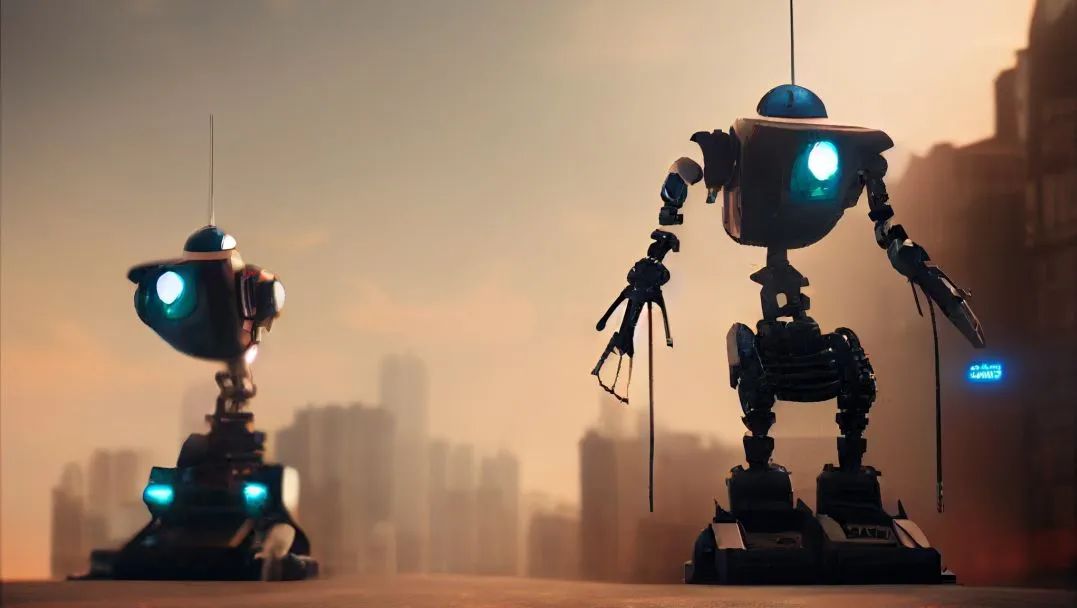
Author of this article:
Tarik Bennett, Beinan Wang, Hope Wang
This article will discuss the data access challenges in artificial intelligence (AI) and reveal that "commonly used NAS/NFS may not be the best choice . "
1. Early artificial intelligence/machine learning architecture
Gartner research shows that although large language models (LLM) have attracted much attention, the use of large language models by most organizations is still in the early stages, and only some have entered the production stage.
The focus of building an AI platform in the early stages is to get the system running so that project pilots and proof-of-concepts can be conducted. These early architectures, or pre-production architectures, are designed to meet the basic needs of model training and deployment. Currently, many organizations are already using this type of early AI architecture for production environments.
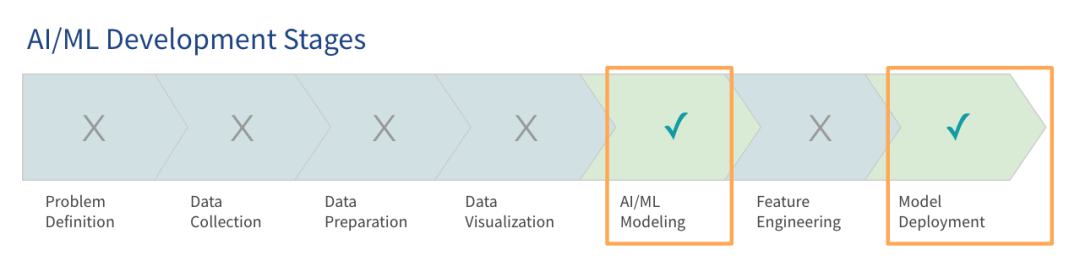
As data and models grow, such early AI architectures often become inefficient. Enterprises train models on the cloud, and as projects expand, their data and cloud usage are expected to increase significantly within 12 months. Many organizations start with data volumes that match their current memory sizes, but are aware of the need to prepare for larger loads.
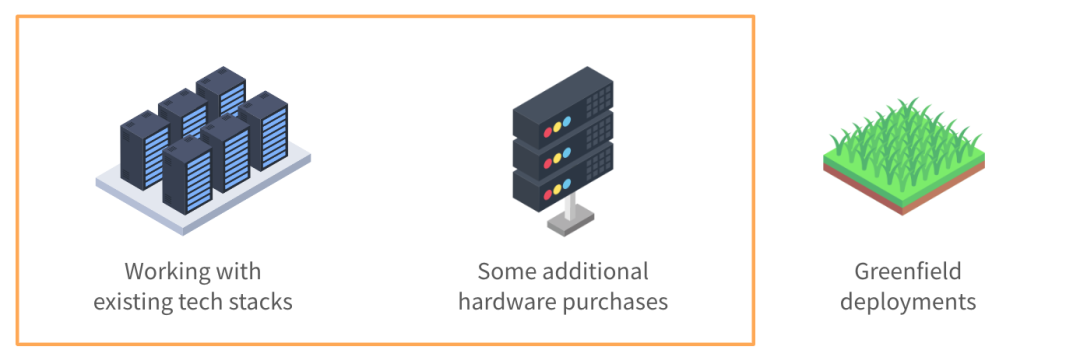
Enterprises may choose to use an existing technology stack or greenfield deployment. This article will focus on how to use your existing technology stack or purchase some additional hardware to design a more scalable, agile, and performant technology stack.
