MapReduce中的参数传递
map阶段、reduce阶段的这些参数是如何传递的
Map阶段的输出
Reduce阶段的输出 (不建议第三种方式传参)
到此参数传递就OK了,然后导出jar包,传到home目录下面,然后运行看结果就可以了。只要有结果就可以了 ,说明参数过来了
<?xml version="1.0" encoding="UTF-8"?>
<?xml-stylesheet type="text/xsl" href="configuration.xsl"?>
<configuration>
<property>
<name>mapreduce.input.dir</name>
<value>/de</value>
</property>
<property>
<name>mapreduce.output.dir</name>
<value>/out/00</value>
</property>
</configuration>
package qf.com.mr;
import java.io.IOException;
import org.apache.hadoop.conf.Configuration;
import org.apache.hadoop.fs.FileSystem;
import org.apache.hadoop.fs.Path;
import org.apache.hadoop.io.IntWritable;
import org.apache.hadoop.io.LongWritable;
import org.apache.hadoop.io.Text;
import org.apache.hadoop.mapreduce.Job;
import org.apache.hadoop.mapreduce.Mapper;
import org.apache.hadoop.mapreduce.Reducer;
import org.apache.hadoop.mapreduce.lib.input.FileInputFormat;
import org.apache.hadoop.mapreduce.lib.output.FileOutputFormat;
import org.apache.hadoop.util.Tool;
import org.apache.hadoop.util.ToolRunner;
/*
*类说明:参数传递
....
*/
public class ParamDemo implements Tool{
public static final int three = 666;
/**
* map阶段
* @author HP
*
*/
public static class MyMapper extends Mapper<LongWritable, Text, Text, IntWritable>{
public static Text k = new Text();
public static IntWritable v = new IntWritable();
@Override
protected void map(LongWritable key, Text value, Context context)
throws IOException, InterruptedException {
//1.从输入数据中获取每一个文件中的每一行的值
String line = value.toString();
//2.对每一行的数据进行切分(有的不用)
String [] words = line.split(" ");
//3.循环处理
for (String word : words) {
k.set(word);
v.set(1);
//map阶段的输出 context上下文环境变量
context.write(k, v);//这个输出在循环里面 有一个输出一个
}
context.getCounter("first param in map", context.getConfiguration().get("param1"));
context.getCounter("second param in map", context.getConfiguration().get("param2"));
context.getCounter("three param in map", three + "");
}
}
/**
* reduce阶段
*/
public static class MyReducer extends Reducer<Text, IntWritable, Text, IntWritable>{
@Override
protected void reduce(Text key, Iterable<IntWritable> values, Context context)
throws IOException, InterruptedException {
//1.自定义一个计数器
int counter = 0;
for (IntWritable i : values) {
counter += i.get();
}
//2.reduce阶段的最终输出
context.write(key, new IntWritable(counter));
//这个输出在循环外面 等统计完了这一个容器再输出
context.getCounter("first param in reduce", context.getConfiguration().get("param1"));
context.getCounter("second param in reduce", context.getConfiguration().get("param2"));
context.getCounter("three param in reduce", three + "");
}
}
public void setConf(Configuration conf) {
// 对conf的属性设置
conf.set("fs.defaultFS", "hdfs://qf");
conf.set("dfs.nameservices", "qf");
conf.set("dfs.ha.namenodes.qf", "nn1, nn2");
conf.set("dfs.namenode.rpc-address.qf.nn1", "hadoop01:9000");
conf.set("dfs.namenode.rpc-address.qf.nn2", "hadoop02:9000");
conf.set("dfs.client.failover.proxy.provider.qf", "org.apache.hadoop.hdfs.server.namenode.ha.ConfiguredFailoverProxyProvider");
}
public Configuration getConf() {
return new Configuration();
}
public int run(String[] args) throws Exception {
// 1.获取配置对象信息
Configuration conf = new Configuration();
//读取配置文件
conf.addResource(ParamDemo.class.getResourceAsStream("/Param.xml"));
conf.set("param1", "first param");
conf.set("param2", args[0]);
// 3.获取job对象 (注意导入的包)
Job job = Job.getInstance(conf, "job");
// 4.设置job的运行主类
job.setJarByClass(ParamDemo.class);
//set inputpath and outputpath
setInputAndOutput(job, conf, args);
// System.out.println("jiazai finished");
// 5.对map阶段进行设置
job.setMapperClass(MyMapper.class);
job.setMapOutputKeyClass(Text.class);
job.setMapOutputValueClass(IntWritable.class);
// System.out.println("map finished");
// 6.对reduce阶段进行设置
job.setReducerClass(MyReducer.class);
job.setOutputKeyClass(Text.class);
job.setOutputValueClass(IntWritable.class);
return job.waitForCompletion(true) ? 0 : 1;
}
//主方法
public static void main(String[] args) throws Exception {
int isok = ToolRunner.run(new Configuration(), new ParamDemo(), args);
System.out.println(isok);
}
/**
* 处理参数的方法
* @param job
* @param conf
* @param args
*/
private void setInputAndOutput(Job job, Configuration conf, String[] args) {
if(args.length != 1) {
System.out.println("usage:yarn jar /*.jar package.classname /* /*");
return ;
}
//正常处理输入输出参数
try {
FileInputFormat.addInputPath(job, new Path(conf.get("mapreduce.input.dir")));
FileSystem fs = FileSystem.get(conf);
Path outputpath = new Path(conf.get("mapreduce.output.dir"));
if(fs.exists(outputpath)) {
fs.delete(outputpath, true);
}
FileOutputFormat.setOutputPath(job, outputpath);
} catch (Exception e) {
e.printStackTrace();
}
}
}
MapReduce的压缩案例
扫描二维码关注公众号,回复:
9247151 查看本文章
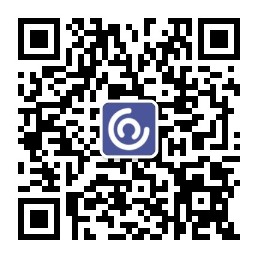
map是压缩文件,reduce阶段自动会去找解压算法
然后直接打包,
对应其他的压缩算法怎么看呢