Author/ Simba
Senior IBM Business Analyst.
IT veteran.
Lifelong learner.
Welcome back, this article starts with a basic concept-retention. "Retention" during the interview is also a question that is likely to be asked. If you want to confirm your answer, let's discuss it together. This article is about 4000 words, and it takes 10 minutes to finish it.
01 Retained how to count
"Isn't retention just about the user's usage in the next xx days? For example, the first day is 1000, 100 people come the next day, and the retention rate is 10%." There is no problem with the algorithm itself, just like the example in the previous article. :
There is an event that encourages trial use lasting for 2 days, let’s look at the calculation of the 3-day retention rate.
On October 1, 1,000 people tried it out; on the first day, 300 of these 1,000 people logged into the CRM software; the second day, 200 people logged into the CRM software; on the third day, 150 people logged into the CRM system.
On October 2, 1500 people tried it out; 400 people logged in to the CRM software on the first day; 200 people logged in to the CRM software on the second day; 150 people logged in to the CRM system on the third day.
Then for the events on October 1 and October 2, the retention rates for the three days are as follows (shown in blue):

But to reflect the 3-day retention rate of the overall activity, how should it be calculated? My method is to divide the total number of people retained on the Xth day of the activities in the past few days by the total number of people who have been refreshed in the past few days, namely:
The overall retention rate for the first day is:
The sum of the total number of people retained on the first day/the total number of people who have been updated in the past few days
The overall retention rate for the second day is:
The sum of the total number of people retained on the second day/the total number of people who have been updated in the past few days
……
And so on, as shown in the green content in the following figure:

This is also the method I mentioned above, but a small partner put forward his opinion (if this small partner sees this article, you can contact me~).

So I put the specific calculation method in a larger group to discuss, different voices appeared, that situation is like a group of north and south friends gathering at my house, I prepared to put sugar in scrambled eggs with tomatoes, and was seen by a northerner. Later, they questioned loudly: "Don't put sugar in tomatoes and eggs", and then another group of southerners answered immediately, "Ah, of course you must put sugar in".
“It’s better to calculate the average value of retention in the same period, not the average of the sum, but the average of the percentage.” So the other method here is to add up the retention percentages of the two days and divide by the total number of days. which is:
The overall retention rate for the first day is:
(Day1 retention rate on October 1 + day1 retention rate on October 2)/2
The overall retention rate for the second day is:
(Day2 retention rate on October 1 + day3 retention rate on October 2)/2
……
And so on (shown in orange in the figure below):

It seems that the results of the two algorithms are similar, but there is another voice:
"Under normal circumstances, there is not much difference between the values obtained by the two algorithms, but if the number of new updates in the two days is very different, and the retention rate is also very different, there will be inconsistencies, such as the following example, according to the first One method is calculated to be 2.75%, and the second method is calculated to be 46%."

Wow, how do you count that. "The second kind is calculated by weighted average, and the weight can be calculated by the number of new recruits." So the third algorithm was derived, but a closer look shows that the weighted calculation according to the number of new recruits is actually the same as the first algorithm. (Shown in light green in the third row of the figure below).

At this time, another voice shifted the front of the conversation to a new question: "Let’s not discuss right or wrong. Have you ever thought about the difference between the two algorithms in business applications? Ignoring the scenario, it is for most operations. People will definitely look at this number first; because there is a 98% loss in the first piece of data, there are too many things to reflect on, and the latter will be easier to implement the strategy and have direction and motivation."
So in order to reflect this kind of anomaly, we need global and detailed retention information, so we return to the original stepped retention form, and use the heat map to highlight the outliers (such as the picture below), so that the business staff can see the abnormality at a glance. Analysis, and these outliers often contain opportunities or risks, or bugs.
