文章目录
图像锐化:是一种用于改善图像质量的技术,它可以增强图像中的高频细节信息,从而使得图像更加清晰和有视觉冲击力。在图像处理和计算机视觉中,图像锐化通常被用于特征提取、图像增强、目标识别等应用中
一:图像边缘分析
图像边缘分析:是一种用于在图像中找到明显的边缘或轮廓的技术,它可以帮助识别图像中的物体边界、内部结构和纹理等特征。在图像处理和计算机视觉中,边缘分析通常被用于物体检测、目标跟踪、图像分割等应用中
图像中的边缘主要有以下几种类型
- 细线型边缘:
- 突变型边缘:
- 渐变型边缘:
如下图,各种边缘检测方法
- 细线型边缘:检测一阶微分过0点,二阶微分极值点
- 突变型边缘:检测一阶微分极值点,二阶微分过0点
- 渐变型边缘:难检测,二阶微分信息略多于一阶微分
二:一阶微分算子
(1)梯度算子
A:定义
梯度算子:是一类用于图像边缘检测和特征提取的算法,它们基于图像灰度值的变化来计算图像中各个位置的梯度信息,用于找到图像中明显的边缘或特征。对于函数图像 f ( x , y ) f(x,y) f(x,y),它在 ( x , y ) (x,y) (x,y)处的梯度为
G [ f ( x , y ) ] = [ ∂ f ∂ x ∂ f ∂ y ] T G[f(x, y)]=\left[\begin{array}{ll}\frac{\partial f}{\partial x} & \frac{\partial f}{\partial y}\end{array}\right]^{T} G[f(x,y)]=[∂x∂f∂y∂f]T
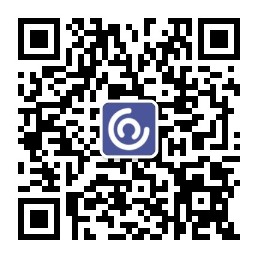
用梯度的幅度来代替,则为
G [ f ( x , y ) ] = [ ( ∂ f ∂ x ) 2 + ( ∂ f ∂ y ) 2 ] 1 2 或 G [ f ( x , y ) ] = ∣ ∂ f ∂ x ∣ + ∣ ∂ f ∂ y ∣ G[f(x, y)]=\left[\left(\frac{\partial f}{\partial x}\right)^{2}+\left(\frac{\partial f}{\partial y}\right)^{2}\right]^{\frac{1}{2}} \text { 或 } G[f(x, y)]=\left|\frac{\partial f}{\partial x}\right|+\left|\frac{\partial f}{\partial y}\right| G[f(x,y)]=[(∂x∂f)2+(∂y∂f)2]21 或 G[f(x,y)]= ∂x∂f + ∂y∂f
离散的数字矩阵,用差分来代替微分,其中 g ( x , y ) g(x,y) g(x,y)称为梯度图像
∂ f ∂ x = Δ f Δ x = f ( x + 1 , y ) − f ( x , y ) x + 1 − x = f ( x + 1 , y ) − f ( x , y ) ∂ f ∂ y = Δ f Δ y = f ( x , y + 1 ) − f ( x , y ) y + 1 − y = f ( x , y + 1 ) − f ( x , y ) g ( x , y ) = ∣ f ( x + 1 , y ) − f ( x , y ) ∣ + ∣ f ( x , y + 1 ) − f ( x , y ) ∣ \begin{array}{l}\frac{\partial f}{\partial x}=\frac{\Delta f}{\Delta x}=\frac{f(x+1, y)-f(x, y)}{x+1-x}=f(x+1, y)-f(x, y) \\\frac{\partial f}{\partial y}=\frac{\Delta f}{\Delta y}=\frac{f(x, y+1)-f(x, y)}{y+1-y}=f(x, y+1)-f(x, y) \\g(x, y)=|f(x+1, y)-f(x, y)|+|f(x, y+1)-f(x, y)|\end{array} ∂x∂f=ΔxΔf=x+1−xf(x+1,y)−f(x,y)=f(x+1,y)−f(x,y)∂y∂f=ΔyΔf=y+1−yf(x,y+1)−f(x,y)=f(x,y+1)−f(x,y)g(x,y)=∣f(x+1,y)−f(x,y)∣+∣f(x,y+1)−f(x,y)∣
B:边缘检测
- 对梯度图像进行阈值化,检测局部变化极值
固定边界灰度:
g ( x , y ) = { L G G [ f ( x , y ) ] ≥ T f ( x , y ) 其他 g(x, y)=\left\{\begin{array}{lc}L_{G} & G[f(x, y)] \geq T \\f(x, y) & \text { 其他 }\end{array}\right. g(x,y)={ LGf(x,y)G[f(x,y)]≥T 其他
