.blu.sort;
import java.io.DataInput;
import java.io.DataOutput;
import java.io.IOException;
import org.apache.hadoop.io.WritableComparable;
public class User implements WritableComparable<User>{
/**
* lisi 160 200 -40.0
*/
private String uname;
private Double income;
private Double expend;
private Double shengyu;
public void readFields(DataInput in) throws IOException {
uname = in.readUTF();
income = in.readDouble();
expend = in.readDouble();
shengyu = in.readDouble();
}
public void write(DataOutput out) throws IOException {
out.writeUTF(uname);
out.writeDouble(income);
out.writeDouble(expend);
out.writeDouble(shengyu);
}
/**
* 比较
* 返回1:a>b
* 返回0:a=b
* 返回-1:a<b
*/
public int compareTo(User o) {
if(shengyu<o.getShengyu()) {
return 1;
}else if(shengyu>o.getShengyu()) {
return -1;
}else {
return 0;
}
}
public String getUname() {
return uname;
}
public void setUname(String uname) {
this.uname = uname;
}
public Double getIncome() {
return income;
}
public void setIncome(Double income) {
this.income = income;
}
public Double getExpend() {
return expend;
}
public void setExpend(Double expend) {
this.expend = expend;
}
public Double getShengyu() {
return shengyu;
}
public void setShengyu(Double shengyu) {
this.shengyu = shengyu;
}
@Override
public String toString() {
return uname + " " + income + " " + expend + " " + shengyu;
}
}
- MySortMapper
package com.blu.sort;
import java.io.IOException;
import org.apache.hadoop.io.LongWritable;
import org.apache.hadoop.io.Text;
import org.apache.hadoop.mapreduce.Mapper;
public class MySortMapper extends Mapper<LongWritable, Text, User, Text>{
User u = new User();
Text t = new Text();
@Override
protected void map(LongWritable key, Text value, Mapper<LongWritable, Text, User, Text>.Context context)
throws IOException, InterruptedException {
String result = value.toString();
String[] vals = result.split(" ");
u.setUname(vals[0]);
u.setIncome(Double.parseDouble(vals[1]));
u.setExpend(Double.parseDouble(vals[2]));
u.setShengyu(Double.parseDouble(vals[3]));
context.write(u, t);
}
}
- MySortReducer
package com.blu.sort;
import java.io.IOException;
import org.apache.hadoop.io.NullWritable;
import org.apache.hadoop.io.Text;
import org.apache.hadoop.mapreduce.Reducer;
public class MySortReducer extends Reducer<User, Text, User, NullWritable>{
@Override
protected void reduce(User key, Iterable<Text> value, Reducer<User, Text, User, NullWritable>.Context context)
throws IOException, InterruptedException {
context.write(key, NullWritable.get());
}
}
- MySortJob
package com.blu.sort;
import org.apache.hadoop.fs.Path;
import org.apache.hadoop.io.NullWritable;
import org.apache.hadoop.io.Text;
import org.apache.hadoop.mapreduce.Job;
import org.apache.hadoop.mapreduce.lib.input.FileInputFormat;
import org.apache.hadoop.mapreduce.lib.output.FileOutputFormat;
public class MySortJob {
public static void main(String[] args) throws Exception {
Job job = Job.getInstance();
job.setJarByClass(MySortJob.class);
job.setMapperClass(MySortMapper.class);
job.setReducerClass(MySortReducer.class);
job.setMapOutputKeyClass(User.class);
job.setMapOutputValueClass(Text.class);
job.setOutputKeyClass(User.class);
job.setOutputValueClass(NullWritable.class);
FileInputFormat.addInputPath(job, new Path("D:\\data\\sort.txt"));