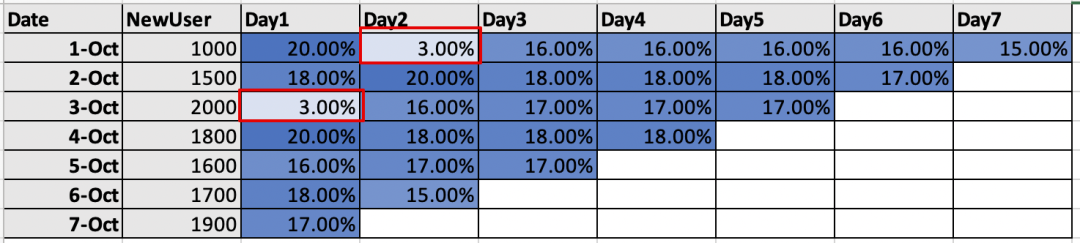
However, if we want to more accurately reflect the three-day retention rate of the overall activity, we need to eliminate these outliers to calculate the retention rate. There are many ways to eliminate them (you can refer to the various algorithms of the Python library Pyod, in this There are various detailed algorithms in the PDF shared by Kaggler: https://www.kaggle.com/getting-started/104950). In the case of eliminating abnormal data, no matter whether the first algorithm or the second algorithm is used, the difference is not big, and the value to the business is almost the same, as shown in the figure below. Well, the problem can be over a little bit now.

However, the discussion on retention does not end here. After understanding retention calculations, as an excellent data analyst, you must know how to calculate retained data and how to use retained data.
02 Retained how to use
After we figure out the retention calculation, let's ask, why should we look at retention? Don't think this is the product manager's business. Data analysts can only provide accurate and reliable data if they understand why. One of the uses of retention is to estimate company earnings based on retention rates and other factors (this is also a problem that data analysts often encounter during interviews), but the use of retention data can go far beyond that.
The author once saw a sentence like "Without retention, your product is a leaky bucket" in an article, which translates as "No retention, it’s like a bamboo basket catching water and an empty bucket" (or a more vivid translation, "Without retention, your product is a leaky bucket" The promotion of "is a bottomless pit"), and finally attracted new customers, all of them flowed out again. In the second half of the Internet wave, another growth model has already surfaced, that is, the AARRR model is transformed into RARRA. As shown in the figure below, in the RARRA model, retention--Retention bears the brunt of it, that is, retention is realized first. , And then do product promotion, let the product operate by itself, and achieve customer acquisition.
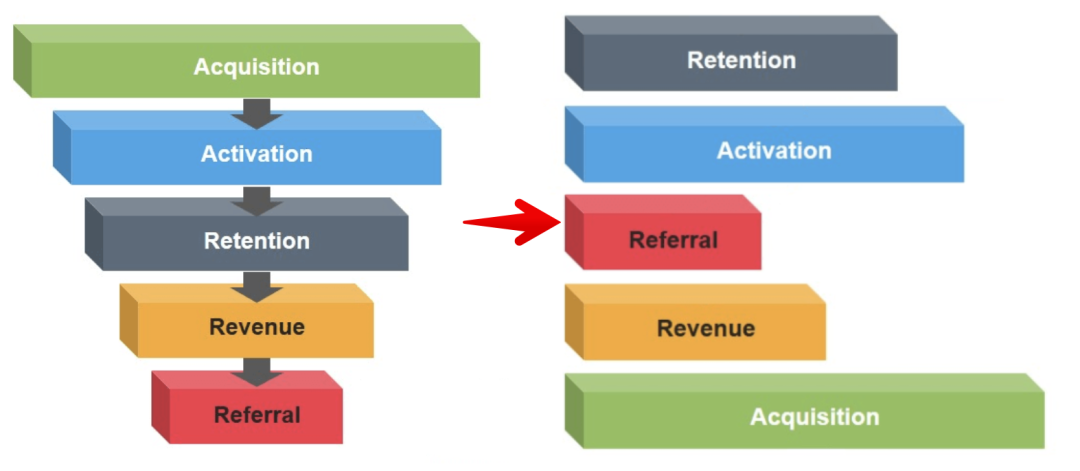
I believe many people have read Zhang Xiaolong’s [WeChat Ten Years]. The speech mentioned: "This is a typical WeChat-style product method, that is, through products rather than operations, to find the point of leveraging things, through "Product capabilities make things work" is highly consistent with RARRA's philosophy. Let’s take a look at the hot spots today and use the remaining framework to "routine" the growth process of WeChat video accounts. It is purely a discussion from the perspective of learning. Welcome everyone to leave a message.