突出边界:
g ( x , y ) = { G [ f ( x , y ) ] G [ f ( x , y ) ] ≥ T f ( x , y ) 其他 g(x, y)=\left\{\begin{array}{lc}G[f(x, y)] & G[f(x, y)] \geq T \\f(x, y) & \text { 其他 }\end{array}\right. g(x,y)={ G[f(x,y)]f(x,y)G[f(x,y)]≥T 其他
二值化边界与背景:
g ( x , y ) = { L G G [ f ( x , y ) ] ≥ T L B 其他 g(x, y)=\left\{\begin{array}{lc}L_{G} & G[f(x, y)] \geq T \\L_{B} & \text { 其他 }\end{array}\right. g(x,y)={ LGLBG[f(x,y)]≥T 其他
C:示例
如下为一个计算示例
D:程序
matlab实现:
Image=im2double(rgb2gray(imread('lotus.jpg')));
subplot(131),imshow(Image),title('原图像');
[h,w]=size(Image);
edgeImage=zeros(h,w);
for x=1:w-1
for y=1:h-1
edgeImage(y,x)=abs(Image(y,x+1)-Image(y,x))+abs(Image(y+1,x)-Image(y,x));
end
end
subplot(132),imshow(edgeImage),title('梯度图像');
sharpImage=Image+edgeImage;
subplot(133),imshow(sharpImage),title('锐化图像');
Python实现:
import cv2
import numpy as np
from matplotlib import pyplot as plt
# 读取图像
img = cv2.imread('lotus.jpg')
# 将图像转为灰度图像并将像素值缩放到[0,1]之间
gray = cv2.cvtColor(img, cv2.COLOR_BGR2GRAY)
gray = gray.astype(np.float64) / 255
# 显示原图像
plt.subplot(131)
plt.imshow(gray, cmap='gray')
plt.title('原图像')
# 计算梯度图像
h, w = gray.shape
edge_img = np.zeros((h, w))
for x in range(w-1):
for y in range(h-1):
edge_img[y,x] = abs(gray[y,x+1]-gray[y,x]) + abs(gray[y+1,x]-gray[y,x])
# 显示梯度图像
plt.subplot(132)
plt.imshow(edge_img, cmap='gray')
plt.title('梯度图像')
# 锐化图像
sharp_img = gray + edge_img
sharp_img = np.clip(sharp_img, 0, 1)
# 显示锐化图像
plt.subplot(133)
plt.imshow(sharp_img, cmap='gray')
plt.title('锐化图像')
# 显示所有图像
plt.show()
(2)Robert算子
A:定义
Robert算子:是一种边缘检测算子,其原理基于图像中像素值的差异。该算子的实现使用了两个 2 × 2 2 \times 2 2×2 的卷积核 G x G_x Gx 和 G y G_y Gy,分别计算像素点 ( x , y ) (x,y) (x,y) 和 ( x + 1 , y + 1 ) (x+1,y+1) (x+1,y+1) 之间的灰度差异。具体来说, G x G_x Gx 和 G y G_y Gy 的取值如下
[ 1 0 0 − 1 ] 和 [ 0 1 − 1 0 ] \begin{bmatrix} 1 & 0 \\ 0 & -1\end{bmatrix} 和\begin{bmatrix} 0 & 1 \\ -1 & 0\end{bmatrix} [100−1]和[0−110]
然后,对于输入图像 I I I,可以通过以下公式计算其边缘强度 E E E
*
表示卷积运算
E ( x , y ) = ( I ( x , y ) ∗ G x ) 2 + ( I ( x , y ) ∗ G y ) 2 E(x, y)=\sqrt{\left(I(x, y) * G_{x}\right)^{2}+\left(I(x, y) * G_{y}\right)^{2}} E(x,y)=(I(x,y)∗Gx)2+(I(x,y)∗Gy)2