FileOutputFormat.setOutputPath(job, new Path("D:\\data\\output"));
boolean flag = job.waitForCompletion(true);
System.exit(flag ?0 : 1);
}
}
- 运行结果:
blu 4000.0 400.0 3600.0
zhangsan 100.0 50.0 50.0
lisi 160.0 200.0 -40.0
自定义排序 — 多个分区
示例:将D:\data\sort.txt文件按结余排序且a-g开头的姓名输出到一个文件,h-n开头的输出到第二个文件,其余的输出到第三个文件
sort.txt:
lisi 160 200 -40.0
blu 4000 400 3600.0
zhangsan 100 50 50.0
xiaoming 160 100 60
kat 0 800 -800
amy 1000 1 999
wangwu 800 400 400
candy 1 1 0
elly 250 20 230
greepy 1400 1300 100
jakaboy 1000 0 1000
micppi 488 108 380
picimy 4000 4001 -1
ruby 300 30 270
sunbun 750 1000 -250
yangyin 4000 1000 3000
修改上面的 “自定义排序 — 默认1个分区” 中的代码:
- 增加自定义分区
创建MySortPartitioner类:
package com.blu.sort;
import org.apache.hadoop.io.Text;
import org.apache.hadoop.mapreduce.Partitioner;
public class MySortPartitioner extends Partitioner<User, Text>{
@Override
public int getPartition(User key, Text value, int numPartitions) {
int num = 2;
String uname = key.getUname();
if(uname.matches("(?i)^[a-g]{1}\\S*$")) {
return 0;
}else if(uname.matches("(?i)^[h-n]{1}\\S*$")){
num = 1;
}else {
return num;
}
return num;
}
}
- 修改MySortJob
package com.blu.sort;
import org.apache.hadoop.fs.Path;
import org.apache.hadoop.io.NullWritable;
import org.apache.hadoop.io.Text;
import org.apache.hadoop.mapreduce.Job;
import org.apache.hadoop.mapreduce.lib.input.FileInputFormat;
import org.apache.hadoop.mapreduce.lib.output.FileOutputFormat;
public class MySortJob {
public static void main(String[] args) throws Exception {
Job job = Job.getInstance();
job.setJarByClass(MySortJob.class);
job.setMapperClass(MySortMapper.class);
job.setReducerClass(MySortReducer.class);
job.setMapOutputKeyClass(User.class);
job.setMapOutputValueClass(Text.class);
job.setOutputKeyClass(User.class);
job.setOutputValueClass(NullWritable.class);
//设置Partitioner类
job.setPartitionerClass(MySortPartitioner.class);
//设置reduce task的数量
job.setNumReduceTasks(3);
FileInputFormat.addInputPath(job, new Path("D:\\data\\sort.txt"));
FileOutputFormat.setOutputPath(job, new Path("D:\\data\\output"));
boolean flag = job.waitForCompletion(true);
System.exit(flag ?0 : 1);
}
}
主要修改代码:
//设置Partitioner类
job.setPartitionerClass(MySortPartitioner.class);
//设置reduce task的数量
job.setNumReduceTasks(3);
- 运行结果
part-r-00000
blu 4000.0 400.0 3600.0
amy 1000.0 1.0 999.0
elly 250.0 20.0 230.0
greepy 1400.0 1300.0 100.0
candy 1.0 1.0 0.0
part-r-00001
jakaboy 1000.0 0.0 1000.0
micppi 488.0 108.0 380.0
lisi 160.0 200.0 -40.0
kat 0.0 800.0 -800.0
part-r-00002
yangyin 4000.0 1000.0 3000.0
wangwu 800.0 400.0 400.0
ruby 300.0 30.0 270.0
xiaoming 160.0 100.0 60.0
zhangsan 100.0 50.0 50.0
picimy 4000.0 4001.0 -1.0
sunbun 750.0 1000.0 -250.0
Combiner合并
Combiner可以减少 Map 阶段的输出结果数,降低网络开销。默认是没有Combiner的,需要用户自定义Combiner类,Combiner类必须是Reducer的子类,且Combiner的输入类型和输出类型都必须是mapper的输出类型。
示例:修改 Hadoop_WordCount单词统计 工程
- 输入数据(D:\data\poem.txt):
Two roads diverged in a yellow wood,
And sorry I could not travel both
And be one traveler, long I stood
And looked down one as far as I could
To where it bent in the undergrowth;
Then took the other, as just as fair,
And having perhaps the better claim,
Because it was grassy and wanted wear;
Though as for that the passing there
Had worn them really about the same,
And both that morning equally lay
In leaves no step had trodden black.
