Chapter 1 Basic Image Operation and Processing
1.1 PIL: Python image processing library
PIL provides general image processing functions, as well as basic image operations, such as image scaling, cropping, rotation, color conversion, etc. Using functions in PIL, data can be read from image format files and then written to image format files.
Image color conversion: convert()
from PIL import Image
from matplotlib import pyplot as plt
plt.figure(figsize=(8,6))
color_img = Image.open('picture1.jpg')
plt.subplot(121)
plt.imshow(color_img,cmap='gray')
# 不显示坐标轴
plt.axis('off')
# 转换成灰度图像
gray_img = color_img.convert('L')
plt.subplot(122)
plt.imshow(gray_img,cmap='gray')
plt.axis('off')
plt.show()
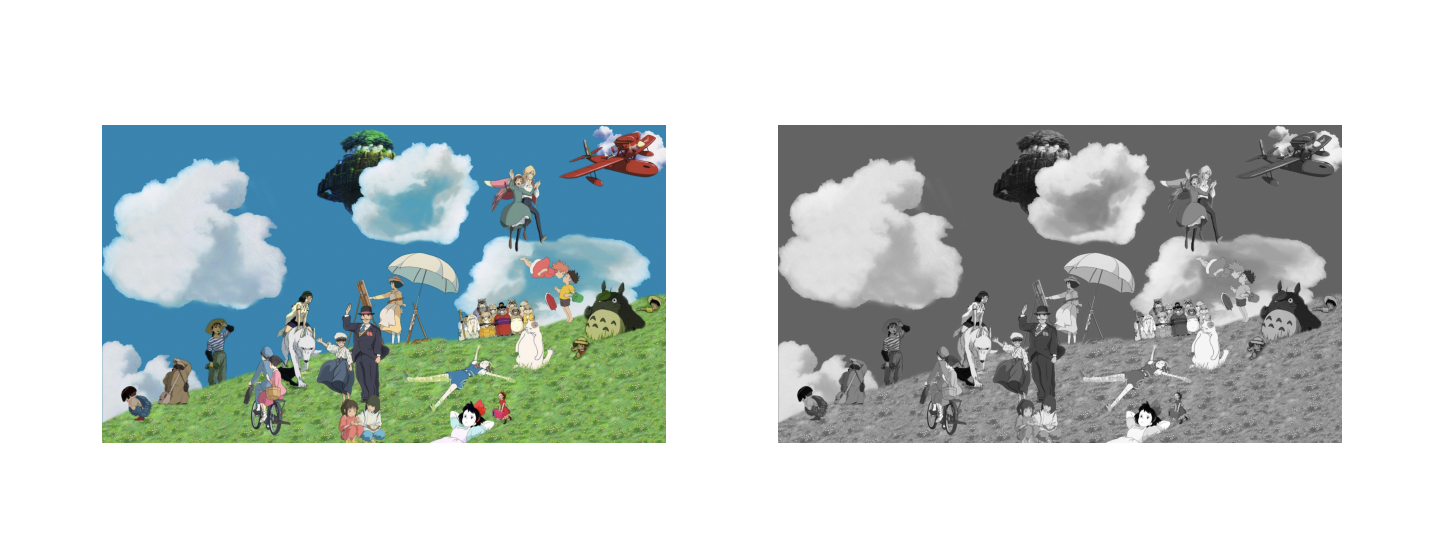
As you can see from the output, the color image can be converted to a grayscale image by the convert('L') method.
1.1.1 Convert image format
from PIL import Image
import os
# 想要更改图片所在的根目录
rootdir = "/Users/xionglulu/Desktop/picture"
# 获取目录下文件名清单
files = os.listdir(rootdir)
# 对文件名清单里的每一个文件名进行处理
for filename in files:
portion = os.path.splitext(filename) # portion为名称和后缀分离后的列表 #os.path.splitext()将文件名和扩展名分开
if portion[1] == ".png": # 如果为后缀为.png则更改名字
