目前在网上看到过各种从0到1训练一个LLM或是miniLLM之类的项目,但是发现好像还没有类似的VLM项目,正好最近计算资源比较充足,所以尝试对llava进行了完整的复现(应该也算是从0到1了吧),最终得到了一个在MMMU上得分超越llava-1.5的模型。
本项目使用qwen2-7b-instruct模型代替llava-1.5-7b的LLM,其他结构和原始llava相同,最终得到的模型我命名为Qllava-7b。训练数据和llava原论文对齐,使用官方提供的pretrain和sft数据,二者都可以在hugging face上找到。值得一提的是官方提供的hugging face上的sft数据里,有关ocr_vqa的部分格式有错误,在读取json时会报错,考虑到ocr_vqa的数据只有8w条,因此在qllava训练时直接从665k的sft数据中去掉了这部分数据。最终训练的框架选择了Llama-Factory,评测框架选择lmms-eval。接下来会给大家介绍项目细节,主要是介绍本项目改动的部分,默认读者对transformers和llama-factory框架的源码以及结构有基础的了解。
1.效果展示
使用lmms-eval在mmmu上对qllava测评得分41.44,OpenCompass 榜单上llava-1.5-7b的mmmu得分35.7。
其他case的推理结果懒得放了。需要注意的是,官方的llava-1.5-7b的mmmu得分在35左右,但是hugging face提供的llava-hf模型在mmmu只能得到20多分,我看到很多类似的issue提出这个问题了,两者的差异在于图片处理的不同,在resize的时候官方的仓库会对图片进行pad,而hugging face的image process中则没有对图片进行pad操作(可能记反了,大致就是一个pad了一个没pad)。本项目的qllava是基于hugging face的llava-hf模型进行的修改,所以image process过程和hf对齐。
2.数据准备
在llava官方仓库中有给出数据的地址:
https://github.com/haotian-liu/LLaVA
预训练数据:在hugging face上有两版pretrain数据,我们使用的是558k的版本,通过这个链接可以同时下载图片和标注的json数据,然后解压图片即可。
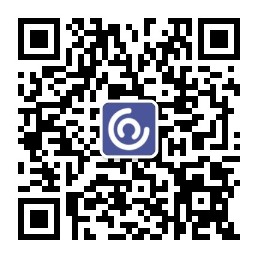
https://huggingface.co/datasets/liuhaotian/LLaVA-Pretrain
sft数据:在hugging face上下载mix665k的json数据,这里只包含了json文件,没有带任何的图片。
https://huggingface.co/datasets/liuhaotian/LLaVA-Instruct-150K/blob/main/llava_v1_5_mix665k.json
根据官方github仓库的指引去下载所有需要的图片,并且解压整理成指定的格式:
最后把hugging face上下载的llava_v1_5_mix665k.json和图片放在同一目录下即可,即最终json文件、coco、qga、ocr_vqa、textvqa、vg这些处于同一目录下。
注意:在数据收集阶段,我有遇到过以下的一些问题:
-
1.pretrain数据中带有图片,hugging face上下载太慢,可以考虑用hf镜像。
-
2.官方给出的各个图片的下载地址基本都是在外网,下载速度很慢,可以考虑国内各种数据收集平台有没有这些数据,例如上海ailab的OpenDataLab之类。
-
3.llava_v1_5_mix665k.json如果直接用dataset.load加载会出现json无法解析的报错,问题出在给出的数据集中,有一些数据的key是int类型的而不是str类型,可以做一个简单的过滤:先读取整个json,然后遍历每条数据,如果key不是str类型就过滤,再重新保存成json。
-
4.官方提供的ocr_vqa数据是他们自己整理过的,保存成了jpg数据,而原始数据中图片不是jpg格式的。而且3中出现问题的key好像都是ocr_vqa的数据,所以最后把ocr_vqa的8w条数据全部过滤了。
3.模型实现
鉴于hugging face上模型的通用性,以及现在大部分训练框架都能适配hf模型,因此本项目决定在hf模型的基础上进行魔改。首先看一下llava-hf模型在transformers库中是如何构建的:
transfomres中的llava
-
1.configuration_llava中实现了llava类的config,以便于通过from_config的方法来通过config初始化模型。
-
2.modeling_llava中构建了llava模型的架构,实现了llavaForConditionalGeneration这个类。
-
3.processing_llava中实现了llava的数据处理过程,包括文本的toknizer和图片image process。
-
4.convert_weight用于把官方的权重转换成hf模型的权重,由于我们从头训练,因此不需要这部分。
为了实现我们自己的qllava模型,我们可以首先完成modeling_qllava.py,在其中定义好我们的模型类。然后根据模型类需要的参数来构建config,即实现configuration_qllava.py,最后再考虑数据的处理即可。
4.modeling_qllava实现
我们的qllava相比llava只是更改了LLM,因此整体结构不会变化太大,可以基本复用modeling_llava.py的内容。首先把hugging face中的modeling_llava.py copy过来,并把其中所有的llava字段改成qllava,然后修改QllavaPreTrainedModel中的config_class = QllavaConfig,这代表我们使用的config的类,具体的QllavaConfig实现在下一部分。
最后我们修改QllavaForConditionalGeneration的__init__方法:
### 这里只放了init方法,其它不用修改所以没有放上来 ###
class QllavaForConditionalGeneration(QllavaPreTrainedModel, GenerationMixin):
def __init__(self, config: QllavaConfig):
super().__init__(config)
if config.train_from_scratch:
logger.info("loading vision model from pretrained")
self.vision_tower = CLIPVisionModel.from_pretrained(config.vision_model)
config.vision_config = self.vision_tower.config
logger.info("loading language model from pretrained")
self.language_model = AutoModelForCausalLM.from_pretrained(
config.text_model, attn_implementation=config._attn_implementation
)
config.text_config = self.language_model.config
else:
self.vision_tower = CLIPVisionModel._from_config(config.vision_config)
self.language_model = AutoModelForCausalLM.from_config(
config.text_config, attn_implementation=config._attn_implementation
)
self.multi_modal_projector = QllavaMultiModalProjector(config)
self.vocab_size = config.text_config.vocab_size
self.pad_token_id = self.config.pad_token_id if self.config.pad_token_id is not None else -1
self.post_init()
主要是修改了__init__方法,因为我们从头开始训练的话,需要加载clip和qwen2的权重作为初始化参数,所以在config中引入train_from_scratch参数,如果为true则qllava初始化的时候需要加载指定的vision_model和text_model作为vision tower和LLM,若为false,则代表我们已经有一个完整的qllava权重了,因此只需要使用from_config方法初始化模型,后续load整个权重即可,避免先load clip和qwen2,再load qllava的权重。
5.configuration_llava实现
"""Qwen-Llava model configuration"""
from transformers.configuration_utils import PretrainedConfig
from transformers.utils import logging
from transformers.models.auto import CONFIG_MAPPING
logger = logging.get_logger(__name__)
class QllavaConfig(PretrainedConfig):
r"""
Args:
vision_config (`Union[AutoConfig, dict]`, *optional*, defaults to `CLIPVisionConfig`):
The config object or dictionary of the vision backbone.
text_config (`Union[AutoConfig, dict]`, *optional*, defaults to `LlamaConfig`):
The config object or dictionary of the text backbone.
ignore_index (`int`, *optional*, defaults to -100):
The ignore index for the loss function.
image_token_index (`int`, *optional*, defaults to 32000):
The image token index to encode the image prompt.
projector_hidden_act (`str`, *optional*, defaults to `"gelu"`):
The activation function used by the multimodal projector.
vision_feature_select_strategy (`str`, *optional*, defaults to `"default"`):
The feature selection strategy used to select the vision feature from the vision backbone.
Can be one of `"default"` or `"full"`.
vision_feature_layer (`int`, *optional*, defaults to -2):
The index of the layer to select the vision feature.
image_seq_length (`int`, *optional*, defaults to 576):
Sequence length of one image embedding.