最终得到的边缘强度 E E E 可以用来检测图像中的边缘,边缘通常在 E E E 取得较大值的地方出现。此外,为了提高计算效率,通常也可以使用预先计算好的卷积核来实现 Robert 算子
B:示例
如下图为一计算示例
C:程序
edge
函数:用于在图像中检测边缘并生成二值化的边缘图像。该函数语法如下
BW = edge(I, method, threshold, direction)
参数含义如下
I
:输入图像,可以是灰度图像或彩色图像。对于彩色图像,通常会先将其转换为灰度图像,然后再进行边缘检测method
:边缘检测的方法,包括以下几种,默认值为'sobel'
'sobel'
:Sobel算子检测边缘'prewitt'
:Prewitt算子检测边缘'roberts'
:Roberts算子检测边缘'log'
:Laplacian of Gaussian算子检测边缘'zerocross'
:使用Laplacian算子和零交叉检测边缘'canny'
:使用Canny算子检测边缘
threshold
:二值化的阈值,用于将检测到的边缘转换为二值化图像。对于Canny算子,此参数可以是包含两个元素的向量,分别表示低阈值和高阈值。默认值为0.2direction
:边缘的检测方向,包括以下几种,默认值为'both'
'both'
:检测水平和垂直方向的边缘'horizontal'
:仅检测水平方向的边缘'vertical'
:仅检测垂直方向的边缘
matlab实现:
Image=im2double(rgb2gray(imread('lotus.jpg')));
% 将名为 lotus.jpg 的彩色图像读入并转换为灰度图像,然后将其类型转换为double型,存储在变量 Image 中。
figure,imshow(Image),title('原图像');
% 显示原始图像
BW= edge(Image,'roberts');
% 对图像进行 Robert 边缘检测,得到一个二值化的边缘图像,存储在变量 BW 中。
figure,imshow(BW),title('边缘检测');
% 显示 Robert 边缘检测结果
H1=[1 0; 0 -1];
H2=[0 1;-1 0];
% 定义两个 2×2 的卷积核 H1 和 H2,分别为 Robert 算子的两个分量。
R1=imfilter(Image,H1);
R2=imfilter(Image,H2);
% 对原始图像分别使用 H1 和 H2 进行卷积操作,得到两个梯度图像 R1 和 R2。
edgeImage=abs(R1)+abs(R2);
% 将 R1 和 R2 两个梯度图像的绝对值相加,得到最终的梯度图像,存储在变量 edgeImage 中。
figure,imshow(edgeImage),title('Robert梯度图像');
% 显示 Robert 算子得到的梯度图像
sharpImage=Image+edgeImage;
% 将原始图像与梯度图像相加,得到锐化后的图像,存储在变量 sharpImage 中。
figure,imshow(sharpImage),title('Robert锐化图像');
% 显示锐化后的图像
Python实现:
import cv2
import numpy as np
from matplotlib import pyplot as plt
# 读入图像并转换为灰度图像
img = cv2.imread('lotus.jpg')
gray = cv2.cvtColor(img, cv2.COLOR_BGR2GRAY)
gray = gray.astype(np.float64) / 255.0
# 显示原图像
plt.imshow(gray, cmap='gray')
plt.title('原图像')
plt.show()
# 进行边缘检测
edge = cv2.Canny(gray, 100, 200)
# 显示边缘检测结果
plt.imshow(edge, cmap='gray')
plt.title('边缘检测')
plt.show()
# 定义两个 2×2 的卷积核 H1 和 H2,分别为 Robert 算子的两个分量
H1 = np.array([[1, 0], [0, -1]])
H2 = np.array([[0, 1], [-1, 0]])
# 对原始图像分别使用 H1 和 H2 进行卷积操作,得到两个梯度图像 R1 和 R2
R1 = cv2.filter2D(gray, -1, H1)
R2 = cv2.filter2D(gray, -1, H2)
