继续跟着高博的博客学习,到了第三篇:
http://www.cnblogs.com/gaoxiang12/p/4659805.html
第三篇其实是分了两个部分:一个就是将产生点云这个功能封装成一个库;另外一个就是将图像匹配得位姿变换。
将产生点云功能封装成一个库:
首先说一下,一个程序编译链接之后,并不一定都生成可执行程序,有的是生成了库,以备其他程序调用。所以这两个有点并行的意味。首先,一个库要有头文件和库文件:
头文件slamBase.h,放在include文件夹中:
//
// Created by robin on 18-1-29.
//
//# pragma once
// 各种头文件
// C++标准库
#include <fstream>
#include <vector>
using namespace std;
// OpenCV
#include <opencv2/core/core.hpp>
#include <opencv2/highgui/highgui.hpp>
//PCL
#include <pcl/io/pcd_io.h>
#include <pcl/point_types.h>
// 类型定义
typedef pcl::PointXYZRGBA PointT;
typedef pcl::PointCloud<PointT> PointCloud;
// 相机内参结构
struct CAMERA_INTRINSIC_PARAMETERS
{
double cx, cy, fx, fy, scale;
};
// 函数接口
// image2PonitCloud 将rgb图转换为点云
PointCloud::Ptr image2PointCloud( cv::Mat& rgb, cv::Mat& depth, CAMERA_INTRINSIC_PARAMETERS& camera );
// point2dTo3d 将单个点从图像坐标转换为空间坐标
// input: 3维点Point3f (u,v,d),注意下这里比较奇葩,这个三维向量并不是坐标,没有什么实际意义,仅仅是一个数列,分别存储了像素坐标uv和深度数据d。用于给函数传递参数。
cv::Point3f point2dTo3d( cv::Point3f& point, CAMERA_INTRINSIC_PARAMETERS& camera );
内容很简单,就是定义了一个存储相机内参的结构体,定义了两个功能函数。
再看src中的源文件slamBase.cpp:
//
// Created by robin on 18-1-29.
//
#include "slamBase.h"
PointCloud::Ptr image2PointCloud( cv::Mat& rgb, cv::Mat& depth, CAMERA_INTRINSIC_PARAMETERS& camera )
{
PointCloud::Ptr cloud ( new PointCloud );
for (int m = 0; m < depth.rows; m++)
for (int n=0; n < depth.cols; n++)
{
// 获取深度图中(m,n)处的值
ushort d = depth.ptr<ushort>(m)[n];
// d 可能没有值,若如此,跳过此点
if (d == 0)
continue;
// d 存在值,则向点云增加一个点
PointT p;
// 计算这个点的空间坐标
p.z = double(d) / camera.scale;
p.x = (n - camera.cx) * p.z / camera.fx;
p.y = (m - camera.cy) * p.z / camera.fy;
// 从rgb图像中获取它的颜色
// rgb是三通道的BGR格式图,所以按下面的顺序获取颜色
p.b = rgb.ptr<uchar>(m)[n*3];
p.g = rgb.ptr<uchar>(m)[n*3+1];
p.r = rgb.ptr<uchar>(m)[n*3+2];
// 把p加入到点云中
cloud->points.push_back( p );
}
// 设置并保存点云
cloud->height = 1;
cloud->width = cloud->points.size();
cloud->is_dense = false;
return cloud;
}
cv::Point3f point2dTo3d( cv::Point3f& point, CAMERA_INTRINSIC_PARAMETERS& camera )
{
cv::Point3f p; // 3D 点
p.z = double( point.z ) / camera.scale;
p.x = ( point.x - camera.cx) * p.z / camera.fx;
p.y = ( point.y - camera.cy) * p.z / camera.fy;
return p;
}
更明了,include进来头文件后,写两个功能函数的定义就好了。
主要说的是CMakeLists.txt的书写,按照动改哪个文件夹的文件就改动哪个文件夹中的CMakeLists.txt的原则,我们对src中的文件做了改动(写了个slamBase.cpp文件嘛~),所以要改动的也是src中的CMakeLists.txt。
首先,最直观的就是我们要生成一个库,那add_library和target_link_libraries不就好了么,生成库,并将库链接到依赖库上,所以在原来的CMakeLists.txt基础上增加两句:
# 增加库文件slamBase
add_library(slambase slamBase.cpp )
target_link_libraries(slambase ${OpenCV_LIBS} ${PCL_LIBS})
OK,这就好了~~~我们点击编译一下看看:
OK,没毛病,在lib中生成了一个名为libslambase.a的静态库文件。这里注意,虽然库文件名叫libslambase.a,但是这个库的名称还是叫slambase(add_library中定义的名字),所以后面使用时,链接此库的时候,就是用它的库名slambase,跟它的头文件名称slamBase.h,源文件名称slamBase.cpp,库文件名称libslambase.a都没关系!