newname = portion[0] + ".jpg" # 要改的新后缀 #改好的新名字
print(newname) # 打印出更改后的文件名
os.chdir(rootdir) # 修改工作路径
os.rename(filename, newname) # 在工作路径下对文件名重新命名
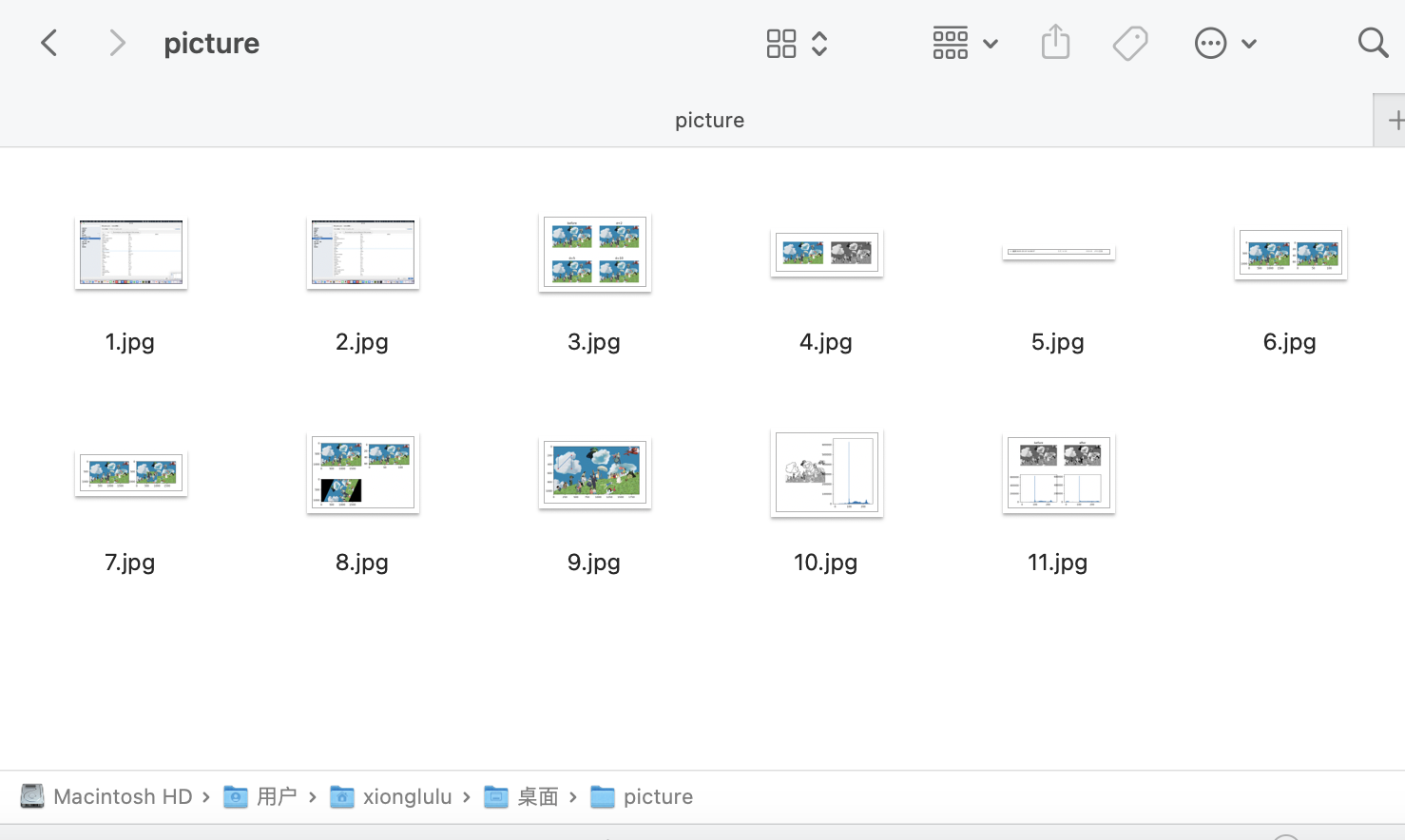
The method of realizing batch conversion of image format: 1. Obtain a list of all file names under the root directory where the image is located 2. Segment the file name 3. Convert the file name format 4. Update the path and rename the image.
In this experiment, splitting the file name is the key. Use the os.path.splitext(filename) method to split the file name into name and suffix. name.
1.1.2 Thumbnailing, copy-pasting image areas, resizing and rotation of images
Image thumbnail: thumbnail()
This method can convert the image into a thumbnail with the specified parameter size.
from PIL import Image
from matplotlib import pyplot as plt
# plt.figure(figsize=(8,6))
color_img= Image.open('picture1.jpg')
plt.subplot(121)
plt.imshow(color_img,cmap='gray')
#创建缩略图
color_img.thumbnail((128,128))
plt.subplot(122)
plt.imshow(color_img,cmap='gray')
plt.show()
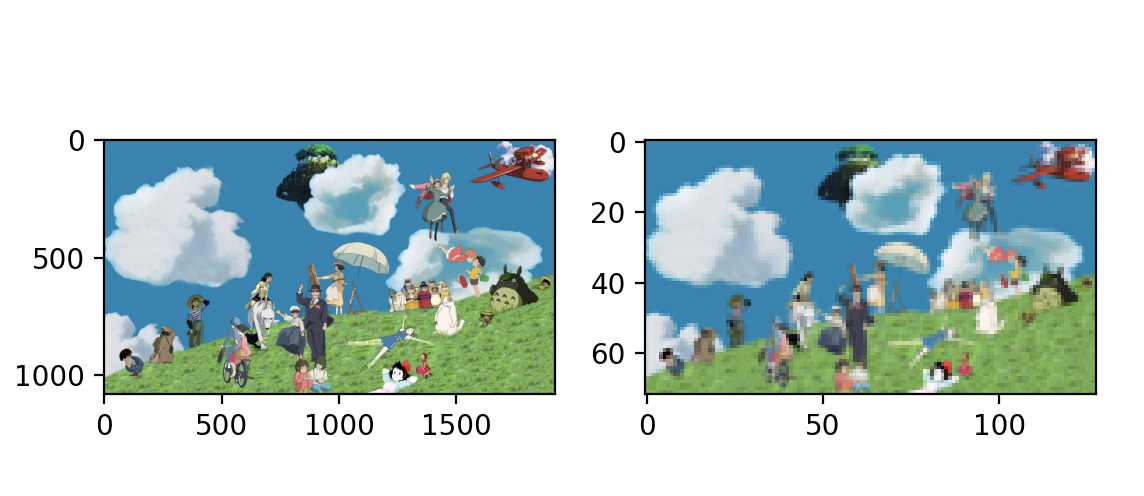
As you can see from the output, the original image is converted to a thumbnail with a longest side of 128 pixels.