# 将 R1 和 R2 两个梯度图像的绝对值相加,得到最终的梯度图像
edgeImage = cv2.addWeighted(cv2.convertScaleAbs(R1), 0.5, cv2.convertScaleAbs(R2), 0.5, 0)
# 显示 Robert 算子得到的梯度图像
plt.imshow(edgeImage, cmap='gray')
plt.title('Robert梯度图像')
plt.show()
# 将原始图像与梯度图像相加,得到锐化后的图像
sharpImage = cv2.addWeighted(gray, 1, edgeImage, 1, 0)
# 显示锐化后的图像
plt.imshow(sharpImage, cmap='gray')
plt.title('Robert锐化图像')
plt.show()
(3)Sobel算子
A:定义
Sobel算子:是一种常用的边缘检测算子,用于在数字图像中检测出边缘部分。它使用两个 3 × 3 3 \times 3 3×3的卷积核,分别对图像在 x x x和 y y y方向进行卷积操作,从而计算出每个像素点处的梯度大小和方向。 x x x和 y y y方向的卷积核可以表示为
H x = [ − 1 − 2 − 1 0 0 0 1 2 1 ] 和 H y = [ − 1 0 1 − 2 0 − 2 − 1 0 1 ] H_{x}=\begin{bmatrix} -1 & -2 & -1\\ 0 & 0 & 0\\ 1 & 2 & 1 \end{bmatrix}和H_{y}=\begin{bmatrix} -1 & 0 & 1\\ -2 & 0 & -2\\ -1 & 0 & 1 \end{bmatrix} Hx= −101−202−101 和Hy= −1−2−10001−21
对于一个灰度图像 I I I,对其在 x x x方向应用Sobel算子,可以得到一个新的图像 G x G_x Gx,其中每个像素的值表示其在 x x x方向上的梯度大小,即
G x ( i , j ) = ∑ m = − 1 1 ∑ n = − 1 1 H x ( m + 2 , n + 2 ) I ( i + m , j + n ) G_{x}(i, j)=\sum_{m=-1}^{1} \sum_{n=-1}^{1} H_{x}(m+2, n+2) I(i+m, j+n) Gx(i,j)=m=−1∑1n=−1∑1Hx(m+2,n+2)I(i+m,j+n)
类似地,对图像 I I I在 y y y方向应用Sobel算子,可以得到一个新的图像 G y G_y Gy,其中每个像素的值表示其在 y y y方向上的梯度大小,即
G y ( i , j ) = ∑ m = − 1 1 ∑ n = − 1 1 H y ( m + 2 , n + 2 ) I ( i + m , j + n ) G_{y}(i, j)=\sum_{m=-1}^{1} \sum_{n=-1}^{1} H_{y}(m+2, n+2) I(i+m, j+n) Gy(i,j)=m=−1∑1n=−1∑1Hy(m+2,n+2)I(i+m,j+n)
最终的梯度图像 G G G可以通过 G x G_x Gx和 G y G_y Gy的平方和再开方得到
G ( i , j ) = G x ( i , j ) 2 + G y ( i , j ) 2 G(i,j)=\sqrt{ G_{x}(i,j)^{2}+G_{y}(i,j)^{2} } G(i,j)=Gx(i,j)2+Gy(i,j)2
Sobel算子的目的是找到图像中灰度变化剧烈的位置,也就是边缘。通过比较每个像素点的梯度大小和方向,可以将图像中的边缘部分提取出来,方便后续的图像分析和处理
B:示例
如下图为一计算示例
C:程序
matlab实现:
Image=im2double(rgb2gray(imread('lotus.jpg')));
figure,imshow(Image),title('原图像');
BW= edge(Image,'sobel');
figure,imshow(BW),title('边缘检测');
H1=[-1 -2 -1;0 0 0;1 2 1];
H2=[-1 0 1;-2 0 2;-1 0 1];
R1=imfilter(Image,H1);
R2=imfilter(Image,H2);
edgeImage=abs(R1)+abs(R2);
figure,imshow(edgeImage),title('Sobel梯度图像');
sharpImage=Image+edgeImage;
figure,imshow(sharpImage),title('Sobel锐化图像');
Python实现:
import cv2
import numpy as np
import matplotlib.pyplot as plt
# 读入图像并转换为灰度图
image = cv2.imread('lotus.jpg')