2. Challenges in data access
With the evolution of AI/ML architecture, the size of model training data sets continues to grow significantly, and the computing power and scale of GPUs are also rapidly increasing. In addition to computing, storage and network, we believe that data access is another key element in building a forward-looking AI platform .
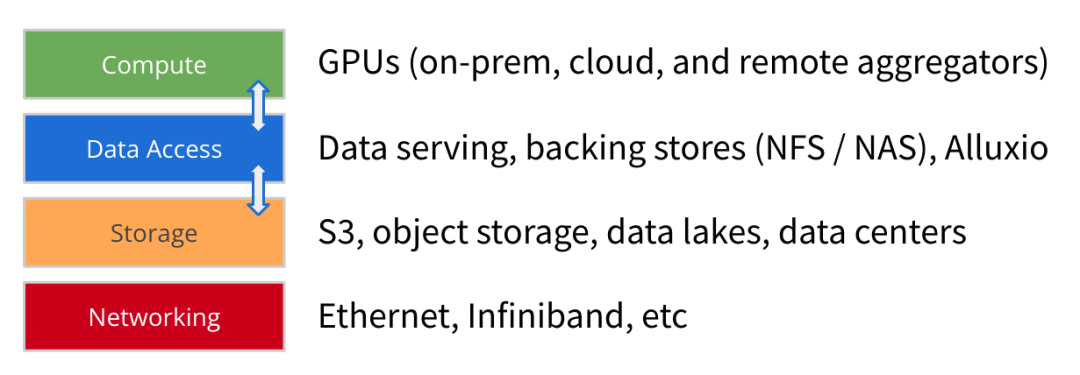
Data access refers to technologies such as data services, backup storage (NFS, NAS) and high-performance cache (such as Alluxio) that help the computing engine obtain data for model training and deployment.
The focus of data access is throughput and data loading efficiency, which is increasingly important for AI/ML architectures where GPU resources are scarce - optimizing data loading can greatly reduce GPU idle waiting time and improve GPU utilization. Therefore, high-performance data access should be the primary goal of architecture deployment.
As enterprises expand model training tasks on early AI architectures, some common data access challenges have emerged:
1
Model training efficiency is lower than expected: Due to data access bottlenecks, training time is longer than estimated based on computing resources. Low-throughput data streams do not provide sufficient data to the GPU.
2
Bottlenecks related to data synchronization: Manually copying or synchronizing data from storage to a local GPU server creates delays in building the data queue to be prepared.
3
Concurrency and metadata issues: When large jobs are launched in parallel, contention can occur on shared storage. Latency increases when metadata operations on the backend store are slow.
4
Slow performance or low GPU utilization: High-performance GPU infrastructure requires a huge investment, and once data access is inefficient, it will lead to idle and under-utilized GPU resources.
In addition, these challenges are compounded by a host of other issues that data teams need to manage. These problems include slow storage I/O speeds that cannot meet the needs of high-performance GPU clusters. Relying on manual data copying and synchronization increases latency while the data team waits for data to be delivered to the GPU server. The data access challenge is also compounded by the architectural complexity of multiple data silos in hybrid infrastructure or multi-cloud environments.
These issues ultimately result in the architecture's end-to-end efficiency falling short of expectations.
Challenges related to data access often have two common solutions.
Purchase faster storage: Many businesses try to solve the problem of slow data access by deploying faster storage options. Cloud vendors provide high-performance storage, while professional hardware vendors sell HPC storage to improve performance.
Add NAS/NFS on top of object storage: Adding centralized NAS or NFS as a backup to object storage such as S3, MinIO or Ceph is a common practice and helps teams consolidate data into shared file systems, simplifying users and workloads collaboration and sharing. In addition, you can also take advantage of related data management functions such as data consistency, availability, backup and scalability provided by mature NAS vendors.
However, these two common solutions above may not actually solve your problem.
Although faster storage and centralized NFS/NAS can gradually achieve some performance improvements, there are also drawbacks.
1
Faster storage means data migration, which can easily lead to data reliability issues
To take advantage of the high performance provided by dedicated storage, data must be migrated from existing storage to a new high-performance storage tier. This causes data to be migrated in the background. Migrating large data sets can result in extended transfer times and data reliability issues such as data corruption or loss during migration. While the team waits for data synchronization to complete, pausing operations can disrupt service and slow down project progress.