Copy and paste image regions: crop(), paste()
Use the crop() method to crop a specified area from an image, which is specified by a quadruple. The coordinates of the quadruple are (left, top, right, bottom) in order. The cropped region can be put back using the paste() method.
from PIL import Image
from matplotlib import pyplot as plt
# plt.figure(figsize=(8,6))
color_img= Image.open('picture1.jpg')
plt.subplot(121)
plt.imshow(color_img,cmap='gray')
box=(500,500,1000,1000)
region=color_img.crop(box)
region=region.transpose(Image.ROTATE_180)
color_img.paste(region,box)
plt.subplot(122)
plt.imshow(color_img,cmap='gray')
plt.show()
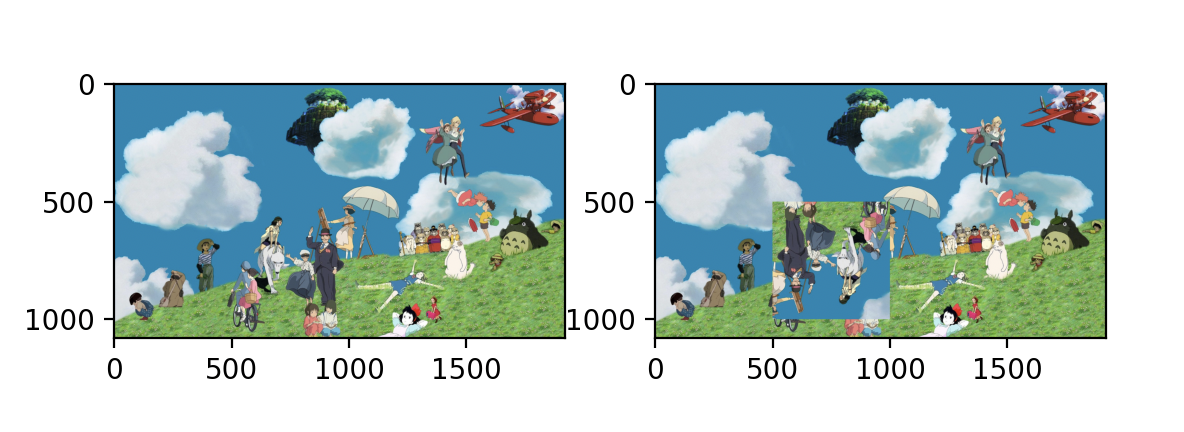
As can be seen from the result comparison chart, after cropping the specified area, it is rotated 180 degrees and put back. The quadruple is (500, 500, 1000, 1000).
Resize and rotate: resize(), rotate()