第二部分,书写detectFeatures.cpp,
高博原始的代码:
#include<iostream>
#include "slamBase.h"
using namespace std;
// OpenCV 特征检测模块
#include <opencv2/features2d/features2d.hpp>
#include <opencv2/nonfree/nonfree.hpp>
#include <opencv2/calib3d/calib3d.hpp>
int main( int argc, char** argv )
{
// 声明并从data文件夹里读取两个rgb与深度图
cv::Mat rgb1 = cv::imread( "./data/rgb1.png");
cv::Mat rgb2 = cv::imread( "./data/rgb2.png");
cv::Mat depth1 = cv::imread( "./data/depth1.png", -1);
cv::Mat depth2 = cv::imread( "./data/depth2.png", -1);
// 声明特征提取器与描述子提取器
cv::Ptr<cv::FeatureDetector> _detector;
cv::Ptr<cv::DescriptorExtractor> _descriptor;
// 构建提取器,默认两者都为sift
// 构建sift, surf之前要初始化nonfree模块
cv::initModule_nonfree();
_detector = cv::FeatureDetector::create( "GridSIFT" );
_descriptor = cv::DescriptorExtractor::create( "SIFT" );
vector< cv::KeyPoint > kp1, kp2; //关键点
_detector->detect( rgb1, kp1 ); //提取关键点
_detector->detect( rgb2, kp2 );
cout<<"Key points of two images: "<<kp1.size()<<", "<<kp2.size()<<endl;
// 可视化, 显示关键点
cv::Mat imgShow;
cv::drawKeypoints( rgb1, kp1, imgShow, cv::Scalar::all(-1), cv::DrawMatchesFlags::DRAW_RICH_KEYPOINTS );
cv::imshow( "keypoints", imgShow );
cv::imwrite( "./data/keypoints.png", imgShow );
cv::waitKey(0); //暂停等待一个按键
// 计算描述子
cv::Mat desp1, desp2;
_descriptor->compute( rgb1, kp1, desp1 );
_descriptor->compute( rgb2, kp2, desp2 );
// 匹配描述子
vector< cv::DMatch > matches;
cv::FlannBasedMatcher matcher;
matcher.match( desp1, desp2, matches );
cout<<"Find total "<<matches.size()<<" matches."<<endl;
// 可视化:显示匹配的特征
cv::Mat imgMatches;
cv::drawMatches( rgb1, kp1, rgb2, kp2, matches, imgMatches );
cv::imshow( "matches", imgMatches );
cv::imwrite( "./data/matches.png", imgMatches );
cv::waitKey( 0 );
// 筛选匹配,把距离太大的去掉
// 这里使用的准则是去掉大于四倍最小距离的匹配
vector< cv::DMatch > goodMatches;
double minDis = 9999;
for ( size_t i=0; i<matches.size(); i++ )
{
if ( matches[i].distance < minDis )
minDis = matches[i].distance;
}
for ( size_t i=0; i<matches.size(); i++ )
{
if (matches[i].distance < 4*minDis)
goodMatches.push_back( matches[i] );
}
// 显示 good matches
cout<<"good matches="<<goodMatches.size()<<endl;
cv::drawMatches( rgb1, kp1, rgb2, kp2, goodMatches, imgMatches );
cv::imshow( "good matches", imgMatches );
cv::imwrite( "./data/good_matches.png", imgMatches );
cv::waitKey(0);
// 计算图像间的运动关系
// 关键函数:cv::solvePnPRansac()
// 为调用此函数准备必要的参数
// 第一个帧的三维点
vector<cv::Point3f> pts_obj;
// 第二个帧的图像点
vector< cv::Point2f > pts_img;
// 相机内参
CAMERA_INTRINSIC_PARAMETERS C;
C.cx = 325.5;
C.cy = 253.5;
C.fx = 518.0;
C.fy = 519.0;
C.scale = 1000.0;
for (size_t i=0; i<goodMatches.size(); i++)
{
// query 是第一个, train 是第二个
cv::Point2f p = kp1[goodMatches[i].queryIdx].pt;
// 获取d是要小心!x是向右的,y是向下的,所以y才是行,x是列!