"""
model_type = "qllava" ### 这里需要修改成我们自己的模型类名,后续会用到
is_composition = True
def __init__(
self,
vision_model=None, ### 新增
text_model=None, ### 新增
vision_config=None,
text_config=None,
ignore_index=-100,
image_token_index=151655, # llava 默认为32000,这里我们使用qwen2的
projector_hidden_act="gelu",
vision_feature_select_strategy="default",
vision_feature_layer=-2,
image_seq_length=576,
train_from_scratch=False, ###新增
**kwargs,
):
self.ignore_index = ignore_index
self.image_token_index = image_token_index
self.projector_hidden_act = projector_hidden_act
self.image_seq_length = image_seq_length
if vision_feature_select_strategy not in ["default", "full"]:
raise ValueError(
"vision_feature_select_strategy should be one of 'default', 'full'."
f"Got: {vision_feature_select_strategy}"
)
self.vision_feature_select_strategy = vision_feature_select_strategy
self.vision_feature_layer = vision_feature_layer
if isinstance(vision_config, dict):
vision_config["model_type"] = (
vision_config["model_type"] if "model_type" in vision_config else "clip_vision_model"
)
vision_config = CONFIG_MAPPING[vision_config["model_type"]](**vision_config)
elif vision_config is None:
vision_config = CONFIG_MAPPING["clip_vision_model"](
intermediate_size=4096,
hidden_size=1024,
patch_size=14,
image_size=336,
num_hidden_layers=24,
num_attention_heads=16,
vocab_size=32000,
projection_dim=768,
)
self.vision_config = vision_config
if isinstance(text_config, dict):
text_config["model_type"] = text_config["model_type"] if "model_type" in text_config else "qwen2"
text_config = CONFIG_MAPPING[text_config["model_type"]](**text_config)
elif text_config is None:
text_config = CONFIG_MAPPING["qwen2"]()
self.text_config = text_config
self.vision_model = vision_model
self.text_model = text_model
self.train_from_scratch = train_from_scratch
super().__init__(**kwargs)
一些关键的新增加的代码在上面进行了注释,主要的变化有2点。
首先是匹配新的tokenizer,将image_token_index的默认值换成我们所使用的qwen2的image_token_index,印象中qwen2的语言模型所使用的tokenizer没有假加入image pad这些special token,但是qwen 2.5以后的所有的模型都是统一的tokenizer,里面同时包含了image video tool code等功能需要的special token,最终qllava项目使用的tokenizer来自qwen2-vl。
其次就是加入了train_from_scratch和vision_model、text_model,因为我们的项目是从0开始训练,初始化的qllava模型应该加载clip的img encoder和qwen2-7b的参数,在模型init时会起到作用(见上一部分)。
6.processing_qllava.py的实现
和modeling差不多,基本copy llava的过来稍微改一下就好~(指把llava替换为qllava)。因为我们使用了和llava相同的vision tower,所以image process完全相同,而hugging face中不同模型的tokenizer都使用相同的api,所以文本处理过程也相同。最后需要修改的一个地方是QllavaProcessor的__init__参数image_token,将默认值改成qwen2的image token即可。
from typing import List, Union
from transformers.feature_extraction_utils import BatchFeature
from transformers.image_utils import ImageInput, get_image_size, to_numpy_array
from transformers.processing_utils import ProcessingKwargs, ProcessorMixin, Unpack, _validate_images_text_input_order
from transformers.tokenization_utils_base import PreTokenizedInput, TextInput
from transformers.utils import logging
logger = logging.get_logger(__name__)
class QllavaProcessorKwargs(ProcessingKwargs, total=False):
_defaults = {
"text_kwargs": {
"padding": False,
},
"images_kwargs": {},
}
class QllavaProcessor(ProcessorMixin):
r"""
Constructs a Llava processor which wraps a Llava image processor and a Llava tokenizer into a single processor.
[`LlavaProcessor`] offers all the functionalities of [`CLIPImageProcessor`] and [`LlamaTokenizerFast`]. See the
[`~LlavaProcessor.__call__`] and [`~LlavaProcessor.decode`] for more information.
Args:
image_processor ([`CLIPImageProcessor`], *optional*):
The image processor is a required input.
tokenizer ([`LlamaTokenizerFast`], *optional*):
The tokenizer is a required input.
patch_size (`int`, *optional*):
Patch size from the vision tower.
vision_feature_select_strategy (`str`, *optional*):
The feature selection strategy used to select the vision feature from the vision backbone.