Use the resize() method to adjust the original image to the specified parameter size, and use the rotate() method to rotate the original image counterclockwise by the specified angle.
from PIL import Image
from matplotlib import pyplot as plt
# plt.figure(figsize=(8,6))
color_img= Image.open('picture1.jpg')
plt.subplot(221)
plt.imshow(color_img,cmap='gray')
#调整尺寸
p1=color_img.resize((128,64))
plt.subplot(222)
plt.imshow(p1,cmap='gray')
#旋转图像
p2=color_img.rotate(65)
plt.subplot(223)
plt.imshow(p2,cmap='gray')
plt.show()
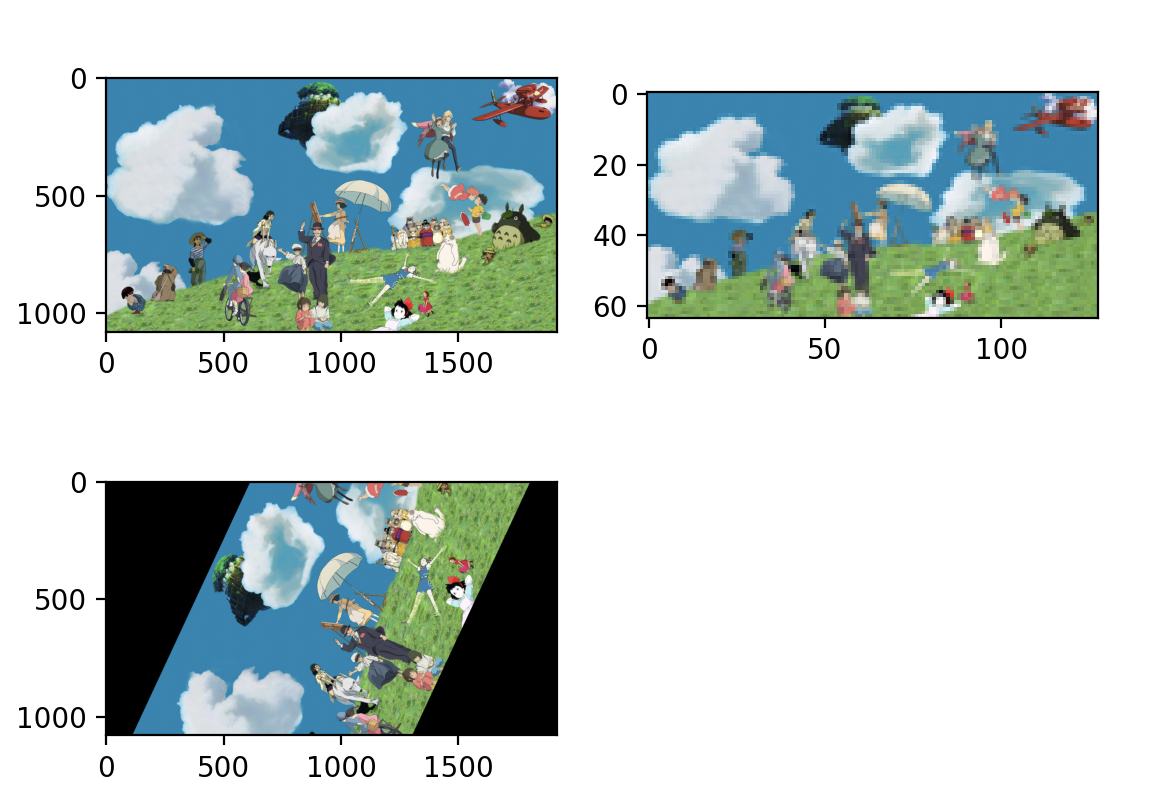
From the output results, it can be seen that the size of the original image becomes smaller, and the original image is rotated 65 degrees counterclockwise.
1.2 Matplotlib
Matplotlib can be used to process mathematical operations, draw charts, and draw points, lines, and curves on images. It is a class library with more powerful drawing functions than PIL. The Pylab interface to Matplotlib contains many functions for creating plots.