image_gray = cv2.cvtColor(image, cv2.COLOR_BGR2GRAY)
image_gray = cv2.normalize(image_gray.astype('float'), None, 0.0, 1.0, cv2.NORM_MINMAX)
# 显示原图像
plt.imshow(image_gray, cmap='gray')
plt.title('原图像')
plt.show()
# 边缘检测
edge_image = cv2.Sobel(image_gray, cv2.CV_64F, 1, 0) + cv2.Sobel(image_gray, cv2.CV_64F, 0, 1)
edge_image = cv2.normalize(np.abs(edge_image), None, 0.0, 1.0, cv2.NORM_MINMAX)
# 显示边缘检测结果
plt.imshow(edge_image, cmap='gray')
plt.title('边缘检测')
plt.show()
# Sobel梯度图像
H1 = np.array([[-1, -2, -1], [0, 0, 0], [1, 2, 1]])
H2 = np.array([[-1, 0, 1], [-2, 0, 2], [-1, 0, 1]])
R1 = cv2.filter2D(image_gray, -1, H1)
R2 = cv2.filter2D(image_gray, -1, H2)
sobel_image = np.abs(R1) + np.abs(R2)
sobel_image = cv2.normalize(sobel_image, None, 0.0, 1.0, cv2.NORM_MINMAX)
# 显示Sobel梯度图像
plt.imshow(sobel_image, cmap='gray')
plt.title('Sobel梯度图像')
plt.show()
# Sobel锐化图像
sharp_image = image_gray + sobel_image
sharp_image = cv2.normalize(sharp_image, None, 0.0, 1.0, cv2.NORM_MINMAX)
# 显示Sobel锐化图像
plt.imshow(sharp_image, cmap='gray')
plt.title('Sobel锐化图像')
plt.show()
(4)Prewitt算子
A:定义
Prewitt算子:是一种经典的图像边缘检测算子,用于检测图像中的水平和垂直边缘。它是一种离散型微分算子,通过对图像像素值的梯度计算来提取边缘信息。对于一个灰度图像 I I I,Prewitt算子分别对图像的水平和垂直方向计算梯度,得到两个梯度图像 G x G_x Gx 和 G y G_y Gy。这些梯度图像的元素值表示在每个像素处的梯度大小和方向。Prewitt算子的水平和垂直模板分别为
H x = [ − 1 0 1 − 1 0 1 − 1 0 1 ] 和 H y = [ − 1 − 1 − 1 − 0 0 0 1 1 1 ] H_{x}=\begin{bmatrix} -1 & 0 & 1\\ -1 & 0 & 1\\ -1 & 0 & 1 \end{bmatrix}和H_{y}=\begin{bmatrix} -1 & -1 & -1\\ -0 & 0 & 0\\ 1 & 1 & 1 \end{bmatrix} Hx= −1−1−1000111 和Hy= −1−01−101−101
水平和垂直梯度图像的计算公式为:
*
表示卷积运算
G x = I ∗ H x , G y = I ∗ H y G_{x}=I*H_{x},G_{y}=I*H_{y} Gx=I∗Hx,Gy=I∗Hy
最后,可以将水平和垂直梯度图像结合起来计算边缘梯度图像 G G G:
G = G x 2 + G y 2 G=\sqrt{ G^{2}_{x}+G^{2}_{y} } G=Gx2+Gy2
根据梯度大小和方向的信息,可以通过设置一个阈值来判断像素是否为边缘,从而提取出图像中的边缘信息
B:示例
C:程序
matlab实现:
clear,clc,close all;
Image=im2double(rgb2gray(imread('lotus.jpg')));
H1=[-1 -1 -1;0 0 0;1 1 1];
H2=[0 -1 -1;1 0 -1; 1 1 0];
H3=[1 0 -1;1 0 -1;1 0 -1];
H4=[1 1 0;1 0 -1;0 -1 -1];
H5=[1 1 1;0 0 0;-1 -1 -1];
H6=[0 1 1;-1 0 1;-1 -1 0];
H7=[-1 0 1;-1 0 1;-1 0 1];
H8=[-1 -1 0;-1 0 1;0 1 1];