Shoudl be same as in model's config
chat_template (`str`, *optional*): A Jinja template which will be used to convert lists of messages
in a chat into a tokenizable string.
image_token (`str`, *optional*, defaults to `"<image>"`):
Special token used to denote image location.
"""
attributes = ["image_processor", "tokenizer"]
valid_kwargs = ["chat_template", "patch_size", "vision_feature_select_strategy", "image_token"]
image_processor_class = "AutoImageProcessor"
tokenizer_class = "AutoTokenizer"
def __init__(
self,
image_processor=None,
tokenizer=None,
patch_size=None,
vision_feature_select_strategy=None,
chat_template=None,
image_token="<|image_pad|>", # set the default and let users change if they have peculiar special tokens in rare cases
**kwargs,
):
self.patch_size = patch_size
self.vision_feature_select_strategy = vision_feature_select_strategy
self.image_token = image_token
super().__init__(image_processor, tokenizer, chat_template=chat_template)
def __call__(
self,
images: ImageInput = None,
text: Union[TextInput, PreTokenizedInput, List[TextInput], List[PreTokenizedInput]] = None,
audio=None,
videos=None,
**kwargs: Unpack[QllavaProcessorKwargs],
) -> BatchFeature:
"""
Main method to prepare for the model one or several sequences(s) and image(s). This method forwards the `text`
and `kwargs` arguments to LlamaTokenizerFast's [`~LlamaTokenizerFast.__call__`] if `text` is not `None` to encode
the text. To prepare the image(s), this method forwards the `images` and `kwrags` arguments to
CLIPImageProcessor's [`~CLIPImageProcessor.__call__`] if `images` is not `None`. Please refer to the doctsring
of the above two methods for more information.
Args:
images (`PIL.Image.Image`, `np.ndarray`, `torch.Tensor`, `List[PIL.Image.Image]`, `List[np.ndarray]`, `List[torch.Tensor]`):
The image or batch of images to be prepared. Each image can be a PIL image, NumPy array or PyTorch
tensor. Both channels-first and channels-last formats are supported.
text (`str`, `List[str]`, `List[List[str]]`):
The sequence or batch of sequences to be encoded. Each sequence can be a string or a list of strings
(pretokenized string). If the sequences are provided as list of strings (pretokenized), you must set
`is_split_into_words=True` (to lift the ambiguity with a batch of sequences).
return_tensors (`str` or [`~utils.TensorType`], *optional*):
If set, will return tensors of a particular framework. Acceptable values are:
- `'tf'`: Return TensorFlow `tf.constant` objects.
- `'pt'`: Return PyTorch `torch.Tensor` objects.
- `'np'`: Return NumPy `np.ndarray` objects.
- `'jax'`: Return JAX `jnp.ndarray` objects.
Returns:
[`BatchFeature`]: A [`BatchFeature`] with the following fields:
- **input_ids** -- List of token ids to be fed to a model. Returned when `text` is not `None`.
- **attention_mask** -- List of indices specifying which tokens should be attended to by the model (when
`return_attention_mask=True` or if *"attention_mask"* is in `self.model_input_names` and if `text` is not
`None`).
- **pixel_values** -- Pixel values to be fed to a model. Returned when `images` is not `None`.
"""
if images is None and text is None:
raise ValueError("You have to specify at least one of `images` or `text`.")
# check if images and text inputs are reversed for BC
images, text = _validate_images_text_input_order(images, text)
output_kwargs = self._merge_kwargs(
QllavaProcessorKwargs,
tokenizer_init_kwargs=self.tokenizer.init_kwargs,
**kwargs,
)
if images is not None:
image_inputs = self.image_processor(images, **output_kwargs["images_kwargs"])
else:
image_inputs = {}
if isinstance(text, str):
text = [text]
elif not isinstance(text, list) and not isinstance(text[0], str):
raise ValueError("Invalid input text. Please provide a string, or a list of strings")
# try to expand inputs in processing if we have the necessary parts
prompt_strings = text
if image_inputs.get("pixel_values") is not None:
if self.patch_size is not None and self.vision_feature_select_strategy is not None:
# Replace the image token with the expanded image token sequence
pixel_values = image_inputs["pixel_values"]
height, width = get_image_size(to_numpy_array(pixel_values[0]))
num_image_tokens = (height // self.patch_size) * (width // self.patch_size) + 1
if self.vision_feature_select_strategy == "default":
num_image_tokens -= 1
prompt_strings = []
for sample in text:
sample = sample.replace(self.image_token, self.image_token * num_image_tokens)
prompt_strings.append(sample)
else:
logger.warning_once(
"Expanding inputs for image tokens in LLaVa should be done in processing. "
"Please add `patch_size` and `vision_feature_select_strategy` to the model's processing config or set directly "
"with `processor.patch_size = {
{patch_size}}` and processor.vision_feature_select_strategy = {
{vision_feature_select_strategy}}`. "
"Using processors without these attributes in the config is deprecated and will throw an error in v4.47."