ushort d = depth1.ptr<ushort>( int(p.y) )[ int(p.x) ];
if (d == 0)
continue;
pts_img.push_back( cv::Point2f( kp2[goodMatches[i].trainIdx].pt ) );
// 将(u,v,d)转成(x,y,z)
cv::Point3f pt ( p.x, p.y, d );
cv::Point3f pd = point2dTo3d( pt, C );
pts_obj.push_back( pd );
}
double camera_matrix_data[3][3] = {
{C.fx, 0, C.cx},
{0, C.fy, C.cy},
{0, 0, 1}
};
// 构建相机矩阵
cv::Mat cameraMatrix( 3, 3, CV_64F, camera_matrix_data );
cv::Mat rvec, tvec, inliers;
// 求解pnp
cv::solvePnPRansac( pts_obj, pts_img, cameraMatrix, cv::Mat(), rvec, tvec, false, 100, 1.0, 100, inliers );
cout<<"inliers: "<<inliers.rows<<endl;
cout<<"R="<<rvec<<endl;
cout<<"t="<<tvec<<endl;
// 画出inliers匹配
vector< cv::DMatch > matchesShow;
for (size_t i=0; i<inliers.rows; i++)
{
matchesShow.push_back( goodMatches[inliers.ptr<int>(i)[0]] );
}
cv::drawMatches( rgb1, kp1, rgb2, kp2, matchesShow, imgMatches );
cv::imshow( "inlier matches", imgMatches );
cv::imwrite( "./data/inliers.png", imgMatches );
cv::waitKey( 0 );
return 0;
}
内容就不说了,逻辑清晰明了。注意一个点就是一定不要忘了将我们自己写的库的头文件include进来:#include "slamBase.h"
相应的还有CMakeLists.txt日常基本操作,添加:
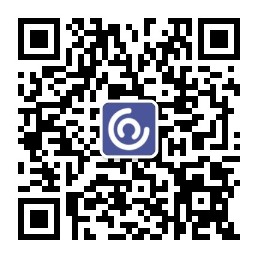
# 增加特征点匹配的可执行文件
add_executable(detectfeacures detectFeacures.cpp )
# 链接上我们自己写的库
target_link_libraries(detectfeacures slambase )
OK,编译日常不通过。。。
首先第一个报错:
CMakeFiles\untitled1.dir/objects.a(main.cpp.obj): In function `_static_initialization_and_destruction_0':
C:/www/cpp/boost_1_62_0/boost/system/error_code.hpp:221: undefined reference to `boost::system::generic_category()'
C:/www/cpp/boost_1_62_0/boost/system/error_code.hpp:222: undefined reference to `boost::system::generic_category()'
C:/www/cpp/boost_1_62_0/boost/system/error_code.hpp:223: undefined reference to `boost::system::system_category()'
以上是解决后网上找的相同报错代码,所以文件名称可能不一样,但是不要在意细节。。。重点是那三个undefined reference:
undefined reference to `boost::system::generic_category()'
undefined reference to `boost::system::generic_category()'
undefined reference to `boost::system::system_category()'
未定义的引用?百度:
https://segmentfault.com/q/1010000007420187?sort=created
大概的原因就是没有链接boost_system库,按照引用答案更改:
首先在CMakeLists.txt中增加添加boost_system库语句:
# 由于需要链接boost库,所以需要find_package和include_directries进 boost库
find_package(Boost REQUIRED COMPONENTS system)
include_directories(${Boost_INCLUDE_DIRS})