1.2.1 绘制图像、点和线
from PIL import Image
from pylab import *
#读取图像到数组中
color_im=array(Image.open('picture1.jpg'))
#绘制图像
plt.imshow(color_im)
#一些点
x=[100,100,400,400]
y=[200,500,200,500]
#使用红色星状标记绘制点
plot(x,y,'r*')
#绘制连接这些点的线
plot(x[:4],y[:4])
plt.show()
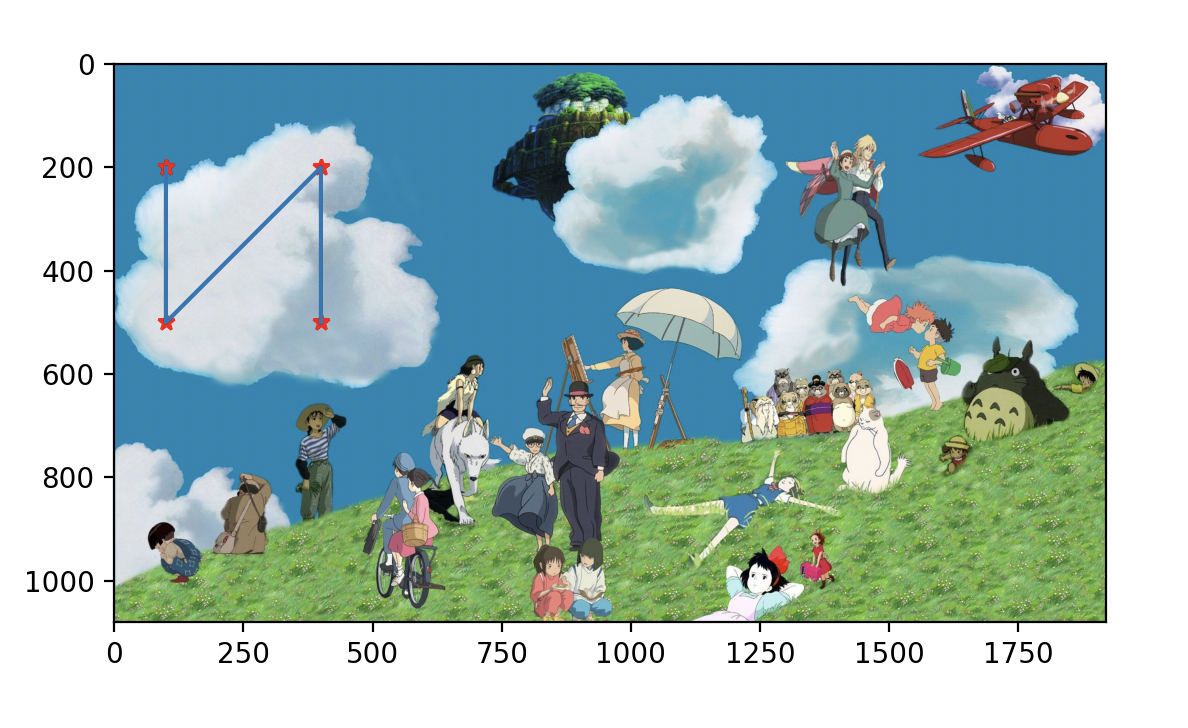
通过结果图可以看出,代码首先绘制出原图像,然后在x和y列表中给定点的x坐标和y坐标上绘制出红色星状标记点,最后在两个列表表示的四个点之间绘制一条线。
1.2.2 图像轮廓和直方图
因为绘制图像的轮廓图需要对每个坐标[x,y]的像素值施加同一个阈值,所以需要首先将图像灰度化。
图像的直方图用来表征该图像像素值的分布情况。用一定数目的小区间来指定表征像素值的范围,每个小区间会得到落入该小区间表示范围的像素数目。
灰度图像的直方图用hist()函数绘制:hist()函数的第二个参数指定小区间的数目。
注意:因为hist()只接受一维数组作为输入,所以在绘制图像直方图之前,必须先对图像进行压平处理。flatten()方法将任意数组按照行优先准则转换为一维数组。
from PIL import Image
from pylab import *
#读取图像到数组中
gray_im=array(Image.open('picture1.jpg').convert('L'))
#新建一个图像
figure()
#不使用颜色信息
gray()
#显示轮廓图像
subplot(121)
contour(gray_im,origin='image')
axis('equal')
axis('off')