)
text_inputs = self.tokenizer(prompt_strings, **output_kwargs["text_kwargs"])
return BatchFeature(data={**text_inputs, **image_inputs})
# Copied from transformers.models.clip.processing_clip.CLIPProcessor.batch_decode with CLIP->Llama
def batch_decode(self, *args, **kwargs):
"""
This method forwards all its arguments to LlamaTokenizerFast's [`~PreTrainedTokenizer.batch_decode`]. Please
refer to the docstring of this method for more information.
"""
return self.tokenizer.batch_decode(*args, **kwargs)
# Copied from transformers.models.clip.processing_clip.CLIPProcessor.decode with CLIP->Llama
def decode(self, *args, **kwargs):
"""
This method forwards all its arguments to LlamaTokenizerFast's [`~PreTrainedTokenizer.decode`]. Please refer to
the docstring of this method for more information.
"""
return self.tokenizer.decode(*args, **kwargs)
@property
# Copied from transformers.models.clip.processing_clip.CLIPProcessor.model_input_names
def model_input_names(self):
tokenizer_input_names = self.tokenizer.model_input_names
image_processor_input_names = self.image_processor.model_input_names
return list(dict.fromkeys(tokenizer_input_names + image_processor_input_names))
7.加载我们的qllava模型
有了模型的实现,现在该考虑如何去利用transformers的方法去加载我们的模型。首先思考一下我们是怎么使用hugging face上的一些模型的:
-
1.在hf上找到我们需要使用的模型的仓库,例如llava-hf,仓库内通常除了有模型权重外,还有模型的config、tokenizer的config等等加载模型需要的信息
-
2.使用AutoXXXModel的from_config或from_pretrain的方法,先通过model、tokenizer、image processor的config来初始化对应的类,然后再load模型的权重
因此,我们也需要保存一份模型和tokenizer等组件的config到本地,方便使用from_config或pretrain的方法加载。其次,由于qllava是我们自己创建的模型,不像qwen2-vl或llava-hf这些已经在transformers库中的模型能被AutoXXXModel的方式识别,我们需要将qllava和相关组件进行注册,让transformers库记住我们的模型。
在模型的同级目录下新建一个config.py,用来实现上述的两个需求:
from configuration_qllava import QllavaConfig
from processing_qllava import QllavaProcessor
from modeling_qllava import QllavaForConditionalGeneration
from transformers import AutoModelForCausalLM, AutoModelForVision2Seq, CLIPVisionConfig, CLIPVisionModel, AutoTokenizer, AutoImageProcessor, CLIPImageProcessor, AutoConfig
from transformers import AutoProcessor, Qwen2TokenizerFast, LlavaProcessor
vision = "clip-vit-large-patch14-336" ### 换成hugging face或本地的路径
text = "Qwen2-7B" ### 换成hugging face或本地的路径
tokenizer_id = "Qwen2-VL-7B-Instruct" ### 换成hugging face或本地的路径
cfg = dict(
vision_model=vision,
text_model=text,
ignore_index=-100,
image_token_index=151655,
projector_hidden_act="gelu",
vision_feature_select_strategy="default",
vision_feature_layer=-2,
image_seq_length=576,
train_from_scratch=False
)
def check_file_exists(directory, filename):
import os
file_path = os.path.join(directory, filename)
return os.path.isfile(file_path)
def prepare_qllava(save_path, prepared_modules=["model", "tokenizer", "processor", "image_processor"]):
from llamafactory.data.template import _register_template, StringFormatter, EmptyFormatter, get_mm_plugin