R1=imfilter(Image,H1);
R2=imfilter(Image,H2);
R3=imfilter(Image,H3);
R4=imfilter(Image,H4);
R5=imfilter(Image,H5);
R6=imfilter(Image,H6);
R7=imfilter(Image,H7);
R8=imfilter(Image,H8);
edgeImage1=abs(R1)+abs(R7);
sharpImage1=edgeImage1+Image;
f1=max(max(R1,R2),max(R3,R4));
f2=max(max(R5,R6),max(R7,R8));
edgeImage2=max(f1,f2);
sharpImage2=edgeImage2+Image;
subplot(221),imshow(edgeImage1),title('两个模板边缘检测');
subplot(222),imshow(edgeImage2),title('八个模板边缘检测');
subplot(223),imshow(sharpImage1),title('两个模板边缘锐化');
subplot(224),imshow(sharpImage2),title('八个模板边缘锐化');
Python实现:
import cv2
import matplotlib.pyplot as plt
import numpy as np
# 读取图像并转换为灰度图像
image = cv2.imread('lotus.jpg')
image = cv2.cvtColor(image, cv2.COLOR_BGR2GRAY)
image = np.float32(image) / 255
# 定义边缘检测和边缘锐化的卷积核
H1 = np.array([[-1, -1, -1], [0, 0, 0], [1, 1, 1]])
H2 = np.array([[0, -1, -1], [1, 0, -1], [1, 1, 0]])
H3 = np.array([[1, 0, -1], [1, 0, -1], [1, 0, -1]])
H4 = np.array([[1, 1, 0], [1, 0, -1], [0, -1, -1]])
H5 = np.array([[1, 1, 1], [0, 0, 0], [-1, -1, -1]])
H6 = np.array([[0, 1, 1], [-1, 0, 1], [-1, -1, 0]])
H7 = np.array([[-1, 0, 1], [-1, 0, 1], [-1, 0, 1]])
H8 = np.array([[-1, -1, 0], [-1, 0, 1], [0, 1, 1]])
# 对图像进行卷积操作,得到八个卷积结果
R1 = cv2.filter2D(image, -1, H1)
R2 = cv2.filter2D(image, -1, H2)
R3 = cv2.filter2D(image, -1, H3)
R4 = cv2.filter2D(image, -1, H4)
R5 = cv2.filter2D(image, -1, H5)
R6 = cv2.filter2D(image, -1, H6)
R7 = cv2.filter2D(image, -1, H7)
R8 = cv2.filter2D(image, -1, H8)
# 计算两个模板和八个模板的边缘检测结果和边缘锐化结果
edgeImage1 = np.abs(R1) + np.abs(R7)
sharpImage1 = edgeImage1 + image
f1 = np.maximum(np.maximum(R1, R2), np.maximum(R3, R4))
f2 = np.maximum(np.maximum(R5, R6), np.maximum(R7, R8))
edgeImage2 = np.maximum(f1, f2)
sharpImage2 = edgeImage2 + image
# 显示图像
plt.subplot(221), plt.imshow(edgeImage1, cmap='gray'), plt.title('两个模板边缘检测')
plt.subplot(222), plt.imshow(edgeImage2, cmap='gray'), plt.title('八个模板边缘检测')
plt.subplot(223), plt.imshow(sharpImage1, cmap='gray'), plt.title('两个模板边缘锐化')
plt.subplot(224), plt.imshow(sharpImage2, cmap='gray',plt.title('八个模板边缘锐化')