#绘制图像直方图
subplot(122)
hist(gray_im.flatten(),128)
show()
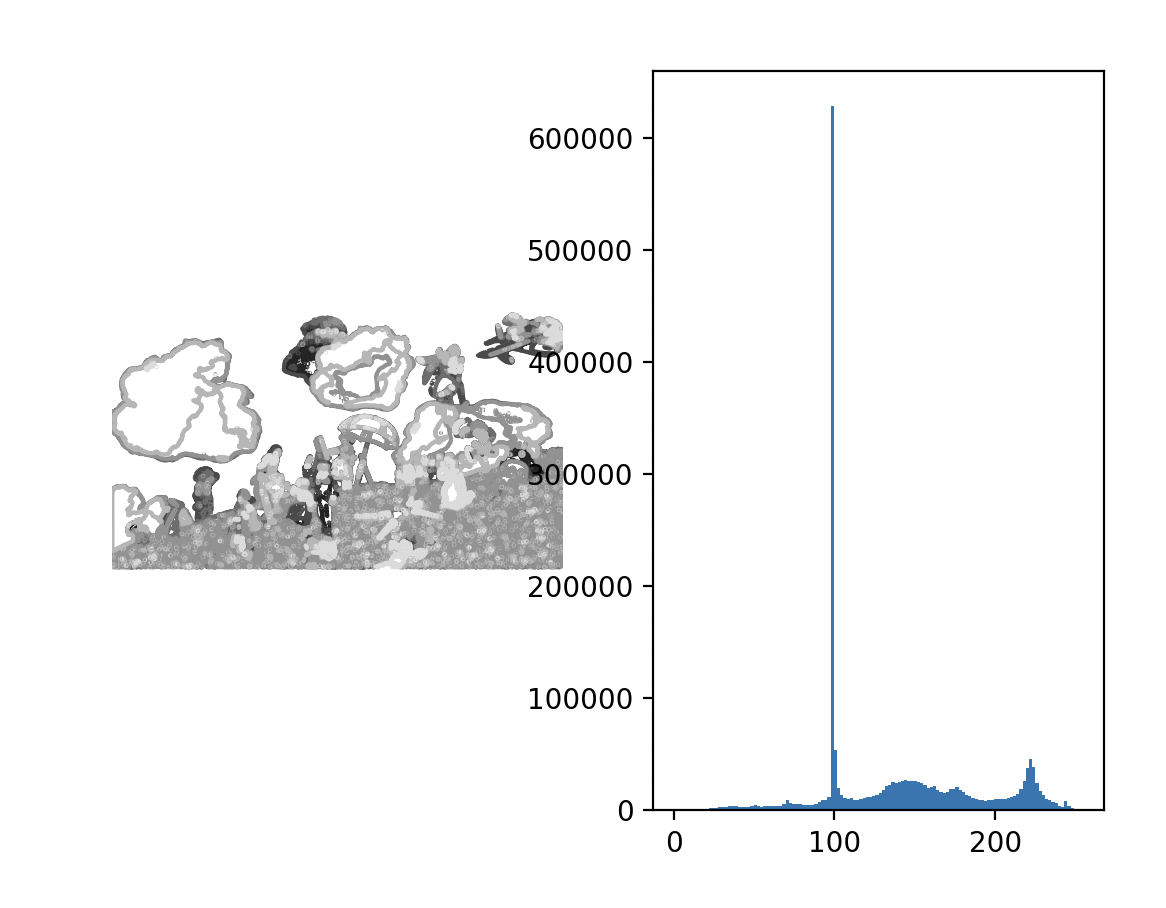
由直方图结果可以看出该灰度图像的亮度较高,灰度值主要集中在100-200之间。
1.2.3 交互式标注
from PIL import Image
from pylab import *
im=array(Image.open('picture1.jpg'))
imshow(im)
print('Please click 3 points')
g=ginput(3)
print("you clicked:",g)
show()
1.3 NumPy
NumPy是Python科学计算工具包,包含数组对象以及线性代数函数。
1.3.1 图像数组表示
from PIL import Image
from numpy import *
im=array(Image.open('picture1.jpg'))
print(im.shape,im.dtype)
im=array(Image.open('picture1.jpg').convert('L'),'f')
print(im.shape,im.dtype)
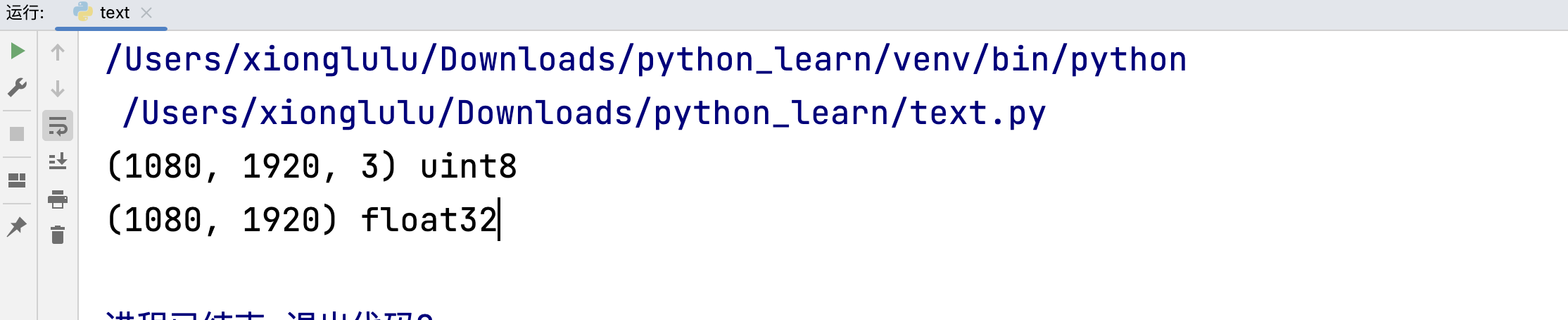
由结果可以看出每行的第一个元组表示图像数组的大小(行、列、颜色通道),紧接着的字符串表示数组元素的数据类型。
其中灰度图像没有颜色信息,所以在形状元组中,它只有两个数值。
1.3.2 灰度变换
from PIL import Image
from numpy import *
from pylab import *
im1=array(Image.open('picture1.jpg').convert('L'))
gray()
im2=255-im1
im3=(100.0/255)*im1+100
im4=255.0*(im1/255.0)**2
subplot(221)
axis('off')
