1.PCA思想:
PCA有两个作用,一个是降维,一个是数据可视化。通过降维可以达到数据可视化的目标。将D维降到K维:寻找K个向量,然后将样本点投影到这K个向量中,使得投影误差最小。比如:从2D到1D:找一条直线,将空间中的点垂直投影到这条直线距离上(正交距离),求一条使得这些距离之和最小的直线,从而实现降维。对于从3D到2D,找两个方向的直线,确定一个平面,然后将点投影到这个平面上,计算投影误差。很高维度的情况下,无法实现可视化,比如50维,我们可以利用PCA将其降到2维进行观察和可视化。
2.PCA步骤
step1:数据预处理 ;对于数据首先我们将其mean normalization(归一化)处理:
,然后用
更新样本值。其次,如果样本的特征值处于不同的范围,我们再进行特征缩放。
,
是均值,
一般是总和。
step2:计算协方差矩阵:
,其中n是维度,就是我们原来的维度是n维。因此协方差矩阵是n*n的。
step3:计算协方差矩阵的特征向量(eigenvectors):
[u,s,v]=svd(Sigma);这里的sigma就是协方差矩阵。svd是进行奇异值分解。svd分解将Sigma分解为U*S*V',,U为特征列向量。然后我们提取前K个特征向量,u_reduce=U(:,1:k);
step4:求解k维z:
z=u_reduce'*X;
如果想要从k维恢复到d维:则x_approx=u_reduce*z;
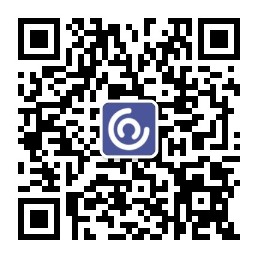
3.选择K?
,(分子表示平均误差,分母表示总变差),使该式最小,约小于1%、10%,则K为合适。
4.使用PCA:
1.首先对于监督学习来说,抽取一些无标签的样本经过PCA,变成
2.通过假设函数,比如逻辑回归的得到z对应的标签,得到一个新的训练集
...
3.然后在测试集和交叉验证集进行测试。将映射成
,然后通过h(z)得到了测试集
...
notes:不要随便使用PCA,在你有压缩数据等需求时使用它。PCA防止过拟合的效果并不好。
notes:PCA形式上与线性回归有点相似,实际上完全不同,PCA是正交距离,而线性回归是垂直距离。
5.编程作业:
function [U, S] = pca(X)
%PCA Run principal component analysis on the dataset X
% [U, S, X] = pca(X) computes eigenvectors of the covariance matrix of X
% Returns the eigenvectors U, the eigenvalues (on diagonal) in S
%
% Useful values
[m, n] = size(X);
% You need to return the following variables correctly.
U = zeros(n);
S = zeros(n);
% ====================== YOUR CODE HERE ======================
% Instructions: You should first compute the covariance matrix. Then, you
% should use the "svd" function to compute the eigenvectors
% and eigenvalues of the covariance matrix.
%
% Note: When computing the covariance matrix, remember to divide by m (the
% number of examples).
%
Sigma=zeros(n,n);
Sigma=(1/m)*X'*X;
[U,S,V]=svd(Sigma);
% =========================================================================
end
function Z = projectData(X, U, K)
%PROJECTDATA Computes the reduced data representation when projecting only
%on to the top k eigenvectors
% Z = projectData(X, U, K) computes the projection of
% the normalized inputs X into the reduced dimensional space spanned by
% the first K columns of U. It returns the projected examples in Z.
%
% You need to return the following variables correctly.
Z = zeros(size(X, 1), K);
% ====================== YOUR CODE HERE ======================
% Instructions: Compute the projection of the data using only the top K
% eigenvectors in U (first K columns).
% For the i-th example X(i,:), the projection on to the k-th
% eigenvector is given as follows:
% x = X(i, :)';
% projection_k = x' * U(:, k);
%
U_reduce=U(:,1:K);
Z=X*U_reduce;
% =============================================================
end
function X_rec = recoverData(Z, U, K)
%RECOVERDATA Recovers an approximation of the original data when using the
%projected data
% X_rec = RECOVERDATA(Z, U, K) recovers an approximation the
% original data that has been reduced to K dimensions. It returns the
% approximate reconstruction in X_rec.
%
% You need to return the following variables correctly.
X_rec = zeros(size(Z, 1), size(U, 1));
% ====================== YOUR CODE HERE ======================
% Instructions: Compute the approximation of the data by projecting back
% onto the original space using the top K eigenvectors in U.
%
% For the i-th example Z(i,:), the (approximate)
% recovered data for dimension j is given as follows:
% v = Z(i, :)';
% recovered_j = v' * U(j, 1:K)';
%
% Notice that U(j, 1:K) is a row vector.
%
U_reduce=U(:,1:K);
X_rec=Z*U_reduce';
% =============================================================
end