2
NFS/NAS: Maintenance and bottlenecks
As an additional storage layer, NFS/NAS maintenance, stability and scalability challenges remain. Manually copying data from NFS/NAS to a local GPU server will increase latency and waste resources caused by repeated backups. Surges in read demand caused by parallel jobs can cluster NFS/NAS servers and interconnected services. In addition, data synchronization problems in remote NFS/NAS GPU clusters still exist.
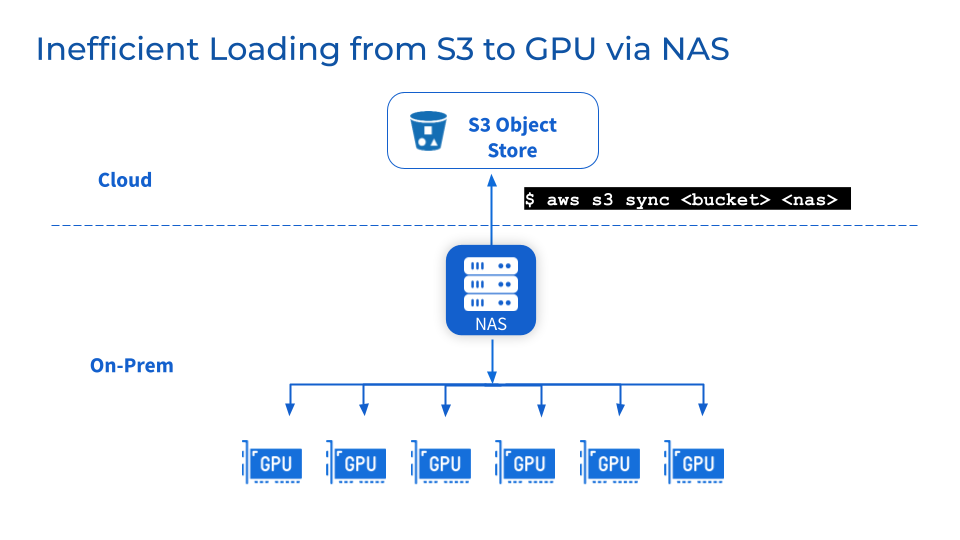
3
What if I need to change suppliers for business reasons?
Businesses may switch suppliers due to cost optimization or contractual reasons. The flexibility of multi-cloud environments requires the ability to easily port large data sets without vendor lock-in. However, moving petabyte-scale data storage can cause significant downtime and disruption to model development.
In short, existing solutions, while helpful in the short term, cannot provide a scalable and optimized data access architecture to meet the exponential growth of data needs of AI/ML.
3. Solutions provided by Alluxio
Alluxio can be deployed between compute and data sources. Provide data abstraction and distributed caching to improve the performance and scalability of AI/ML architecture.
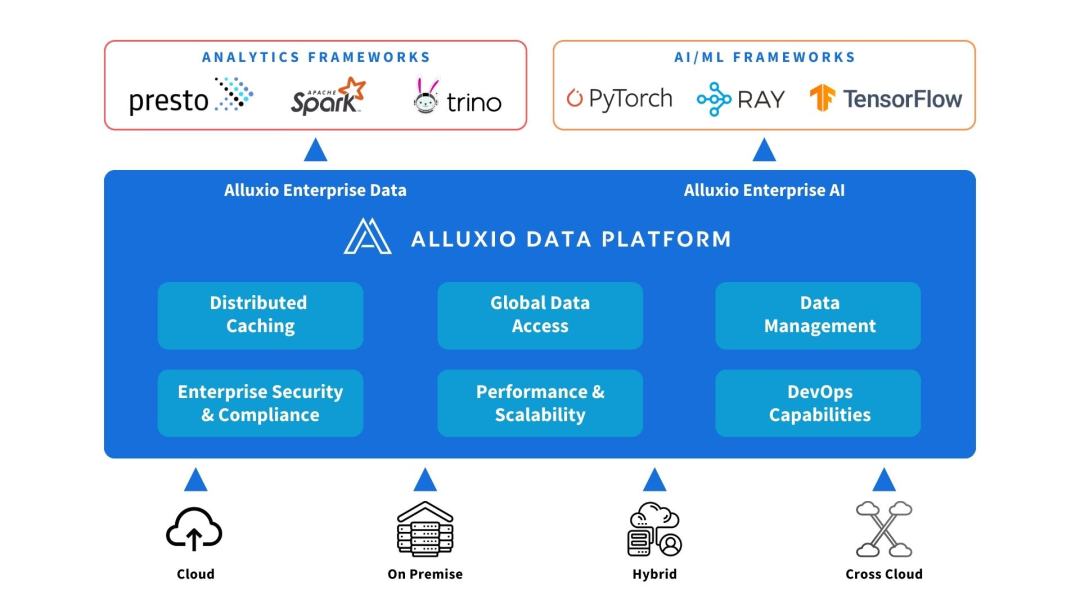
Alluxio helps solve the challenges faced by early AI architectures in scalability, performance and data management as the amount of data, model complexity increases, and GPU clusters expand.