imshow(im1)
subplot(222)
axis('off')
imshow(im2)
subplot(223)
axis('off')
imshow(im3)
subplot(224)
axis('off')
imshow(im4)
show()
print (int(im1.min()),int(im1.max()))
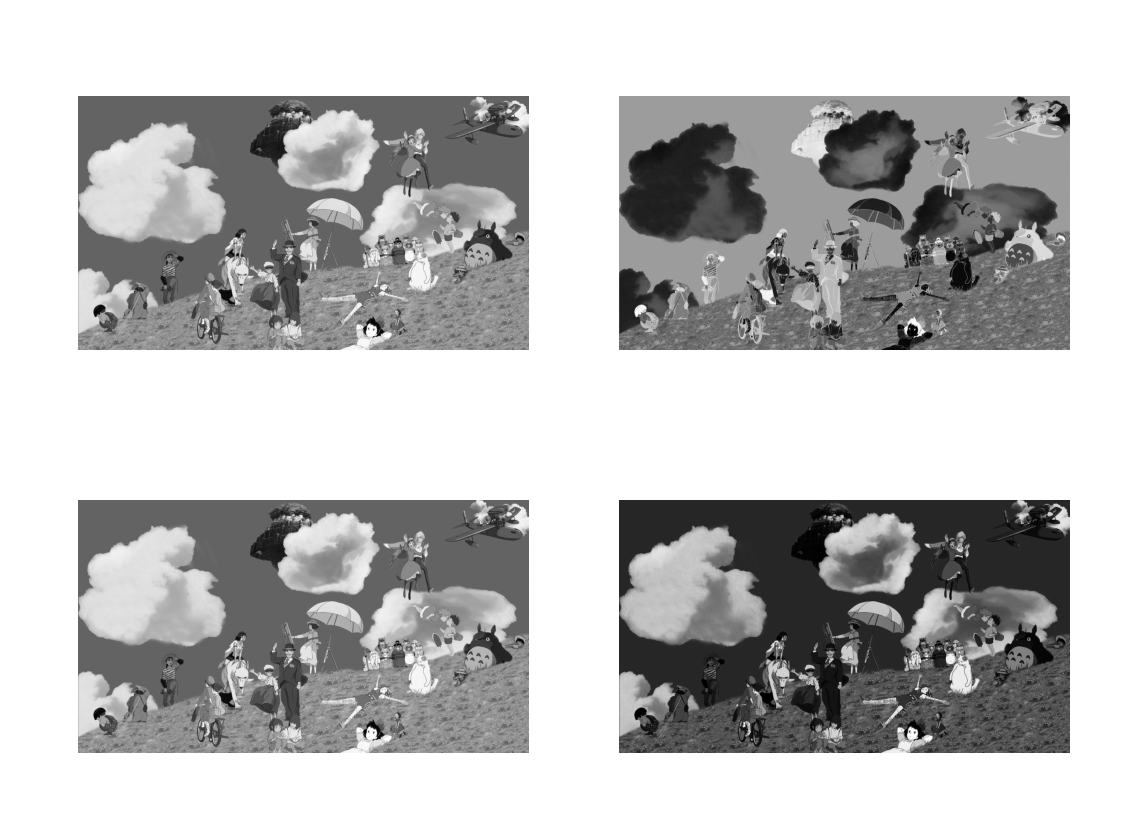
从结果可以看出,第二幅图是将灰度图像进行反相处理的结果,第三幅图是将图像的像素值变换到100-200区间的结果,最后一幅图是对图像进行二次函数变换的结果,使较暗的像素值变得更小。
1.3.3 直方图均衡
直方图均衡化是指将一幅图像的灰度直方图变平,使变换后的图像中每个灰度值的分布概率都相同。在对图像做进一步处理之前,直方图均衡化通常是对图像灰度值进行归一化的一个非常好的方法,并且可以增强图像的对比度。
直方图均衡化的变换函数是图像中像素值的累积分布函数( cumulative distribution function, 简写为 cdf,将像素值的范围映射到目标范围的归一化操作)。
def histeq(im,nbr_bins=256):
#计算图像直方图
imhist,bins=histogram(im.flatten(),nbr_bins,density=True)
cdf=imhist.cumsum()#累积分布函数
cdf=255*cdf/cdf[-1]#归一化
im2=interp(im.flatten(),bins[:-1],cdf) #使用累积分布函数的线性插值,计算新的像素值
return im2.reshape(im.shape),cdf
#绘制直方图
from PIL import Image
from pylab import *
im=array(Image.open('picture1.jpg').convert('L'))
im2,cdf=histeq(im)
figure()
gray()
subplot(221)
axis('off')