I personally think that under the RARRA growth model, there are the following stages in the product growth process, and retention is one of the indicators that need to be paid attention to in these stages:
Find Market Fit, this stage of retention analysis can help the product discover "survivability".
Cultivate the initial usage habits of retained users and continue to optimize core functions. At this stage, retention analysis can help the product discover "basic capabilities".
Let more people see the core functions and make the product be used by a wider range of people. At this stage, retention analysis can help the product discover "value capabilities."
Let's expand on it in detail:
Phase 1: Find Market Fit (ie, the fit point between the product and the market). At this stage, the retention analysis can help the product discover the "survivability".
The process of finding Market Fit by WeChat video account is like this, "The following is an excerpt from the original text of Zhang Xiaolong's "WeChat Ten Years"".
"Maybe in 2017... but then it stopped...
The first version actually just built such an ID system, but the effect is not good...
But scrolling in the first few months was particularly difficult, and seemed to be stuck in a deadlock...
(2020) In May, we made the most significant change in the video account... So in May we released a new gray version based on friends’ likes, and finally saw the rising data, and the retention of users was very high. . "
I don’t know how you feel when you see this process. I’m thinking that, as the industry’s “top product manager”, who has gone through so many twists and turns to try new things, what reason do we have not to take risks and try quickly? Wrong?
