CompHub 实时聚合多平台的数据类(Kaggle、天池…)和OJ类(Leetcode、牛客…)比赛。本账号会推送最新的比赛消息,欢迎关注!
更多比赛信息见 CompHub主页
以下内容摘自比赛主页(点击文末阅读原文进入)
Part1赛题介绍
题目
Large-Scale Fine-Grained Building Classification for Semantic Urban Reconstruction
举办平台
背景
Buildings are essential components of urban areas. While research on the extraction and 3D reconstruction of buildings is widely conducted, information on the fine-grained roof types of buildings is usually ignored. This limits the potential of further analysis, e.g., in the context of urban planning applications. The fine-grained classification of building roof type from satellite images is a highly challenging task due to ambiguous visual features within the satellite imagery. The difficulty is further increased by the lack of corresponding fine-grained building classification datasets.
建筑是城市的重要组成部分。在建筑物的提取和三维重建研究广泛开展的同时,细粒度屋顶类型的信息往往被忽略。这限制了进一步分析的潜力,例如在城市规划应用的背景下。从卫星图像中对建筑物屋顶类型进行细粒度分类是一项极具挑战性的任务,因为卫星图像中存在模糊的视觉特征。由于缺乏相应的细粒度建筑分类数据集,难度进一步增加。
The 2023 IEEE GRSS Data Fusion Contest, organized by the Image Analysis and Data Fusion Technical Committee (IADF TC) of the IEEE Geoscience and Remote Sensing Society (GRSS), the Aerospace Information Research Institute under the Chinese Academy of Sciences, the Universität der Bundeswehr München, and GEOVIS Earth Technology Co., Ltd. aims to push current research on building extraction, classification, and 3D reconstruction towards urban reconstruction with fine-grained semantic information of roof types.
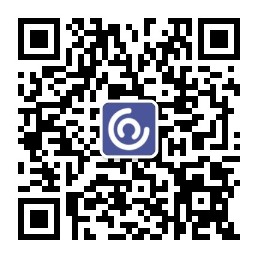
2023年IEEE GRSS数据融合大赛,由IEEE地球科学与遥感学会(GRSS)图像分析与数据融合技术委员会(IADF TC)、中国科学院航空航天信息研究所、Universität der Bundeswehr München和GEOVIS地球技术有限公司组织,旨在推动当前建筑提取、分类、以及基于屋顶类型语义信息的城市重建三维重建。
To this aim, the DFC23 establishes a large-scale, fine-grained, and multi-modal benchmark for the classification of building roof types. It consists of two challenging competition tracks investigating the fusion of optical and SAR data, while focusing on roof type classification and building height estimation, respectively.
为此,DFC23建立了大规模、细粒度、多模式的建筑屋顶类型分类基准。它由两个具有挑战性的赛道组成,研究光学和SAR数据的融合,同时分别专注于屋顶类型分类和建筑物高度估计。
Part2时间安排
-
Development Phase, Start: Jan. 4, 2023, noon
-
Test Phase, Start: March 6, 2023, noon
Part3奖励机制
-
The first, second, third and fourth-ranked teams in each track will be declared as winners.
-
The authors of the winning submissions will:
-
Present their approach in an invited session dedicated to the DFC23 at IGARSS 2023
-
Publish their manuscripts in the Proceedings of IGARSS 2023
-
Be awarded IEEE Certificates of Recognition
-
The first, second, and third-ranked teams of each track will receive 2,000, and $1,000 (USD), respectively, as a cash prize.
-
The authors of the first and second-ranked teams of each track will co-author a journal paper which will summarize the outcome of the DFC23 and will be submitted with open access to IEEE JSTARS.
-
Top-ranked teams will be awarded during IGARSS 2023, Pasadena, USA in July 2023.
Part4赛题描述
Track 1: Building Detection and Roof Type Classification
This track focuses on the detection and classification of building roof types from high-resolution satellite optical imagery and SAR images. The SAR and optical modalities are expected to provide complementary information. The given dataset covers senventeen cities worldwide across six continents. The classification task consists of 12 fine-grained, predefined roof types. Figure 1 shows an example.
本赛道的重点是从高分辨率卫星光学图像和SAR图像中检测和分类建筑物屋顶类型。SAR和光学模式有望提供补充信息。给定的数据集涵盖了全球六大洲的17个城市。分类任务由12种细粒度的预定义屋顶类型组成。图1显示了一个示例。
Figure 1: An example image tile of multi-modal data (optical and SAR) for building detection and roof type classification.
Track 2: Multi-Task Learning of Joint Building Extraction and Height Estimation
This track defines the joint task of building extraction and height estimation. Both are two very fundamental and essential tasks for building reconstruction. Same as in Track 1, the input data are multi-modal optical and SAR satellite imagery. Building extraction and height estimation from single-view satellite imagery depend on semantic features extracted from the imagery. Multi-task learning provides a potentially superior solution by reusing features and forming implicit constraints between multiple tasks in comparison to conventional separate implementations. Satellite images are provided with reference data, i.e., building annotations and normalized Digital Surface Models (nDSMs). The participants are required to reconstruct building heights and extract building footprints. Figure 2 shows an example.
本赛道定义了建筑物提取和高度估计的联合任务。这是建筑改造中两个非常基本和必要的任务。与赛道1相同,输入数据为多模态光学和SAR卫星图像。单视角卫星图像的建筑物提取和高度估计依赖于从图像中提取的语义特征。与传统的单独实现相比,多任务学习通过重用特性和在多个任务之间形成隐式约束,提供了一种潜在的优秀解决方案。卫星图像提供了参考数据,即建筑注释和标准化数字表面模型(ndsm)。参与者需要重建建筑高度和提取建筑足迹。图2显示了一个示例。
Figure 2: An example for joint building extraction and height estimation.