title(r'before')
imshow(im)
subplot(222)
axis('off')
title(r'after')
imshow(im2)
subplot(223)
hist(im.flatten(),128)
subplot(224)
hist(im2.flatten(),128)
show()
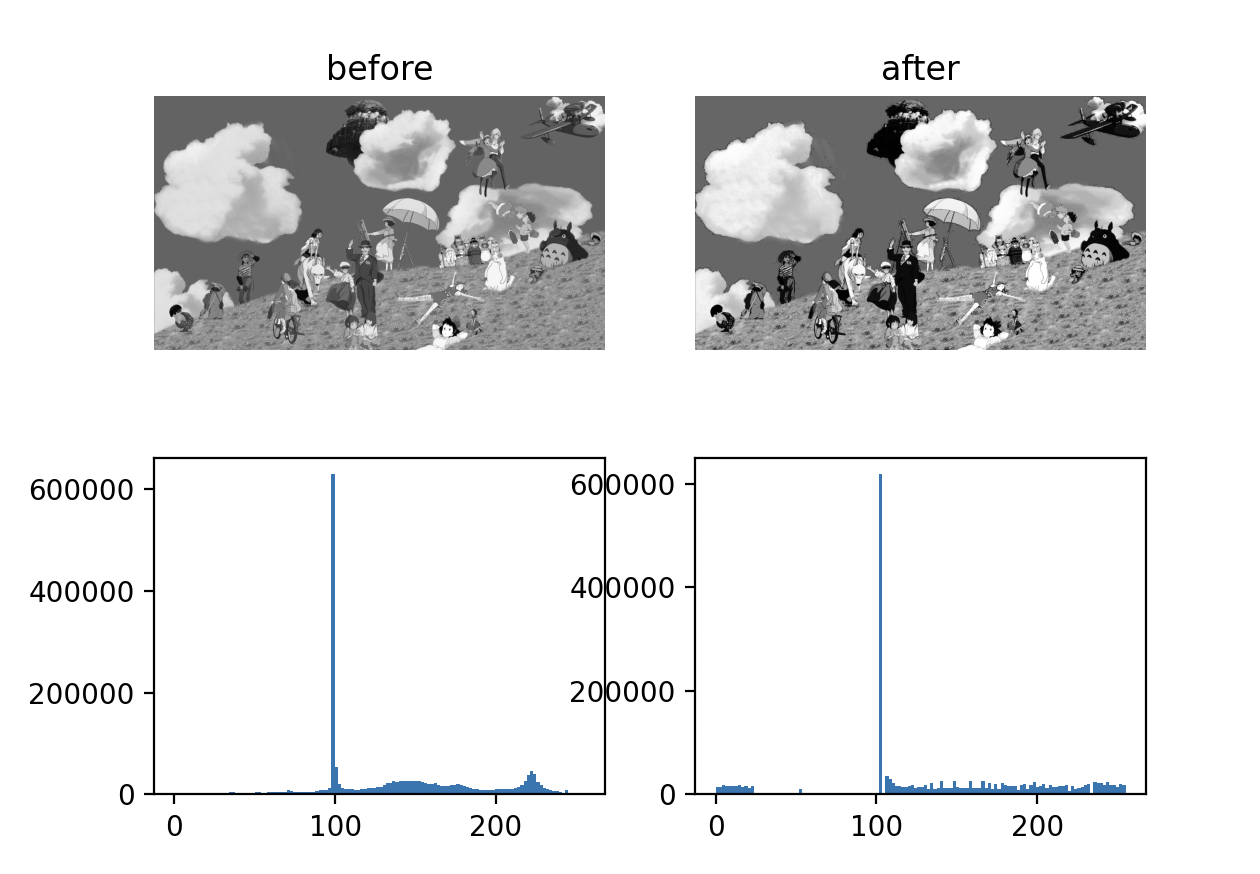
通过输出结果可以看出,直方图均衡化后图像的对比度增强了,原先图像灰色区域的细节变得清晰了。
1.4 SciPy
SciPy是建立在NumPy基础上,用于数值运算的工具包。可以实现数值积分、优化、统计、信号处理,以及图像处理功能。
1.4.1 图像模糊(高斯滤波)
图像的高斯模糊是将灰度图像I和一个高斯核进行卷积操作:其中*表示卷积操作,
是标准差为
的二维高斯核
from PIL import Image
from numpy import *
from pylab import *
from scipy.ndimage import gaussian_filter
im=array(Image.open('picture1.jpg'))
subplot(221)
axis('off')
title('before')
imshow(im)
# 不同标准差下进行图像滤波
for n,m in enumerate([2,5,10]):
# 数组初始化
im2=zeros(im.shape)
# 彩色图像高斯滤波
for i in range(3):
im2[:,:,i]=gaussian_filter(im[:,:,i],m)
im2=uint8(im2)
subplot(2,2,2+n)
title(f'σ={m}')
axis('off')
imshow(im2)
show()
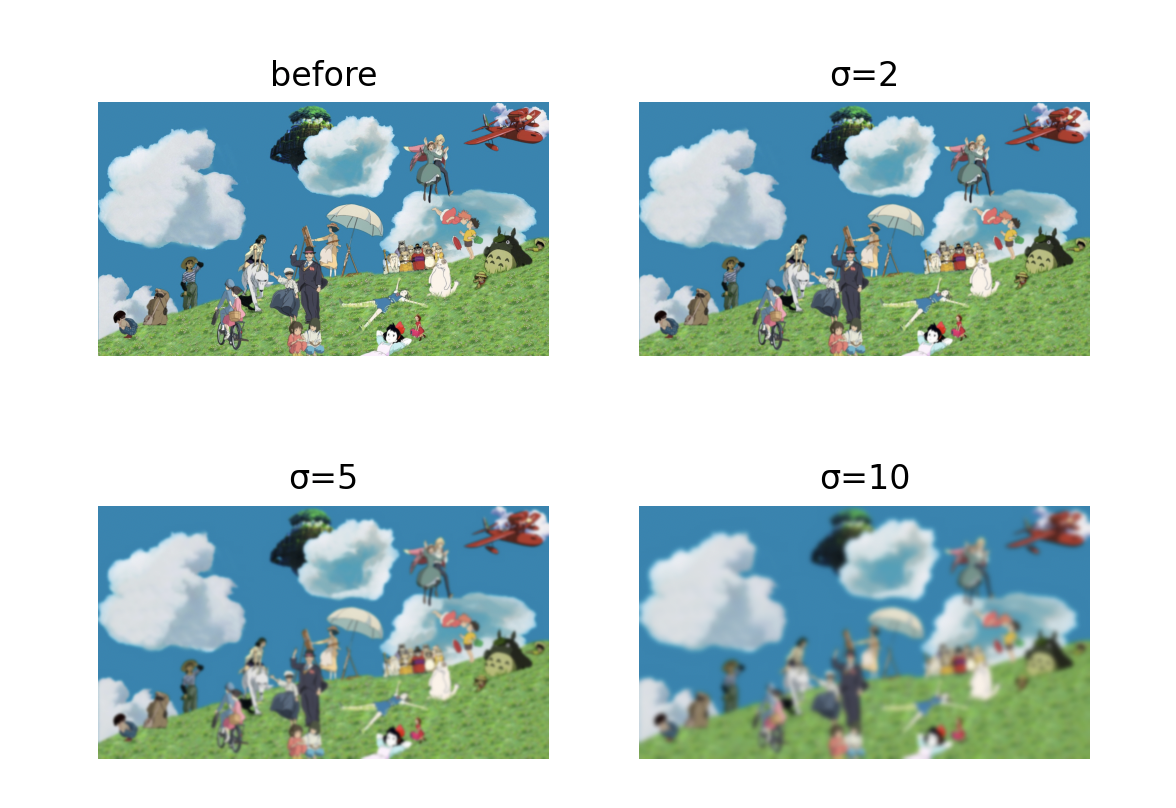
从输出结果可以看出,随着的增加,一幅图像被模糊的程度越大,图像丢失的细节越多。想要模糊彩色图像,对每一个颜色通道进行高斯模糊即可。