背景
了解dagSchedular提交job,就需要了解什么是job,什么是stage,如果我们写了一段程序,其中调用了多个spark算子,但是我们知道,实际在计算的时候,只有在遇到action算子的时候,才会触发计算操作,而这个计算操作就是一个job,所以说一次action操作就会触发提交一个job,比如collect和first操作都会触发sparkcontext的runjob提交job的操作,代码如下:
def collect(): Array[T] = withScope { //调用的sparkcontext的runjob方法 val results = sc.runJob(this, (iter: Iterator[T]) => iter.toArray) Array.concat(results: _*) }
stage具体划分方式取决于依赖的类型,宽依赖还是窄依赖,一个job触发往往会包含一个或者多个stage,这些stage都会包含一个driver生成的一个jobId,具体的sparkcontext对象的runjob的源码,如下文所叙述。
附带Application,Driver,Job,Task,Stage介绍的一篇文章:点击打开链接
https://www.cnblogs.com/superhedantou/p/5699201.html
过程
1.sparkcontext.runjob 方法
方法主要调用dagschedular的runjob方法,将处理任务转至dagschedular进行上层任务调度阶段的处理,主要步骤包含:
(1)清理闭包(文末附带了介绍文章链接)
(2)dagScheduler.runJob
(3)rdd.doCheckpoint()
def runJob[T, U: ClassTag]( rdd: RDD[T], func: (TaskContext, Iterator[T]) => U, partitions: Seq[Int], resultHandler: (Int, U) => Unit): Unit = { if (stopped.get()) { throw new IllegalStateException("SparkContext has been shutdown") } val callSite = getCallSite val cleanedFunc = clean(func) logInfo("Starting job: " + callSite.shortForm) if (conf.getBoolean("spark.logLineage", false)) { logInfo("RDD's recursive dependencies:\n" + rdd.toDebugString) } //核心代码 dagScheduler.runJob(rdd, cleanedFunc, partitions, callSite, resultHandler, localProperties.get) progressBar.foreach(_.finishAll()) //任务完成磁盘存储 rdd.doCheckpoint() }
2.dagScheduler.runJob 方法
方法是调用submitJob方法提交任务,并等待结果处理,
def runJob[T, U]( rdd: RDD[T], func: (TaskContext, Iterator[T]) => U, partitions: Seq[Int], callSite: CallSite, resultHandler: (Int, U) => Unit, properties: Properties): Unit = { val start = System.nanoTime //提交job 其实是提交rdd和func任务 //submit其实是向另外一个loop消息线程提交,而不是网络提交 val waiter = submitJob(rdd, func, partitions, callSite, resultHandler, properties) //结果处理 ThreadUtils.awaitReady(waiter.completionFuture, Duration.Inf) waiter.completionFuture.value.get match { case scala.util.Success(_) => logInfo("Job %d finished: %s, took %f s".format (waiter.jobId, callSite.shortForm, (System.nanoTime - start) / 1e9)) case scala.util.Failure(exception) => logInfo("Job %d failed: %s, took %f s".format (waiter.jobId, callSite.shortForm, (System.nanoTime - start) / 1e9)) // SPARK-8644: Include user stack trace in exceptions coming from DAGScheduler. val callerStackTrace = Thread.currentThread().getStackTrace.tail exception.setStackTrace(exception.getStackTrace ++ callerStackTrace) throw exception } }
3.DagSchedular.submitJob() 方法
该方法的核心是,向eventLoop线程,发送提交任务请求,主要步骤为:
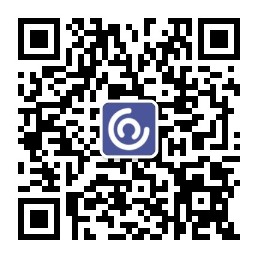
(1) 检验分区
(2) 为本job生成一个新的jobId
(3) eventProcessLoop.post 发送事件
def submitJob[T, U]( rdd: RDD[T], func: (TaskContext, Iterator[T]) => U, partitions: Seq[Int], callSite: CallSite, resultHandler: (Int, U) => Unit, properties: Properties): JobWaiter[U] = { // Check to make sure we are not launching a task on a partition that does not exist. //partitoin有效性检验 val maxPartitions = rdd.partitions.length partitions.find(p => p >= maxPartitions || p < 0).foreach { p => throw new IllegalArgumentException( "Attempting to access a non-existent partition: " + p + ". " + "Total number of partitions: " + maxPartitions) } //生成一个jobid val jobId = nextJobId.getAndIncrement() if (partitions.size == 0) { // Return immediately if the job is running 0 tasks return new JobWaiter[U](this, jobId, 0, resultHandler) } assert(partitions.size > 0) val func2 = func.asInstanceOf[(TaskContext, Iterator[_]) => _] val waiter = new JobWaiter(this, jobId, partitions.size, resultHandler) //发送线程消息 eventProcessLoop.post(JobSubmitted( jobId, rdd, func2, partitions.toArray, callSite, waiter, SerializationUtils.clone(properties))) waiter }
4.EventLoop
EventLoop核心是一个线程安全的LinkedBlockingQueue(介绍网址点击打开链接),向EventLoop提交job,实际上就是向LinkedBlockingQueue对象中添加一个job事件,每一个队列中的事件在处理的时候,会反向调用DAGSchedular.onReceive()方法,没错! DAGSchedular发送的消息给eventLoop,eventLoop又反向调用DAGSchedular,具体的原因可能如下:
5.dagScheduler.receive 方法
dagScheduler.onReceive()方式调用的doOnReceive方法,所以我们直接分析doOnReceive方法,doOnReceive根据不同的submit类型使用不同的handler处理,我们会匹配到JobSubmitted(jobId, rdd, func, partitions, callSite, listener, properties) ,调用了dagScheduler.handleJobSubmitted(jobId, rdd, func, partitions, callSite, listener, properties)处理方法,源码如下:
private def doOnReceive(event: DAGSchedulerEvent): Unit = event match { //我们此次分析所匹配到的case case JobSubmitted(jobId, rdd, func, partitions, callSite, listener, properties) => dagScheduler.handleJobSubmitted(jobId, rdd, func, partitions, callSite, listener, properties) case MapStageSubmitted(jobId, dependency, callSite, listener, properties) => dagScheduler.handleMapStageSubmitted(jobId, dependency, callSite, listener, properties) case StageCancelled(stageId, reason) => dagScheduler.handleStageCancellation(stageId, reason) case JobCancelled(jobId, reason) => dagScheduler.handleJobCancellation(jobId, reason) case JobGroupCancelled(groupId) => dagScheduler.handleJobGroupCancelled(groupId) //省略剩余代码 }
6.DagSchedular.handleJobSubmitted 方法
private[scheduler] def handleJobSubmitted(jobId: Int, finalRDD: RDD[_], func: (TaskContext, Iterator[_]) => _, partitions: Array[Int], callSite: CallSite, listener: JobListener, properties: Properties) { var finalStage: ResultStage = null try { // New stage creation may throw an exception if, for example, jobs are run on a // HadoopRDD whose underlying HDFS files have been deleted. // 创建最终stage // 1.createResultStage方法会创建所有依赖(直接或者间接依赖stage) // 2.关联jobid和Stage finalStage = createResultStage(finalRDD, func, partitions, jobId, callSite) } catch { case e: Exception => logWarning("Creating new stage failed due to exception - job: " + jobId, e) listener.jobFailed(e) return } //使用jobid创建job val job = new ActiveJob(jobId, finalStage, callSite, listener, properties) //清楚Rdd parttition缓存记录 clearCacheLocs() logInfo("Got job %s (%s) with %d output partitions".format( job.jobId, callSite.shortForm, partitions.length)) logInfo("Final stage: " + finalStage + " (" + finalStage.name + ")") logInfo("Parents of final stage: " + finalStage.parents) logInfo("Missing parents: " + getMissingParentStages(finalStage)) val jobSubmissionTime = clock.getTimeMillis() //记录job jobIdToActiveJob(jobId) = job activeJobs += job //设置active job finalStage.setActiveJob(job) val stageIds = jobIdToStageIds(jobId).toArray //stage 最后一次运行信息、重复运行次数的数据机构,当前只有失败以后重试的nextAttmptId val stageInfos = stageIds.flatMap(id => stageIdToStage.get(id).map(_.latestInfo)) //这其实是个广播事件的事件,凡事注册了SparkListenerJobStart事件的listner都会监听到消息 listenerBus.post( SparkListenerJobStart(job.jobId, jobSubmissionTime, stageInfos, properties)) //提交Stage submitStage(finalStage) }
7.DagSchedular.submitStage 方法
该方法会递归的调用自身,当前stage存在依赖的时候就会递归提交父stage,当不存在依赖父stage时候调用,submitMissingTasks()真正提交task。
private def submitStage(stage: Stage) { //是否关联jobID,在handleJobSubmitted方法中,我们创建了本次job的所有的stage,并且关联了本次jobid val jobId = activeJobForStage(stage) if (jobId.isDefined) { logDebug("submitStage(" + stage + ")") //验证stage是否已经提交过了 if (!waitingStages(stage) && !runningStages(stage) && !failedStages(stage)) { val missing = getMissingParentStages(stage).sortBy(_.id) logDebug("missing: " + missing) if (missing.isEmpty) { logInfo("Submitting " + stage + " (" + stage.rdd + "), which has no missing parents") //没有依赖的stage直接准备提交taskset submitMissingTasks(stage, jobId.get) } else { for (parent <- missing) { //存在依赖的stage,递归提交 submitStage(parent) } waitingStages += stage } } } else { abortStage(stage, "No active job for stage " + stage.id, None) } }
7.DagSchedular.submitMissingTasks 方法