Oh, I kept the first for another day!
Yet knowing how way leads on to way,
I doubted if I should ever come back.
I shall be telling this with a sigh
Somewhere ages and ages hence:
Two roads diverged in a wood,
and I took the one less traveled by,
And that has made all the difference.
- 先运行MyWordCount类
package com.blu.mywordcount;
import org.apache.hadoop.conf.Configuration;
import org.apache.hadoop.fs.Path;
import org.apache.hadoop.io.IntWritable;
import org.apache.hadoop.io.Text;
import org.apache.hadoop.mapreduce.Job;
import org.apache.hadoop.mapreduce.lib.input.FileInputFormat;
import org.apache.hadoop.mapreduce.lib.output.FileOutputFormat;
import com.blu.mywordcount.combiner.MyCombiner;
import com.blu.mywordcount.partition.MyPartitioner;
public class MyWordCount {
public static void main(String[] args) {
try {
Configuration conf = new Configuration();
Job job = Job.getInstance(conf);
job.setJarByClass(MyWordCount.class);
job.setMapperClass(MyWordCountMapper.class);
job.setReducerClass(MyWordCountReducer.class);
job.setMapOutputKeyClass(Text.class);
job.setMapOutputValueClass(IntWritable.class);
job.setOutputKeyClass(Text.class);
job.setOutputValueClass(IntWritable.class);
FileInputFormat.addInputPath(job, new Path(args[0]));
FileOutputFormat.setOutputPath(job, new Path(args[1]));
boolean flag = job.waitForCompletion(true);
System.exit(flag ?0 : 1);
} catch (Exception e) {
e.printStackTrace();
}
}
}
- 控制台打印( 默认没有Combine的情况:Combine 的输入和输出都为0 且 Map output records 等于 Reduce input records):
Map input records=20
Map output records=143
Map output bytes=1296
Map output materialized bytes=1588
Input split bytes=87
Combine input records=0
Combine output records=0
Reduce input groups=99
Reduce shuffle bytes=1588
Reduce input records=143
Reduce output records=99
- 创建 MyCombiner 类
package com.blu.mywordcount.combiner;
import java.io.IOException;
import org.apache.hadoop.io.IntWritable;
import org.apache.hadoop.io.Text;
import org.apache.hadoop.mapreduce.Reducer;
/**
* Combiner的输入类型和输出类型都是mapper的输出类型
* @author BLU
*/
public class MyCombiner extends Reducer<Text, IntWritable, Text, IntWritable>{
IntWritable iw = new IntWritable();
@Override
protected void reduce(Text key, Iterable<IntWritable> value,
Reducer<Text, IntWritable, Text, IntWritable>.Context context) throws IOException, InterruptedException {
int sum = 0;
for(IntWritable i : value) {
sum += i.get();
}
iw.set(sum);
context.write(key, iw);
}
}
- 在 MyWordCount 中添加 MyCombiner 类:
//设置Combiner的类
job.setCombinerClass(MyCombiner.class);
- 重新运行,控制台打印(此时 Combine 的输入输出不为0,且 Reduce input records 不等于 Map output records,):
Map input records=20
Map output records=143
Map output bytes=1296
Map output materialized bytes=1158
Input split bytes=87
Combine input records=143
Combine output records=99
Reduce input groups=99
Reduce shuffle bytes=1158
Reduce input records=99
Reduce output records=99