1
increase capacity
Alluxio scales beyond the limit of a single node to accommodate larger training data sets than cluster memory or local SSDs can accommodate. It connects different storage systems and provides a unified data access layer to mount petabyte-level data lakes. Alluxio intelligently caches frequently used files and metadata in memory and SSD tiers close to the compute, eliminating the need to copy the entire data set.
2
Reduce data management
Alluxio simplifies data movement and storage between GPU clusters through automated distributed caching. Data teams don't need to manually copy or sync data to local staging files. The Alluxio cluster can automatically capture hot files or objects to a location close to the computing node without going through complex workflow operations. Alluxio simplifies workflows even with 50 million or more objects per node.
3
Improve performance
Alluxio is built to accelerate workloads, eliminating the I/O bottlenecks in traditional storage that limit GPU throughput. Distributed caching increases data access speed by orders of magnitude. Compared with accessing remote storage through the network, Alluxio provides local data access at the memory and SSD levels, thereby improving GPU utilization.
In short, Alluxio provides a high-performance and scalable data access layer that can maximize the use of GPU resources in AI/ML data expansion scenarios.
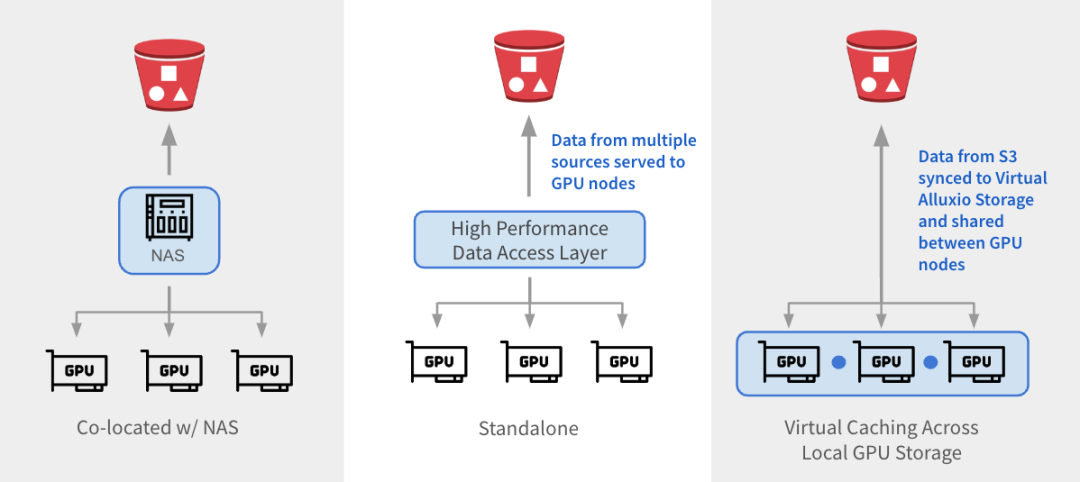