private def submitMissingTasks(stage: Stage, jobId: Int) { logDebug("submitMissingTasks(" + stage + ")") // First figure out the indexes of partition ids to compute. val partitionsToCompute: Seq[Int] = stage.findMissingPartitions() // Use the scheduling pool, job group, description, etc. from an ActiveJob associated // with this Stage val properties = jobIdToActiveJob(jobId).properties runningStages += stage // SparkListenerStageSubmitted should be posted before testing whether tasks are // serializable. If tasks are not serializable, a SparkListenerStageCompleted event // will be posted, which should always come after a corresponding SparkListenerStageSubmitted // event. stage match { case s: ShuffleMapStage => outputCommitCoordinator.stageStart(stage = s.id, maxPartitionId = s.numPartitions - 1) case s: ResultStage => outputCommitCoordinator.stageStart( stage = s.id, maxPartitionId = s.rdd.partitions.length - 1) } //获取本地行信息 调用 getPreferredLocs方法 val taskIdToLocations: Map[Int, Seq[TaskLocation]] = try { stage match { case s: ShuffleMapStage => partitionsToCompute.map { id => (id, getPreferredLocs(stage.rdd, id))}.toMap case s: ResultStage => partitionsToCompute.map { id => val p = s.partitions(id) (id, getPreferredLocs(stage.rdd, p)) }.toMap } } catch { case NonFatal(e) => stage.makeNewStageAttempt(partitionsToCompute.size) listenerBus.post(SparkListenerStageSubmitted(stage.latestInfo, properties)) abortStage(stage, s"Task creation failed: $e\n${Utils.exceptionString(e)}", Some(e)) runningStages -= stage return } //更新stage的执行次数记录信息----- _latestInfo stage.makeNewStageAttempt(partitionsToCompute.size, taskIdToLocations.values.toSeq) //发送广播 listenerBus.post(SparkListenerStageSubmitted(stage.latestInfo, properties)) //此处省略一些task处理代码 //调用taskScheduler.submitTasks 提交tasksets if (tasks.size > 0) { //提交 taskScheduler.submitTasks(new TaskSet( tasks.toArray, stage.id, stage.latestInfo.attemptId, jobId, properties)) stage.latestInfo.submissionTime = Some(clock.getTimeMillis()) } else { // Because we posted SparkListenerStageSubmitted earlier, we should mark // the stage as completed here in case there are no tasks to run markStageAsFinished(stage, None) val debugString = stage match { case stage: ShuffleMapStage => s"Stage ${stage} is actually done; " + s"(available: ${stage.isAvailable}," + s"available outputs: ${stage.numAvailableOutputs}," + s"partitions: ${stage.numPartitions})" case stage : ResultStage => s"Stage ${stage} is actually done; (partitions: ${stage.numPartitions})" } logDebug(debugString) submitWaitingChildStages(stage) } }
7.DagSchedular.getPreferredLocs 方法
private[spark] def getPreferredLocs(rdd: RDD[_], partition: Int): Seq[TaskLocation] = { getPreferredLocsInternal(rdd, partition, new HashSet) }
7.DagSchedular.getPreferredLocsInternal方法
该方法为实际获取本地心信息的方法,通过该方法DAGSchedular 可以确定task具体的运行位置(ip,executorId),本地行信息获取顺序为:
(1) DagSchedular的内存数据结构中--cacheLocs
(2) 调用rdd自身的缓存信息确定,rdd.getPreferredLocations
(3)如果是窄依赖,则递归调用获取父rdd对应partition的本地行信息
private def getPreferredLocsInternal( rdd: RDD[_], partition: Int, visited: HashSet[(RDD[_], Int)]): Seq[TaskLocation] = { // 是否已经访问过 if (!visited.add((rdd, partition))) { // Nil has already been returned for previously visited partitions. return Nil } // 获取Schedular存储的缓存信息 // 1.获取DAGSchedular.cacheLocs结构存储了rdd对应的partition存储情况,有则直接返回 // 2.DAGSchedular还拥有blockManagerMaster对象,还可以从blockManager获取存储信息 val cached = getCacheLocs(rdd)(partition) if (cached.nonEmpty) { return cached } // 获取RDD自身的本地信息 val rddPrefs = rdd.preferredLocations(rdd.partitions(partition)).toList if (rddPrefs.nonEmpty) { return rddPrefs.map(TaskLocation(_)) } // 通过窄依赖获取父rdd对应partition的本地性信息 rdd.dependencies.foreach { case n: NarrowDependency[_] => for (inPart <- n.getParents(partition)) { val locs = getPreferredLocsInternal(n.rdd, inPart, visited) if (locs != Nil) { return locs } } case _ => } Nil }
总结
DAGSchedular 获取本次运行job信息,划分Stage,递归创建提交Stage,获取Task本地性运行信息,将Task提交给TaskScheudlar,交由下层调度管理。