So what is the "upward data" mentioned by Long Ge? What kind of retention curve is the Market Fit found? As shown in the figure below, the green represents the retention curve of the Market Fit not found, and the blue represents the curve of the Market Fit found, showing a "rising" trend. Of course, this process is not as simple as this curve, just for learning, I simplified this curve.
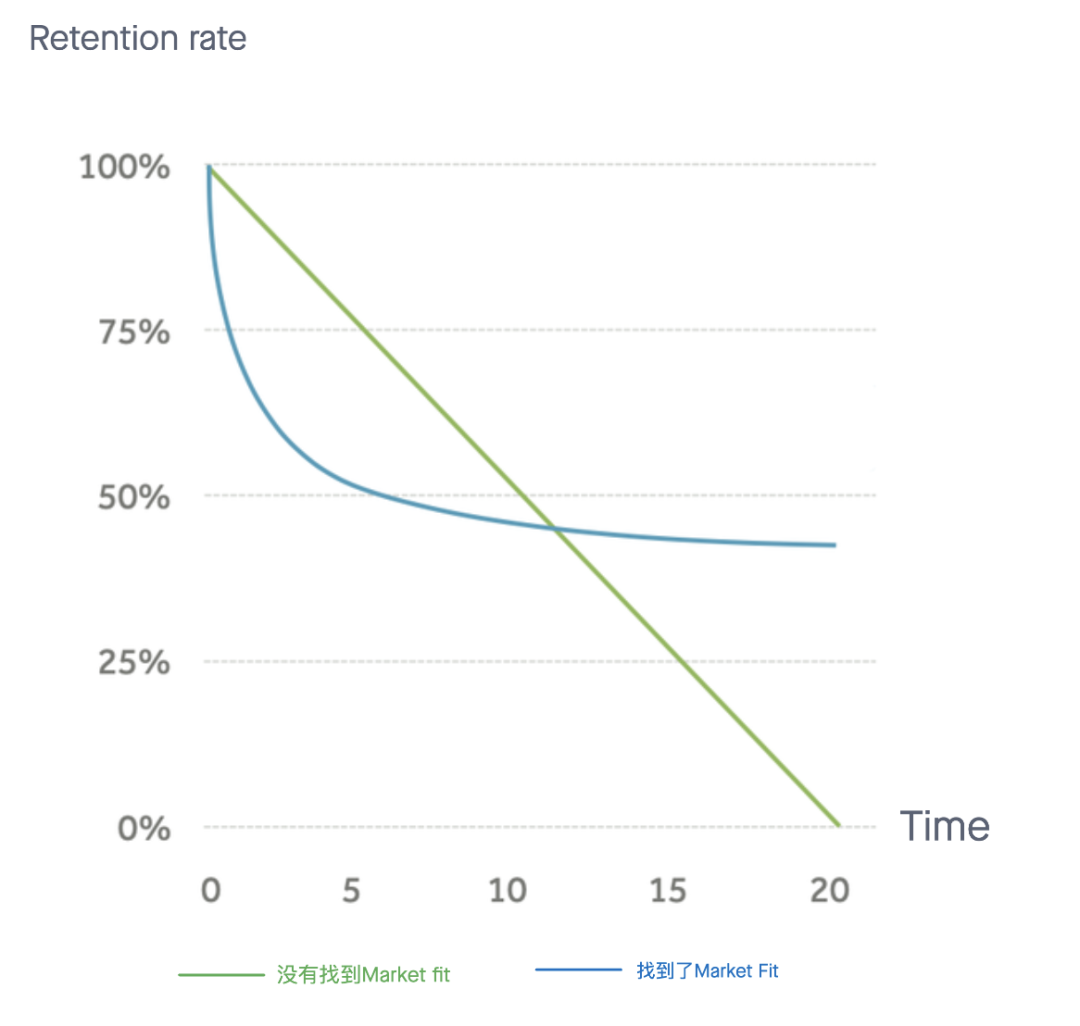
Phase 2: Cultivate the initial usage habits of retained users and continue to optimize core functions. At this stage, retention analysis can help the product discover "basic capabilities".
"The following is still taken from the original text of Zhang Xiaolong's "WeChat Ten Years"."
"So the users of the June video account have reached an order of magnitude. The number is actually not important, but for a content-based product, a certain order of users means that the problem of life and death has been solved, that is, the circulation of traffic has started... Yes This user base shows that you have survived, and you can start to improve basic functions, such as live broadcast capabilities. If there is no life and death line, no matter how many functions are used, it is no use."
Noted whether: "A magnitude...number is actually not important.. To complete the basic functions" means that after the survival problem is solved in Phase 1, the growth of numbers is not focused on, but starts to do "basic functions." Perfection". That is to say, in the second stage, it is to continuously stimulate these users to continue to use, stick to them, and obtain long-term retention, so the simplified retention curve you see may look like this:
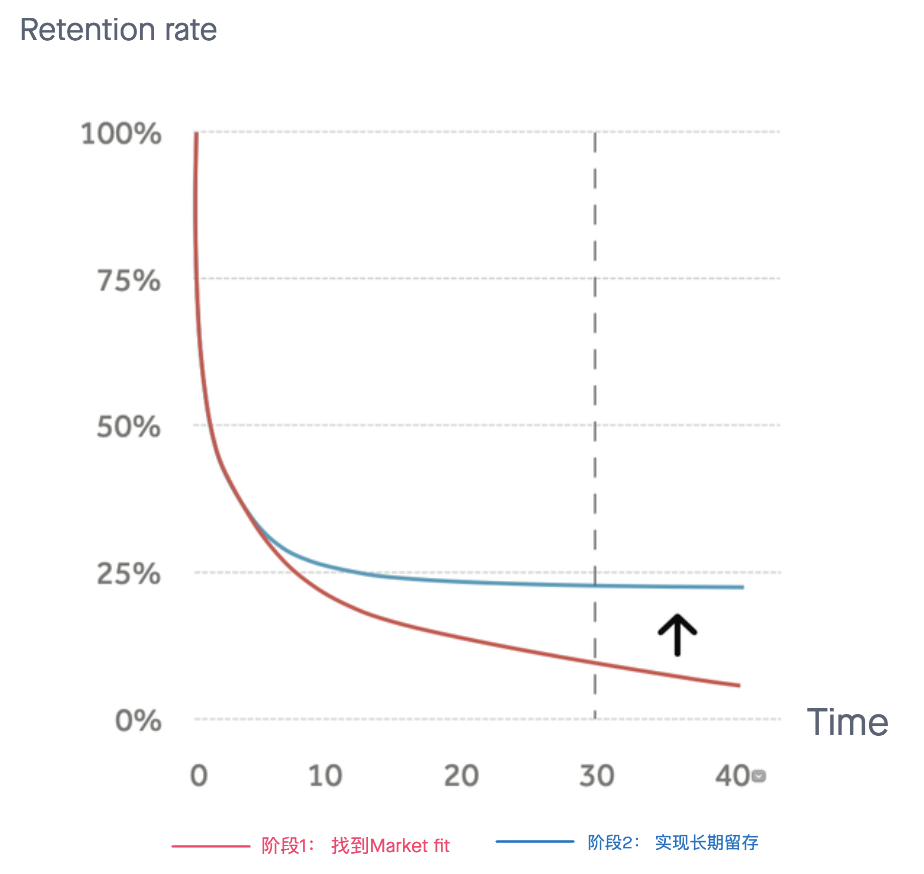
Stage 3: Let more people see the core functions, so that the product can be used by a wider range of people. At this stage, retention analysis can help the product discover "value capabilities".