之后还需要将编译的文件链接上,将target_link_libraries改为:
# 这里也是将boost库链接进来
target_link_libraries(detectfeacures ${Boost_LIBRARIES} slambase )
OK,继续编译。。。旧问题解决了,日常新问题,主要就是因为OpenCV日常更新后的变化导致,有三个:
1、声明特征提取器与描述子提取器的创建改为ORB了:
// 声明特征提取器与描述子提取器
cv::Ptr<cv::FeatureDetector> _detector = cv::ORB::create();;
cv::Ptr<cv::DescriptorExtractor> _descriptor = cv::ORB::create();
2、匹配器matcher的创建要随动ORB,改成Brute Force match:
//cv::FlannBasedMatcher matcher; //这是高博的
auto matcher = cv::DescriptorMatcher::create ( "BruteForce-Hamming" );
3、solvePnPRansac函数增加了confidence参数:算法产生有用结果的置信系数。具体改动就是增加一个参数值(这里就用函数默认值0.99好了。。。):
cv::solvePnPRansac( pts_obj, pts_img, cameraMatrix, cv::Mat(), rvec, tvec, false, 100, 1.0, 0.99, inliers );
这三个改动在高博博客里开头都有说,不过没找github上最新的,自己改改也有好处,参见了以下博客:
http://blog.csdn.net/wuliyanyan/article/details/55805238?locationNum=4&fps=1
改动完最终测试能跑通的代码:
//
// Created by robin on 18-1-29.
//
#include<iostream>
#include "slamBase.h"
using namespace std;
// OpenCV 特征检测模块
#include <opencv2/core/core.hpp>
#include <opencv2/features2d/features2d.hpp>
#include <opencv2/highgui/highgui.hpp>
#include <opencv2/calib3d/calib3d.hpp>
int main( int argc, char** argv )
{
// 声明并从data文件夹里读取两个rgb与深度图
cv::Mat rgb1 = cv::imread( "../data/rgb1.png");
cv::Mat rgb2 = cv::imread( "../data/rgb2.png");
cv::Mat depth1 = cv::imread( "../data/depth1.png", -1);
cv::Mat depth2 = cv::imread( "../data/depth2.png", -1);
// 声明特征提取器与描述子提取器
cv::Ptr<cv::FeatureDetector> _detector = cv::ORB::create();;
cv::Ptr<cv::DescriptorExtractor> _descriptor = cv::ORB::create();
vector< cv::KeyPoint > kp1, kp2; //关键点
_detector->detect( rgb1, kp1 ); //提取关键点
_detector->detect( rgb2, kp2 );
cout<<"Key points of two images: "<<kp1.size()<<", "<<kp2.size()<<endl;
// 可视化, 显示关键点
cv::Mat imgShow;
cv::drawKeypoints( rgb1, kp1, imgShow, cv::Scalar::all(-1), cv::DrawMatchesFlags::DRAW_RICH_KEYPOINTS );
cv::imshow( "keypoints", imgShow );
cv::imwrite( "../data/keypoints.png", imgShow );
cv::waitKey(0); //暂停等待一个按键
// 计算描述子
cv::Mat desp1, desp2;
_descriptor->compute( rgb1, kp1, desp1 );
_descriptor->compute( rgb2, kp2, desp2 );
// 匹配描述子
vector< cv::DMatch > matches;
//cv::FlannBasedMatcher matcher;
auto matcher = cv::DescriptorMatcher::create ( "BruteForce-Hamming" );
matcher->match( desp1, desp2, matches );
cout<<"Find total "<<matches.size()<<" matches."<<endl;