Alluxio can be integrated with existing architectures in three ways.
1
与 NAS 并置:Alluxio 作为透明缓存层与现有 NAS并置部署,增强 I/O 性能。Alluxio将NAS中的活跃数据缓存在跨GPU节点的本地 SSD 中。作业将读取请求重新定向到Alluxio上的SSD缓存,绕过NAS,从而消除NAS瓶颈。写入操作通过 Alluxio 对 SSD 进行低延迟写入,然后异步持久化保存到 NAS中。
2
独立数据访问层:Alluxio 作为专用的高性能数据访问层,整合来自 S3、HDFS、NFS 或本地数据湖等多个数据源的数据,为GPU节点提供数据访问服务。Alluxio 将不同的数据孤岛统一在一个命名空间下,并将存储后端挂载为底层存储。经常访问的数据会被缓存在 Alluxio Worker节点的SSD中,从而加速GPU对数据的本地访问。
3
跨GPU存储的虚拟缓存:Alluxio充当跨本地GPU存储的虚拟缓存。S3中的数据会被同步到虚拟 Alluxio存储并在GPU节点之间共享,无需在节点之间手动拷贝数据。
1
参考架构
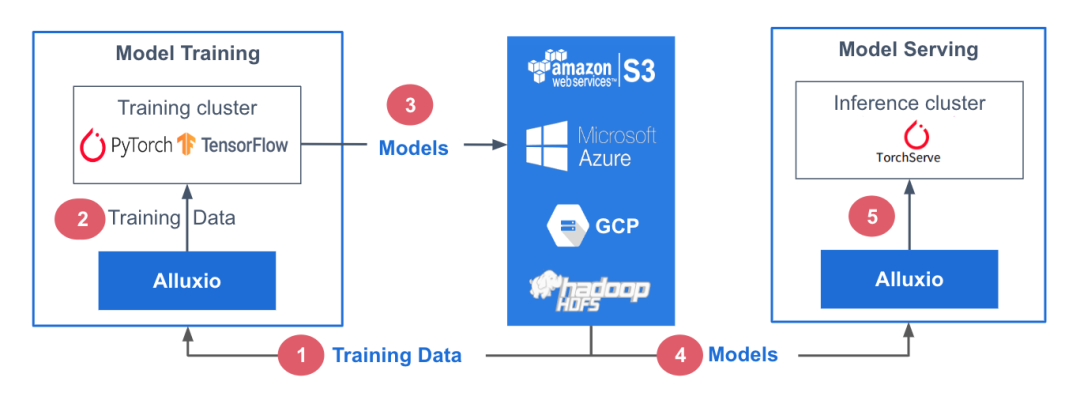
在此参考架构中,训练数据存储在中心化数据存储平台AWS S3中。Alluxio可帮助实现模型训练集群对训练数据的无缝访问。PyTorch、TensorFlow、scikit-learn和XGBoost等ML训练框架都在CPU/GPU/TPU集群上层执行。这些框架利用训练数据生成机器学习模型,模型生成后被存储在中心化模型库中。