existsed = False
if check_file_exists(save_path, "config.json"):
existsed = True
if existsed:
qllava_config = QllavaConfig.from_pretrained(save_path)
else:
qllava_config = QllavaConfig(**cfg)
model = QllavaForConditionalGeneration._from_config(qllava_config)
tokenizer = AutoTokenizer.from_pretrained(tokenizer_id)
image_process = CLIPImageProcessor.from_pretrained(vision)
process = QllavaProcessor(image_processor=image_process, tokenizer=tokenizer)
if not existsed:
qllava_config.train_from_scratch = False
model = QllavaForConditionalGeneration._from_config(qllava_config)
qllava_config = model.config
qllava_config.architectures = [QllavaForConditionalGeneration.__name__]
qllava_config.pad_token_id = tokenizer.pad_token_id if tokenizer.pad_token_id is not None else tokenizer.eos_token_id
qllava_config.padding_side = tokenizer.padding_side
if not existsed:
qllava_config.save_pretrained(save_path)
tokenizer.save_pretrained(save_path)
image_process.save_pretrained(save_path)
process.save_pretrained(save_path)
# register into hugginface
AutoConfig.register(qllava_config.model_type, QllavaConfig)
AutoModelForVision2Seq.register(QllavaConfig, QllavaForConditionalGeneration)
AutoProcessor.register(QllavaConfig, processor_class=QllavaProcessor)
# 适配Llama-Factory
_register_template(
#### same as qwen2-vl ####
name="qllava",
format_user=StringFormatter(slots=["<|im_start|>user\n{
{content}}<|im_end|>\n<|im_start|>assistant\n"]),
format_system=StringFormatter(slots=["<|im_start|>system\n{
{content}}<|im_end|>\n"]),
format_observation=StringFormatter(slots=["<|im_start|>tool\n{
{content}}<|im_end|>\n<|im_start|>assistant\n"]),
format_separator=EmptyFormatter(slots=["\n"]),
default_system="You are a helpful assistant.",
stop_words=["<|im_end|>"],
replace_eos=True,
replace_jinja_template=False,
### use llava plugin and qwen2 image token###
mm_plugin=get_mm_plugin(name="llava", image_token="<|image_pad|>", video_token="<|video_pad|>"),
)
首先检查本地路径下是否有config.json文件,如果没有后续会保存在本地,如果有的话则只注册进transformers库当中。最后的_register_template则是为了适配Llama-Factory库,用来注册一个“qllava”的template,这个template使用的模版和qwen2的相同,多模态插件mm_plugin则使用了Llama-Factory中自带的llava 插件,但是更改了image_token(和qwen2的相同)。
现在,只需要在加载模型前运行prepare_qllava函数,即可把qllava当作hugging face上已有的模型一样正常使用,也可以用来进行推理。
8.使用Llama-Factory对qllava进行训练
众所周知,这是一个对LLM进行各种阶段训练的库,实际上它也能对VLM模型进行训练(而且是为数不多支持qwen2-vl微调的框架,这让我放弃了学习xtuner 。)
由于我对Llama-Factory做了太多魔改,如果直接放代码出来可能会不兼容,这里介绍一下步骤和需要注意的点,大致上和训练一个正常的模型也差不多
-
1.在正式进入训练前记得使用prepare_qllava,保证加载模型时能够识别qllava,以及llama-factory能找到qllava的template,可以加在sft的workfolw的最前面或其他地方
-
2.从头开始训练时,记得修改qllava config中的train_from_scratch(默认为false)
-
3.修改LLaMA-Factory/src/llamafactory/model/model_utils/visual.py中get_forbidden_modules函数,有一行:
if model_type in ["llava", "llava_next", "llava_next_video", "paligemma", "video_llava"]