// 可视化:显示匹配的特征
cv::Mat imgMatches;
cv::drawMatches( rgb1, kp1, rgb2, kp2, matches, imgMatches );
cv::imshow( "matches", imgMatches );
cv::imwrite( "../data/matches.png", imgMatches );
cv::waitKey( 0 );
// 筛选匹配,把距离太大的去掉
// 这里使用的准则是去掉大于四倍最小距离的匹配
vector< cv::DMatch > goodMatches;
//先将最小距离设置为一个很大的值
double minDis = 9999;
//然后遍历整个matches,找到最小的距离。
for ( size_t i=0; i<matches.size(); i++ )
{
if ( matches[i].distance < minDis )
minDis = matches[i].distance;
}
//遍历,小于最小距离四倍的匹配push进goodmatches。
for ( size_t i=0; i<matches.size(); i++ )
{
if (matches[i].distance < 4*minDis)
goodMatches.push_back( matches[i] );
}
// 显示 good matches
cout<<"good matches="<<goodMatches.size()<<endl;
cv::drawMatches( rgb1, kp1, rgb2, kp2, goodMatches, imgMatches );
cv::imshow( "good matches", imgMatches );
cv::imwrite( "../data/good_matches.png", imgMatches );
cv::waitKey(0);
// 计算图像间的运动关系
// 关键函数:cv::solvePnPRansac()
// 为调用此函数准备必要的参数
// 第一个帧的三维点
vector<cv::Point3f> pts_obj;
// 第二个帧的图像点
vector< cv::Point2f > pts_img;
// 相机内参
CAMERA_INTRINSIC_PARAMETERS C;
C.cx = 325.5;
C.cy = 253.5;
C.fx = 518.0;
C.fy = 519.0;
C.scale = 1000.0;
for (size_t i=0; i<goodMatches.size(); i++)
{
// query 是第一个关键点的索引, train 是第二个关键点的索引
//获取3d点
cv::Point2f p = kp1[goodMatches[i].queryIdx].pt;
// 获取d时要小心!x是向右的,y是向下的,所以y才是行,x是列!
ushort d = depth1.ptr<ushort>( int(p.y) )[ int(p.x) ];
if (d == 0)
continue;
// 将(u,v,d)转成(x,y,z)
cv::Point3f pt ( p.x, p.y, d );
cv::Point3f pd = point2dTo3d( pt, C );
pts_obj.push_back( pd );
//获取2d点
pts_img.push_back( cv::Point2f( kp2[goodMatches[i].trainIdx].pt ) );
}
double camera_matrix_data[3][3] = {
{C.fx, 0, C.cx},
{0, C.fy, C.cy},
{0, 0, 1}
};
// 构建相机矩阵
cv::Mat cameraMatrix( 3, 3, CV_64F, camera_matrix_data );
cv::Mat rvec, tvec, inliers;
// 求解pnp
cv::solvePnPRansac( pts_obj, pts_img, cameraMatrix, cv::Mat(), rvec, tvec, false, 100, 1.0, 0.99, inliers );
cout<<"inliers: "<<inliers.rows<<endl;
cout<<"R="<<rvec<<endl;
cout<<"t="<<tvec<<endl;
// 画出inliers匹配
vector< cv::DMatch > matchesShow;
for (size_t i=0; i<inliers.rows; i++)
{
matchesShow.push_back( goodMatches[inliers.ptr<int>(i)[0]] );
}
cv::drawMatches( rgb1, kp1, rgb2, kp2, matchesShow, imgMatches );
cv::imshow( "inlier matches", imgMatches );
cv::imwrite( "../data/inliers.png", imgMatches );
cv::waitKey( 0 );
return 0;
}