Let me talk about my observations on WeChat video accounts. At present, among the people around me, the proportion of users of WeChat video accounts is not too high. Most people still think that video accounts are time-killing machines like Douyin (currently Indeed). Forgive me that the people around me are more diligent, not "watching the crowd", and unwilling to spend time on recreational social activities. But at the same time, I also see that more and more executives, elites, and those who used to write official accounts have started to make WeChat video accounts. The trend is good. Therefore, I personally feel that the WeChat video account is still at this stage 3. As for whether the WeChat video account can go to stage 3 well, it remains to be seen in the future.
So, at this stage of the product, how to use retention analysis to help the product discover "value capabilities"? We can roughly divide users into new users, existing users, and lost users for analysis.
New user retention analysis: find an event that allows new users to come back again (mined from stage 2), and use this event to improve the first impression of the product. From the retention curve, the change in the retention curve of new users is more inclined to down Picture this. Of course, in order to exaggerate the effect of the first impression, we simplified this retention curve a lot.
Retention analysis of existing users: Some people may say that if existing users mean that they have been retained, there is no need to do retention analysis. On the contrary, the retention analysis of this part of users best reflects the core value of the product, that is, using the traces of existing users' behavior to help us understand "what value the product can bring to users."
Take video content products as an example. Users may want to use videos for brand promotion, may be to find their own circle to learn professional domain knowledge, or it may be for fun, and there may be some value that we don't see. According to the behavior, we roughly divide the users of video content products into two categories (in fact, it can be subdivided), creators and viewers. We need to define different retention standards for two types of roles:
1. The definition of creator retention may look like this:
The initial act of retention is to publish the video
Keeping the follow-up action is to post the video
Time frequency is per week, or other customized days
That is, users who have posted another video within a week (or other customized days) since the last time the video was posted are reserved for the creator.
2. The definition of retention for viewers can look like this:
The initial behavior of retention is to watch the video
Keeping the follow-up action is to watch the video
Time frequency is every day, or other customized days
That is, users who have watched the video again within 1 day (or other customized days) since the last time they watched the video are retained for the viewers.