这里model_type固定了只可能是这几类,把我们的qllava加进去即可,其他地方也有一些类似的情况,但大部分都对训练不影响。
9.pretrain阶段的配置
和正常训练llava基本相同,需要修改的点有:
-
1.model_name_or_path设置为我们prepare_qllava函数传入的路径,在这个路径下我们保存了qllava各个组件的config
-
2.train_mm_proj_only设置为true,因为在第一阶段只训练proj层
-
3.stage设置为sft,VLM的pretrain和LLM的pretrain是不相同的,在VLM中不管第一阶段pretrain还是第二阶段sft,在llama-factory中都对应stage为sft
-
4.template设置为qllava,我们在prepare_qllava中对qllava对应的template完成了注册
本项目训练使用的一些超参数:
-
1.cutoff_len:2048, 对齐llava
-
2.单卡batch 32,gradient_accumulation_steps为1,一共8卡,总batch为 3218 = 256,对齐llava
-
3.lr:2.0e-3,对齐llava
-
4.warmup_ratio: 0.03, 对齐llava
-
5.lr_scheduler_type: cosine, 对齐llava
-
6.epoch:1, 对齐llava
sft阶段的配置:
和pretrain阶段基本相同,需要修改的有:
-
1.model_name_or_path设置为我们预训练得到的权重的路径
-
2.freeze_vision_tower: true,第二阶段同时训练llm和proj,由于llm需要训练,显存不够这里可以用lora
-
3.stage: sft,这里stage仍是sft
本项目使用的超参数:
-
1.cutoff_len:2048, 对齐llava
-
2.单卡batch 8,gradient_accumulation_steps为2,一共8卡,总batch为 288 = 128,对齐llava
-
3.lr:2.0e-5,对齐llava
-
4.warmup_ratio: 0.03, 对齐llava
-
5.lr_scheduler_type: cosine, 对齐llava
-
6.epoch:1, 对齐llava
10.使用qllava进行推理
如前文所言,只需要在使用模型前运行prepare_qllava函数即可,值得注意的是,qllava的generate_config中没有保存eos_token_id,这里应该在哪解决暂时还没找到,但是也没有太大影响,只需在generate时添加eos_token_id=tokenizer.eos_token_id即可,不然模型会一直输出到最大的长度。
import torch
from PIL import Image
from transformers import AutoProcessor, AutoModelForVision2Seq, AutoTokenizer, AutoImageProcessor
from XXX import prepare_qllava ### 这里根据自己的路径导入prepare_qllava
model_id = "PATH" ### 这里换成自己保存的模型的路径
prepare_qllava(model_id)
IMAGE_TOKEN = "<|image_pad|>"
#STOP_WORDS = "<|im_end|>"
template = f"<|im_start|>user\n{
{content}}<|im_end|>\n<|im_start|>assistant\n"
model = AutoModelForVision2Seq.from_pretrained(model_id, device_map="cuda:0")
tokenizer = AutoTokenizer.from_pretrained(model_id)
#print(tokenizer.eos_token)
image_processor = AutoImageProcessor.from_pretrained(model_id)
processor = AutoProcessor.from_pretrained(model_id)
prompt_1 = template.format(content=f"{IMAGE_TOKEN}\nWhat does this image show?")
prompt_2 = template.format(content=f"{IMAGE_TOKEN} {IMAGE_TOKEN} \nWhat is the difference between these two images?")
image_file_1 = "1.jpg"
image_file_2 = "2.jpg"
raw_image_1 = Image.open(image_file_1)
raw_image_2 = Image.open(image_file_2)
inputs = processor([prompt_1, prompt_2], [raw_image_1, raw_image_1, raw_image_2], padding=True, return_tensors="pt").to(0, torch.float16)
output = model.generate(**inputs, max_new_tokens=200, do_sample=False, eos_token_id=tokenizer.eos_token_id)
print(processor.batch_decode(output, skip_special_tokens=True))
11.总结
本项目从0开始训练了一个MMMU分数超过llava-1.5的qllava模型,和llava相比差别在于LLM变成了qwen2。虽然xtuner和一些其他的框架可以很轻易的实现更换VLM中的某个组件后训练,但是我个人更喜欢Llama-factory的整体架构和社区的活跃度,并且自己从0开始构建模型相比更改config来替换结构更能加深对模型的理解,以及对transformers库的理解吧,本项目同时也可以看作 “如何在transformers库中添加自己的模型”以及“如何在Llama-Factory中添加自己的模型”的教程。