三:二阶微分算子
(1)定义
二阶微分算子(拉普拉斯算子):在三维欧几里得空间中,拉普拉斯算子通常表示为 Δ \Delta Δ,它可以被定义为向量算子 ∇ \nabla ∇ 的散度的运算,即
Δ 2 f = ∂ 2 f ∂ x 2 + ∂ 2 f ∂ y 2 \Delta^{2} f=\frac{\partial^{2} f}{\partial x^{2}}+\frac{\partial^{2} f}{\partial y^{2}} Δ2f=∂x2∂2f+∂y2∂2f
其中
∂ 2 f ∂ x 2 = Δ x f ( x + 1 , y ) − Δ x f ( x , y ) = [ f ( x + 1 , y ) − f ( x , y ) ] − [ f ( x , y ) − f ( x − 1 , y ) ] = f ( x + 1 , y ) + f ( x − 1 , y ) − 2 f ( x , y ) ∂ 2 f ∂ y 2 = Δ y f ( x , y + 1 ) − Δ y f ( x , y ) = [ f ( x , y + 1 ) − f ( x , y ) ] − [ f ( x , y ) − f ( x , y − 1 ) ] = f ( x , y + 1 ) + f ( x , y − 1 ) − 2 f ( x , y ) \begin{aligned}\frac{\partial^{2} f}{\partial x^{2}} & =\Delta_{x} f(x+1, y)-\Delta_{x} f(x, y) \\& =[f(x+1, y)-f(x, y)]-[f(x, y)-f(x-1, y)] \\& =f(x+1, y)+f(x-1, y)-2 f(x, y) \\\frac{\partial^{2} f}{\partial y^{2}} & =\Delta_{y} f(x, y+1)-\Delta_{y} f(x, y) \\& =[f(x, y+1)-f(x, y)]-[f(x, y)-f(x, y-1)] \\& =f(x, y+1)+f(x, y-1)-2 f(x, y)\end{aligned} ∂x2∂2f∂y2∂2f=Δxf(x+1,y)−Δxf(x,y)=[f(x+1,y)−f(x,y)]−[f(x,y)−f(x−1,y)]=f(x+1,y)+f(x−1,y)−2f(x,y)=Δyf(x,y+1)−Δyf(x,y)=[f(x,y+1)−f(x,y)]−[f(x,y)−f(x,y−1)]=f(x,y+1)+f(x,y−1)−2f(x,y)
(2)示例
如下图为示例
(3)程序
matlab实现:
Image=im2double(rgb2gray(imread('lotus.jpg')));
figure,imshow(Image),title('原图像');
H=fspecial('laplacian',0);
R=imfilter(Image,H);
edgeImage=abs(R);
figure,imshow(edgeImage),title('Laplacian梯度图像');
H1=[0 -1 0;-1 5 -1;0 -1 0];
sharpImage=imfilter(Image,H1);
figure,imshow(sharpImage),title('Laplacian锐化图像');
Python实现:
import cv2
import matplotlib.pyplot as plt
Image = cv2.imread('lotus.jpg')
Image = cv2.cvtColor(Image, cv2.COLOR_BGR2GRAY)
Image = cv2.normalize(Image.astype('float'), None, 0.0, 1.0, cv2.NORM_MINMAX)
plt.imshow(Image, cmap='gray')
plt.title('原图像')
plt.show()
H = cv2.Laplacian(Image, ddepth=-1, ksize=3)
R = cv2.filter2D(Image, -1, H)
edgeImage = cv2.convertScaleAbs(R)
plt.imshow(edgeImage, cmap='gray')
plt.title('Laplacian梯度图像')
plt.show()
H1 = np.array([[0, -1, 0], [-1, 5, -1], [0, -1, 0]])
sharpImage = cv2.filter2D(Image, -1, H1)
plt.imshow(sharpImage, cmap='gray')
plt.title('Laplacian锐化图像')
plt.show()