The simplified retention curve you see might look like this:
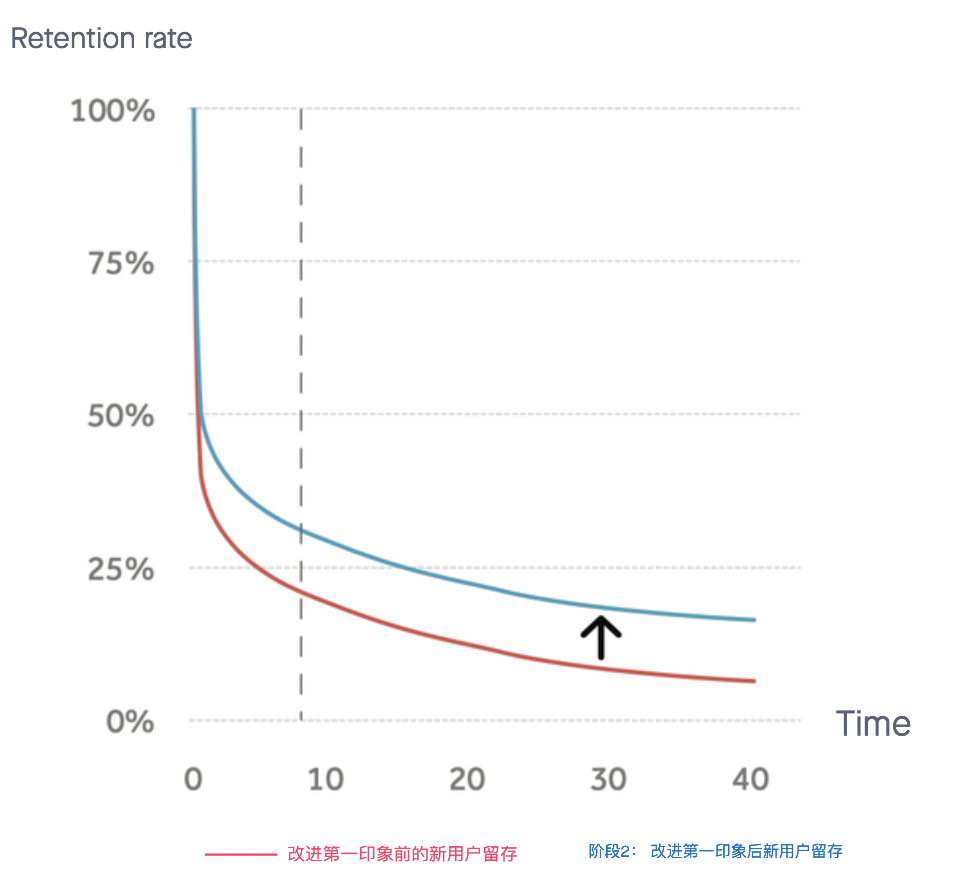
Through the retention analysis of different behavior groups is a judgment on the value of product segmentation, we can help the product build a healthy growth engine.
Analysis of retention of lost users:
Why do lost customers still need to be retained?
Because we need to bring old customers back.
Studies have shown that reactivating old customers is actually cheaper than acquiring new customers.
These users may be the users you acquired in the first stage, because the product was of little value to them at that time, but in the third stage, it may be a good time to win them back:
Appropriately organize old customers to pull back activities and tell them whether the recent product changes (rather than harassment push) will be more effective.
When doing such activities, we also need to observe the retention rate of customers re-returned to judge whether the product changes have the ability to bring back "lost users".
Extend the time, this part of the retention curve may even show a "smiling curve."
Of course, the application of the above-mentioned retention analysis at different stages of the product is not a generalization. It can only be said that at different stages, our retention analysis has a different focus. One thing that remains unchanged is that "retention analysis" plays a very important role in the entire life cycle of a product, especially in an era when Internet products are flourishing, retention is particularly important.
03 summary
Retention is an important evaluation index for current Internet products, and it is also the first element of the growth *** model under the current situation. This article discusses retention analysis from two perspectives:
How to calculate retention, we need to provide an accurate retention rate analysis report, which is more of the responsibility of data analysts.
How to use retention and how to use retention analysis to promote product iteration and achieve product growth is more of the responsibility of product managers, but as a data analyst, understanding the use of data is equally important.
Of course, this article is an introduction. Data analysts, product managers, and data product managers are welcome to discuss together, and feel free to correct any errors. See you next time.
The private place of a data person is a big family that helps the data person grow, helping partners who are interested in data to clarify the learning direction and accurately improve their skills. Follow me and take you to explore the magical mysteries of data
1. Go back to "Data Products" and get <Interview Questions for Data Products from Big Factory>
2. Go back to "Data Center" and get <Dachang Data Center Information>
3. Go back to "Business Analysis" and get <Dachang Business Analysis Interview Questions>;
4. Go back to "make friends", join the exchange group, and get to know more data partners.