在模型服务阶段,使用专用服务/推理集群,并采用TorchServe、TensorFlow Serving、Triton 和 KFServing等框架。这些服务集群利用Alluxio从模型存储库中获取模型。模型加载后,服务集群会处理输入的查询、执行必要的推理作业并返回计算结果。
训练和服务环境都基于Kubernetes,有助于增强基础设施的可扩展性和可重复性。
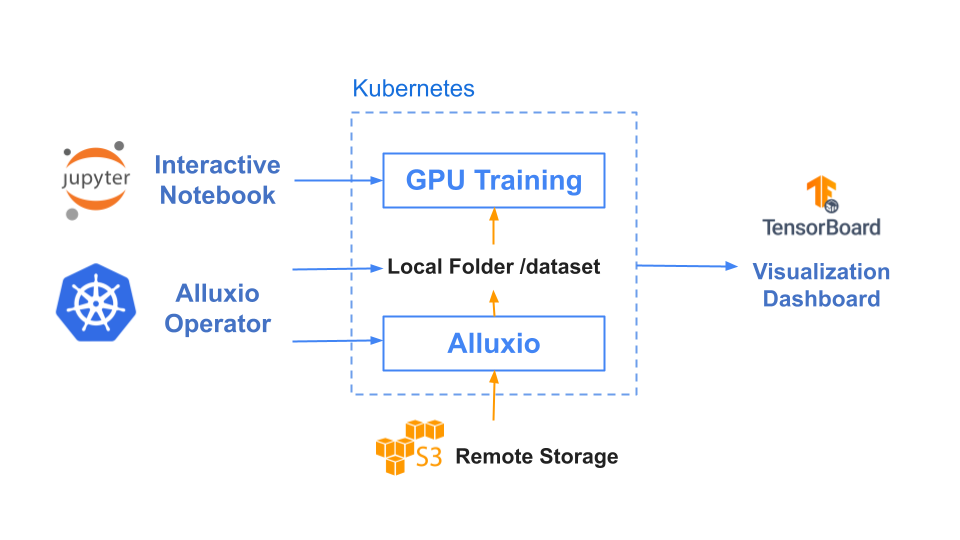
2
基准测试结果
在本基准测试中,我们用计算机视觉领域的典型应用场景之一——图片分类任务作为示例,其中我们以ImageNet的数据集作为训练集,通过ResNet来训练图片分类模型。
基于Resnet-50上3个epochs性能基准测试的结果,使用Alluxio比使用S3-FUSE的速度快5倍。一般来说,提高数据访问性能可缩短模型训练的总时间。
|
Alluxio |
S3 - FUSE |
Total training time (3 epochs) |
17 minutes |
85 minutes |
使用Alluxio后,GPU利用率得到大幅提升。Alluxio将数据加载时间由82%缩短至1%,从而将GPU利用率由17%提升至93%。
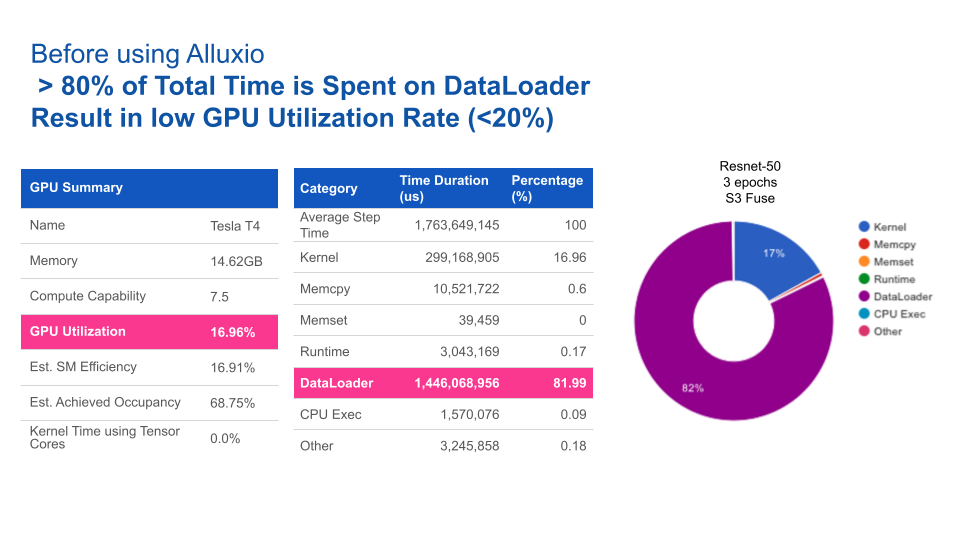
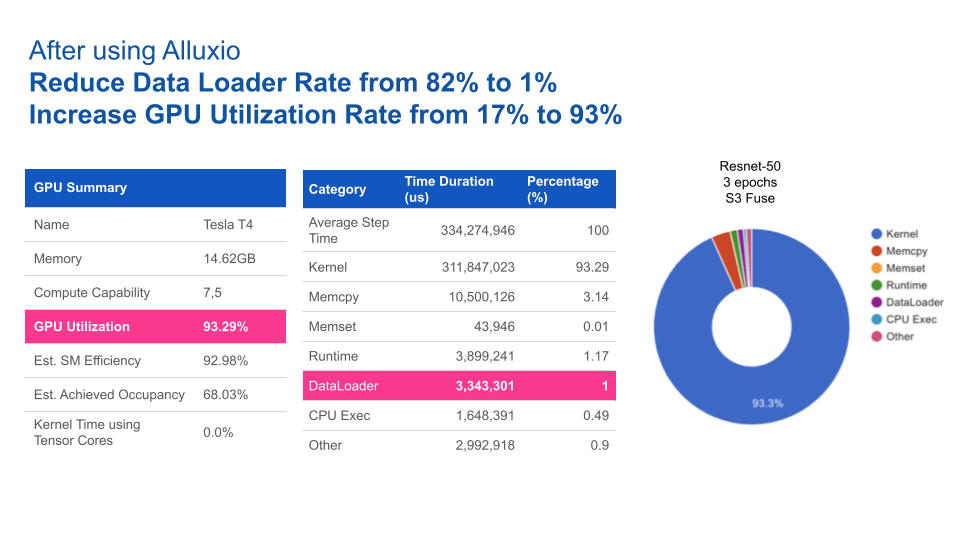
四、结论
随着AI/ML学习架构从早期的预生产架构向着可扩展架构发展,数据访问始终是瓶颈。仅靠添加更快的存储硬件或中心化NAS/NFS无法完全消除性能不达标以及影响系统操作的管理问题。
Alluxio提供了一种专为优化AI/ML任务数据流而设计的软件解决方案。与传统存储方案相比,其优势包括:
1
优化数据加载:Alluxio智能地加速训练任务和模型服务的数据访问,从而将GPU利用率最大化。
2
维护需求低:无需在节点或集群之间手动拷贝数据。Alluxio通过其分布式缓存层处理热文件传输。
3
支持扩展:当数据量大到需要扩展更多节点的情况下,Alluxio也能维持性能稳定。Alluxio通过使用SSD扩展内存,可缓存任何大小的文件,避免拷贝全部文件。
4
更快的切换:Alluxio将底层存储抽象化,使得数据团队能够轻松地在云厂商、本地或多云环境中迁移数据。数据迁移无需替换硬件,也不会导致停机。
部署Alluxio后,企业通过针对数据访问进行优化的数据架构,可以构建出性能卓越、可扩展的数据平台,从而加速模型开发,满足不断增长的数据需求。
✦
【近期热门】
✦
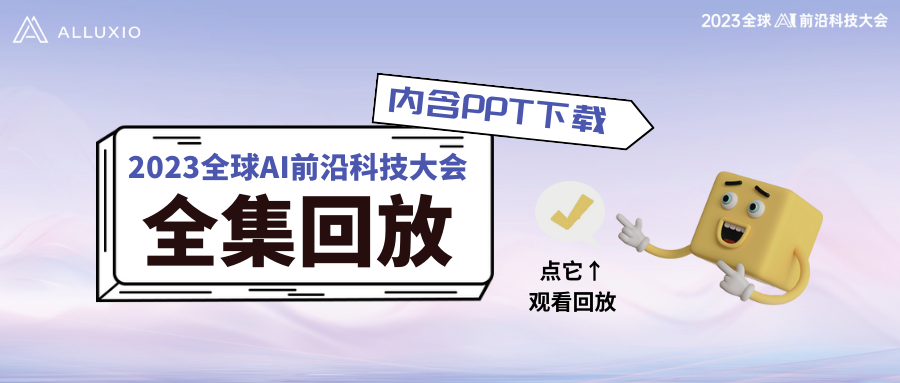
✦
【宝典集市】
✦
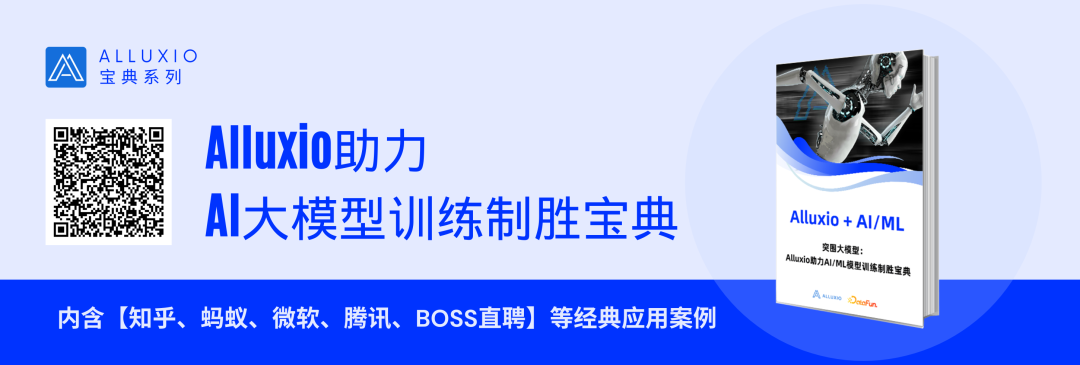
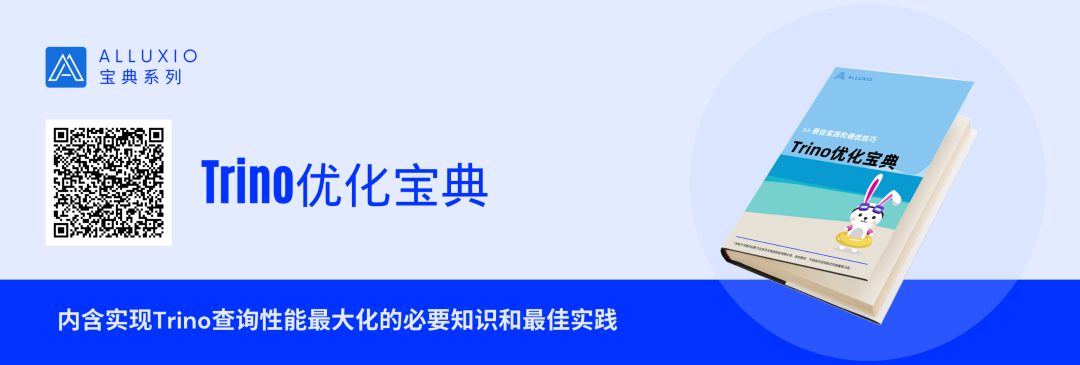
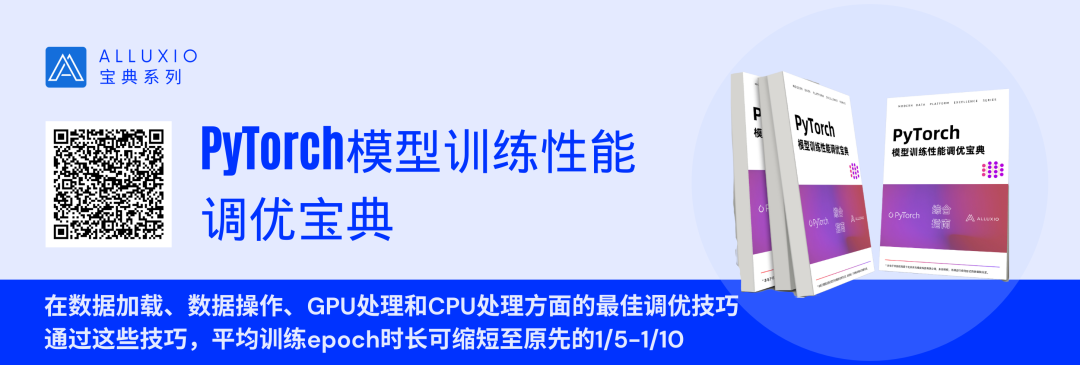
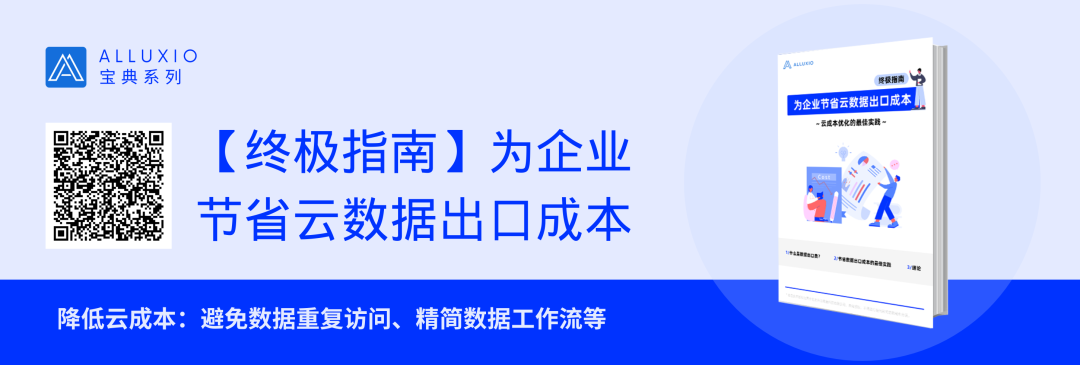
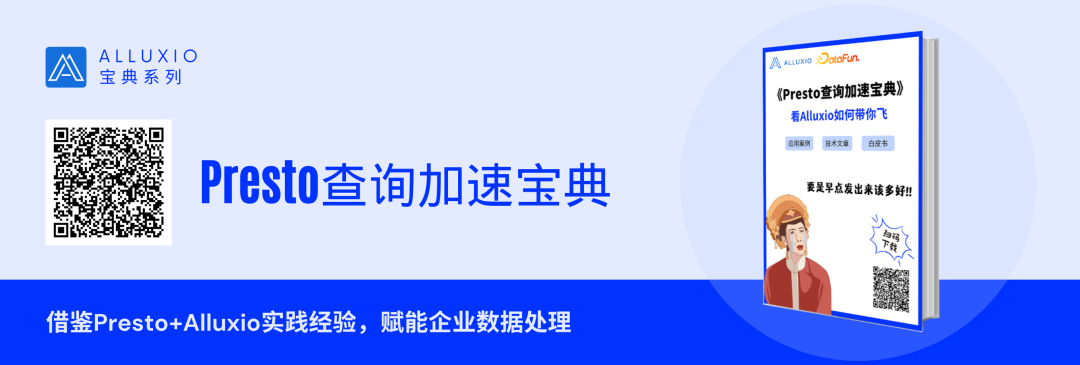
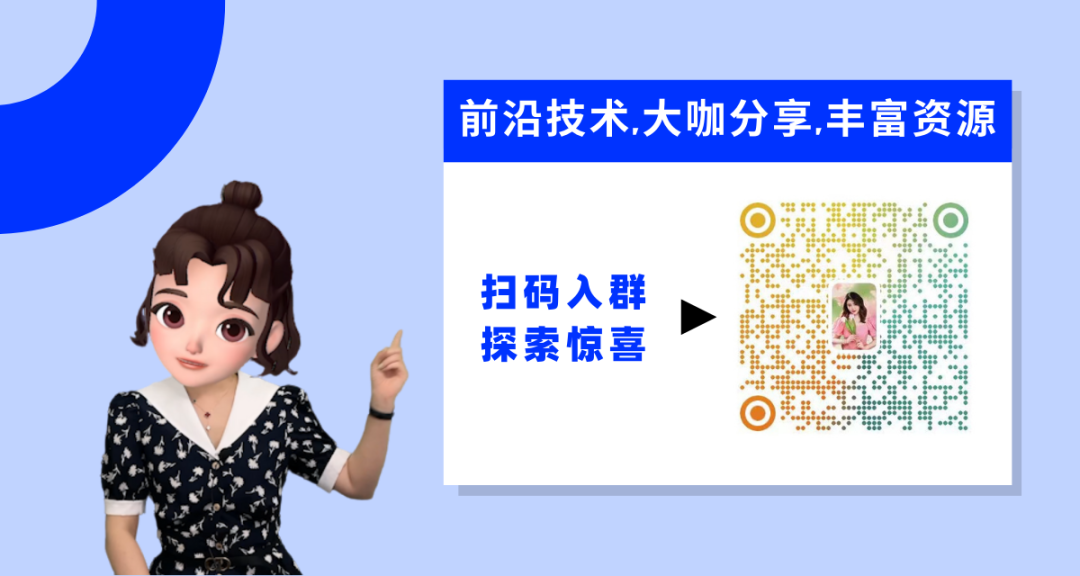
本文分享自微信公众号 - Alluxio(Alluxio_China)。
如有侵权,请联系 [email protected] 删除。
本文参与“OSC源创计划”,欢迎正在阅读的你也加入,一起分享。